Multi-level self-adaptive prototypical networks for few-shot node classification on attributed networks
NEURAL COMPUTING & APPLICATIONS(2023)
摘要
Attributed networks, such as social networks, citation networks, and traffic networks, are ubiquitous nowadays. Node classification is an essential analysis task on attributed networks that has attracted increasing research attention. However, the conventional node classification methods usually require a large number of labeled training instances, which is labor-consuming and cannot be applied to real-world attributed networks. Thus, various meta-learning methods have been proposed to tackle the problem of insufficient labeled instances. However, few-shot learning for graph-structured data is still under-explored due to two reasons: (1) In the few-shot scenario, equally treating each node makes the model vulnerable to noisy instances; (2) The adjacency matrix and attributed features suffer from the data sparsity and not all dimensions in the feature space are discriminative to support final classification. To solve the above problems, we propose a novel graph meta-learning framework: Multi-Level Self-Adaptive Prototypical Networks (MSPN). The proposed MSPN leverages multi-level adaptive learning to highlight the most expressive nodes and features and rectify the prototype to obtain a more accurate one. The episodic training strategy mimics the real testing scenario and transfers knowledge from previous tasks to unseen target tasks. Extensive experimental results demonstrate that the MSPN is superior to the other methods on few-shot node classification.
更多查看译文
关键词
Attributed networks,Few-shot learning,Node classification,Graph neural network,Attentive strategy
AI 理解论文
溯源树
样例
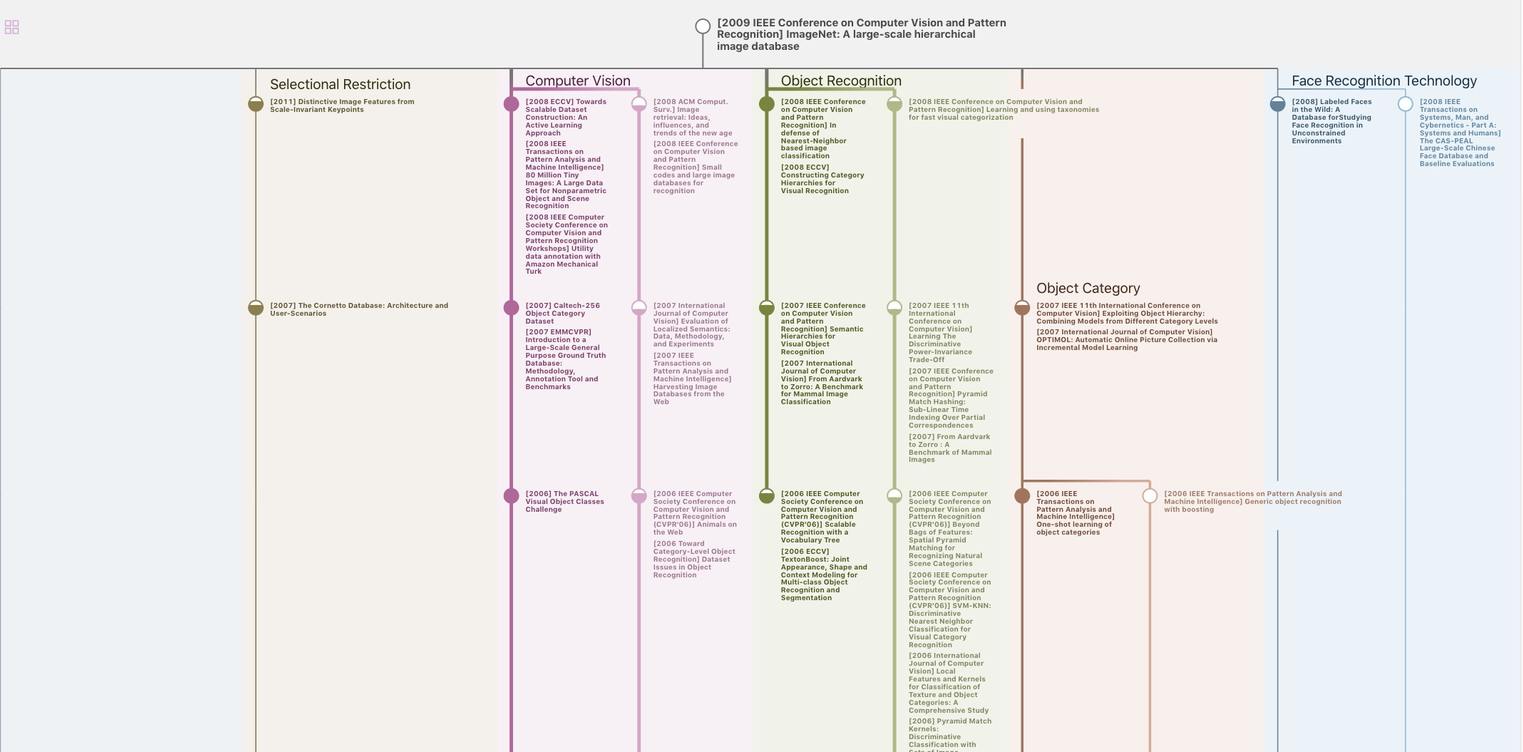
生成溯源树,研究论文发展脉络
Chat Paper
正在生成论文摘要