Prediction of tunnel mechanical behaviour using multi-task deep learning under the external condition
GEORISK-ASSESSMENT AND MANAGEMENT OF RISK FOR ENGINEERED SYSTEMS AND GEOHAZARDS(2024)
摘要
Accurate prediction of the future mechanical behaviour of the underground structure is important for the traffic system. However, the existing works mostly just predicted one type of property and failed to study the influence of different properties in the tunnel. Besides, most of them predicted future behaviours without considering the external influence of the environment like temperature and water pressure. In this paper, we propose a multi-task prediction model named MSTNet which combines different types of indicators and external factors for capturing the temporal and spatial characteristics in the tunnel. Firstly, we integrate time series of multiple indicators and build a deep learning algorithm based on a graph neural network and recurrent network to capture the temporal, spatial and external impacts in the tunnel. Then, we have a case study on the Wuhan Yangtze River tunnel which contains the comparison of different components in our model, the compared results between the single indicator and multiple indicators and the performance analysis among traditional models. From the experiment results, we could find that the ability of the MSTNet model is superior to other methods, whose capability achieved over 93% and 94% in strain variation and joint opening sensors on Pearson Correlation Coefficient (PCC) in the next 45 days, respectively.
更多查看译文
关键词
Tunnel,deep learning,mechanical behaviours,prediction,data mining
AI 理解论文
溯源树
样例
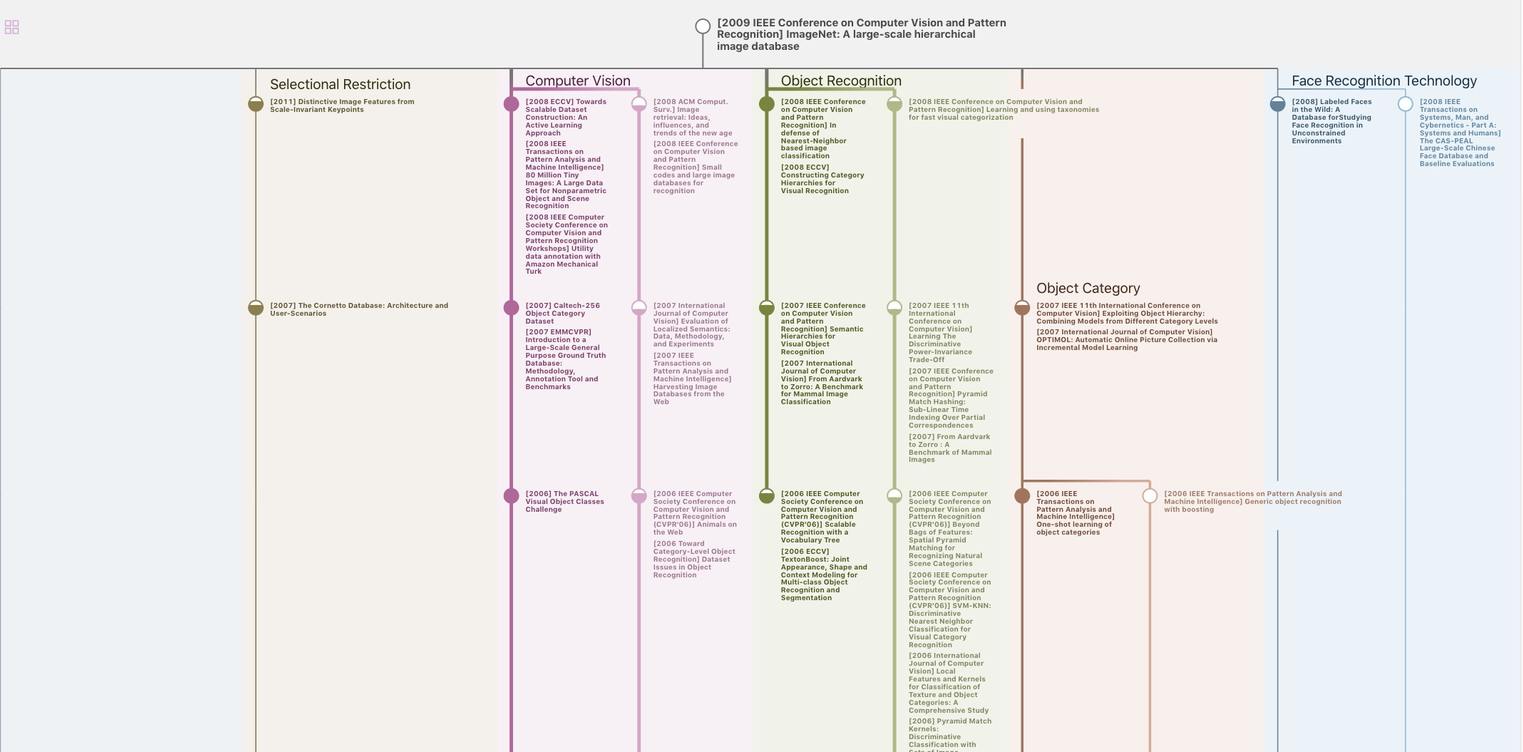
生成溯源树,研究论文发展脉络
Chat Paper
正在生成论文摘要