Reinforcement Learning for Autonomous Underwater Vehicles via Data-Informed Domain Randomization
APPLIED SCIENCES-BASEL(2023)
摘要
Featured Application The proposed RL via data-informed Domain Randomization (DDR) is designed to stabilize autonomous underwater vehicles and the platforms of underwater vehicle-manipulator systems, which are further subject to unknown dynamics and varying payloads. The approach captures the differences in dynamics between the simulated AUVs and real AUVs, trains the controller in simulations that are suitable for real AUVs, and avoids the tedious procedures of parameter-tuning. The proposed RL-DDR requires only a few training samples, making the controller adaptation efficient for real-time applications. Autonomous Underwater Vehicles (AUVs) or underwater vehicle-manipulator systems often have large model uncertainties from degenerated or damaged thrusters, varying payloads, disturbances from currents, etc. Other constraints, such as input dead zones and saturations, make the feedback controllers difficult to tune online. Model-free Reinforcement Learning (RL) has been applied to control AUVs, but most results were validated through numerical simulations. The trained controllers often perform unsatisfactorily on real AUVs; this is because the distributions of the AUV dynamics in numerical simulations and those of real AUVs are mismatched. This paper presents a model-free RL via Data-informed Domain Randomization (DDR) for controlling AUVs, where the mismatches between the trajectory data from numerical simulations and the real AUV were minimized by adjusting the parameters in the simulated AUVs. The DDR strategy extends the existing adaptive domain randomization technique by aggregating an input network to learn mappings between control signals across domains, enabling the controller to adapt to sudden changes in dynamics. The proposed RL via DDR was tested on the problems of AUV pose regulation through extensive numerical simulations and experiments in a lab tank with an underwater positioning system. These results have demonstrated the effectiveness of RL-DDR for transferring trained controllers to AUVs with different dynamics.
更多查看译文
关键词
autonomous underwater vehicles,uncertainty attenuation,reinforcement learning,domain randomization
AI 理解论文
溯源树
样例
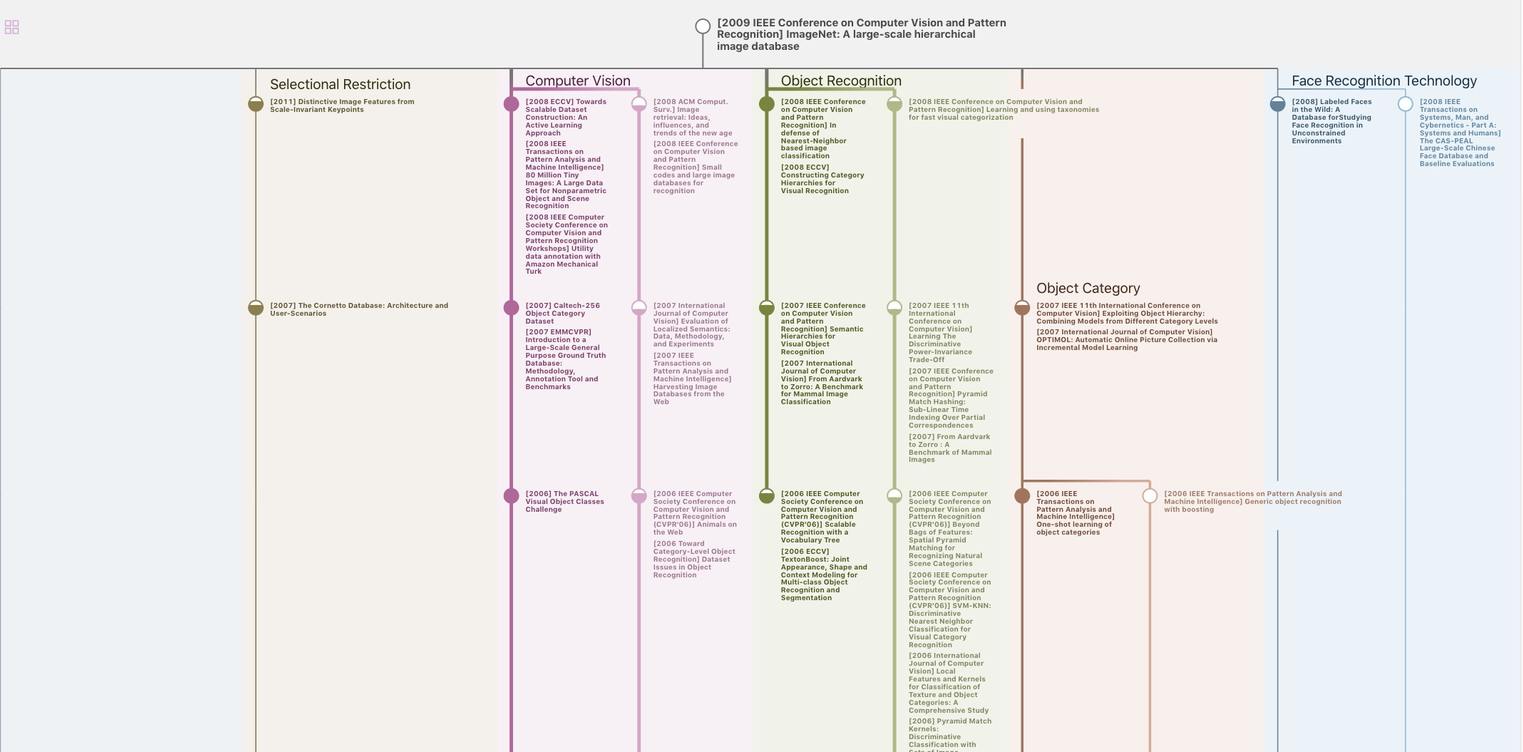
生成溯源树,研究论文发展脉络
Chat Paper
正在生成论文摘要