mfeeU-Net: A multi-scale feature extraction and enhancement U-Net for automatic liver segmentation from CT Images.
MATHEMATICAL BIOSCIENCES AND ENGINEERING(2023)
摘要
Medical image segmentation of the liver is an important prerequisite for clinical diagnosis and evaluation of liver cancer. For automatic liver segmentation from Computed Tomography (CT) images, we proposed a Multi-scale Feature Extraction and Enhancement U-Net (mfeeU-Net), incorporating Res2Net blocks, Squeeze-and-Excitation (SE) blocks, and Edge Attention (EA) blocks. The Res2Net blocks which are conducive to extracting multi-scale features of the liver were used as the backbone of the encoder, while the SE blocks were also added to the encoder to enhance channel information. The EA blocks were introduced to skip connections between the encoder and the decoder, to facilitate the detection of blurred liver edges where the intensities of nearby organs are close to the liver. The proposed mfeeU-Net was trained and evaluated using a publicly available CT dataset of LiTS2017. The average dice similarity coefficient, intersection-over-union ratio, and sensitivity of the mfeeU-Net for liver segmentation were 95.32%, 91.67%, and 95.53%, respectively, and all these metrics were better than those of U-Net, Res-U-Net, and Attention U-Net. The experimental results demonstrate that the mfeeU-Net can compete with and even outperform recently proposed convolutional neural networks and effectively overcome challenges, such as discontinuous liver regions and fuzzy liver boundaries.
更多查看译文
关键词
liver segmentation,U-Net,multi-scale,feature extraction and enhancement,Res2Net,squeeze-and-excitation,edge attention
AI 理解论文
溯源树
样例
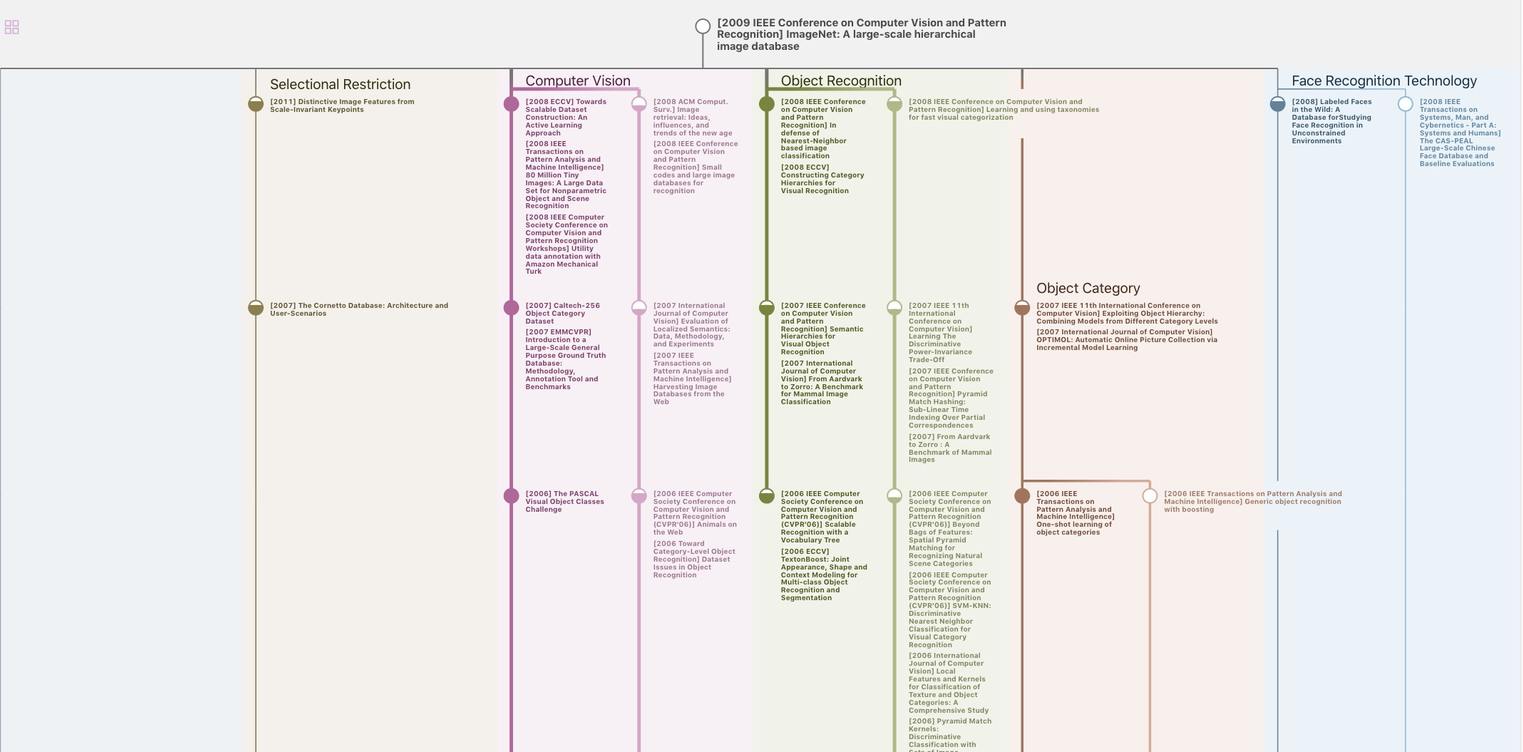
生成溯源树,研究论文发展脉络
Chat Paper
正在生成论文摘要