Machine Learning Techniques for Non-Terrestrial Networks
ELECTRONICS(2023)
摘要
Traditionally, non-terrestrial networks (NTNs) are used for a limited set of applications, such as TV broadcasting and communication support during disaster relief. Nevertheless, due to their technological improvements and integration in the 5G 3GPP standards, NTNs have been gaining importance in the last years and will provide further applications and services. 3GPP standardization is integrating low-Earth orbit (LEO) satellites, high-altitude platform stations (HAPSs) and unmanned aerial systems (UASs) as non-terrestrial elements (NTEs) in the NTNs within the terrestrial 5G standard. Considering the NTE characteristics (e.g., traffic congestion, processing capacity, oscillation, altitude, pitch), it is difficult to dynamically set the optimal connection based also on the required service to properly steer the antenna beam or to schedule the UE. To this aim, machine learning (ML) can be helpful. In this paper, we present novel services supported by the NTNs and their architectures for the integration in the terrestrial 5G 3GPP standards. Then, ML techniques are proposed for managing NTN connectivity as well as to improve service performance.
更多查看译文
关键词
non-terrestrial networks,machine learning,unmanned aerial vehicle,high-altitude platform station,low-Earth orbit satellite,3GPP release 17,3GPP release 18
AI 理解论文
溯源树
样例
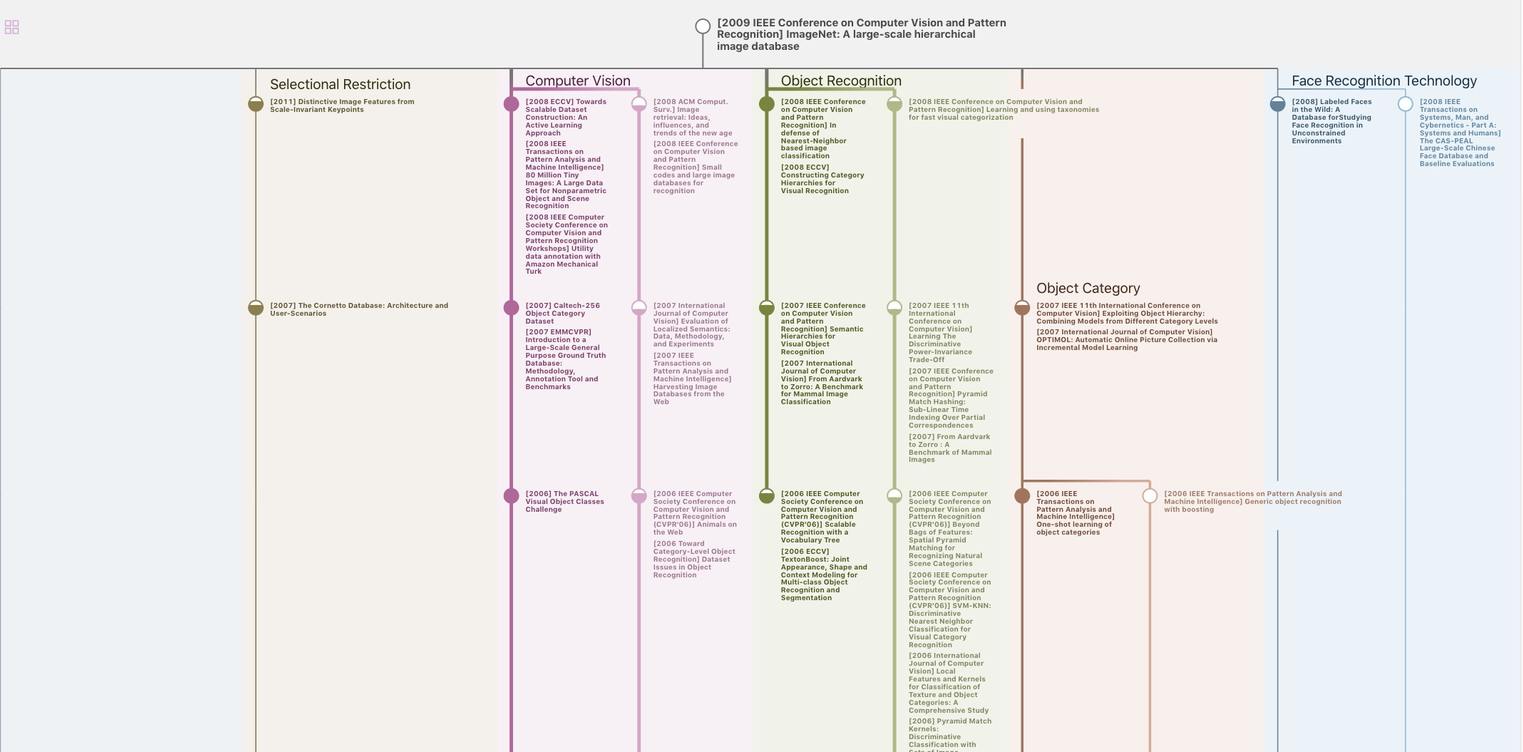
生成溯源树,研究论文发展脉络
Chat Paper
正在生成论文摘要