ARMOR: Differential Model Distribution for Adversarially Robust Federated Learning
ELECTRONICS(2023)
摘要
In this work, we formalize the concept of differential model robustness (DMR), a new property for ensuring model security in federated learning (FL) systems. For most conventional FL frameworks, all clients receive the same global model. If there exists a Byzantine client who maliciously generates adversarial samples against the global model, the attack will be immediately transferred to all other benign clients. To address the attack transferability concern and improve the DMR of FL systems, we propose the notion of differential model distribution (DMD) where the server distributes different models to different clients. As a concrete instantiation of DMD, we propose the ARMOR framework utilizing differential adversarial training to prevent a corrupted client from launching white-box adversarial attack against other clients, for the local model received by the corrupted client is different from that of benign clients. Through extensive experiments, we demonstrate that ARMOR can significantly reduce both the attack success rate (ASR) and average adversarial transfer rate (AATR) across different FL settings. For instance, for a 35-client FL system, the ASR and AATR can be reduced by as much as 85% and 80% over the MNIST dataset.
更多查看译文
关键词
federated learning,model robustness,adversarial training,differential model distribution,Byzantine robustness
AI 理解论文
溯源树
样例
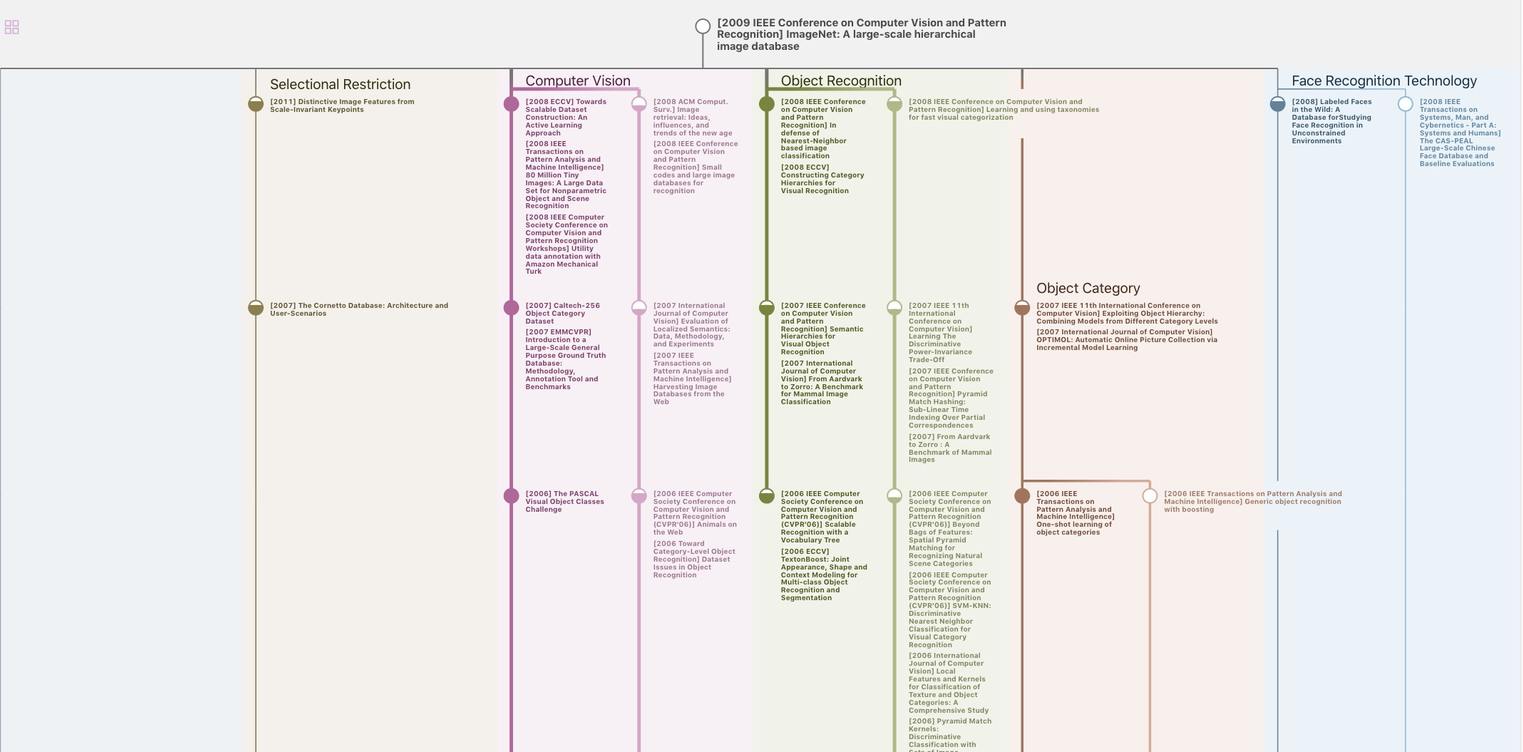
生成溯源树,研究论文发展脉络
Chat Paper
正在生成论文摘要