An optimized hybrid deep learning model for PM 2.5 and O 3 concentration prediction
AIR QUALITY ATMOSPHERE AND HEALTH(2023)
摘要
As people focus more on environmental protection, air quality prediction plays an increasingly important role in reducing pollution hazards. Both fine particulate matter (PM 2.5 ) and ozone (O 3 ) pollutants can cause serious damage to human health and property, so it is necessary to accurately predict the concentration of these pollutants. In this study, a hybrid deep air quality prediction model consisting of a one-dimensional convolutional neural network (CNN), bidirectional long-term and short-term memory (BiLSTM), and a gated recurrent unit (GRU) is proposed to predict air quality pollutant concentrations. This model overcomes the limitations of a single model while taking advantages of its benefits. The BiLSTM neural network has more parameters and poor convergence performance, and the GRU has a poor ability to capture long-distance dependencies between features. Compared with the other three deep learning models, the CNN-BiLSTM-GRU model achieves better prediction results. The model proposed in this paper with both meteorological factors and pollutant factors shows the best prediction results with an R 2 of 0.956 and RMSE of 17.2 μg/m 3 for PM 2.5 and an R 2 of 0.958 and RMSE of 13.43 μg/m 3 for O 3 . The original data set from the Aotizhongxin Observator of Beijing with 35,064 samples is selected as the experimental data. The experimental results show that the CNN-BiLSTM-GRU model proposed in this paper achieves the best prediction results. The results show that the proposed model can predict PM 2.5 and O 3 more accurately and more robustly, which indicates that it is a promising method for air and particulate pollutants’ performance prediction.
更多查看译文
关键词
Air pollution forecasting,Convolutional neural network,Long-term and short-term memory,Gated recurrent unit
AI 理解论文
溯源树
样例
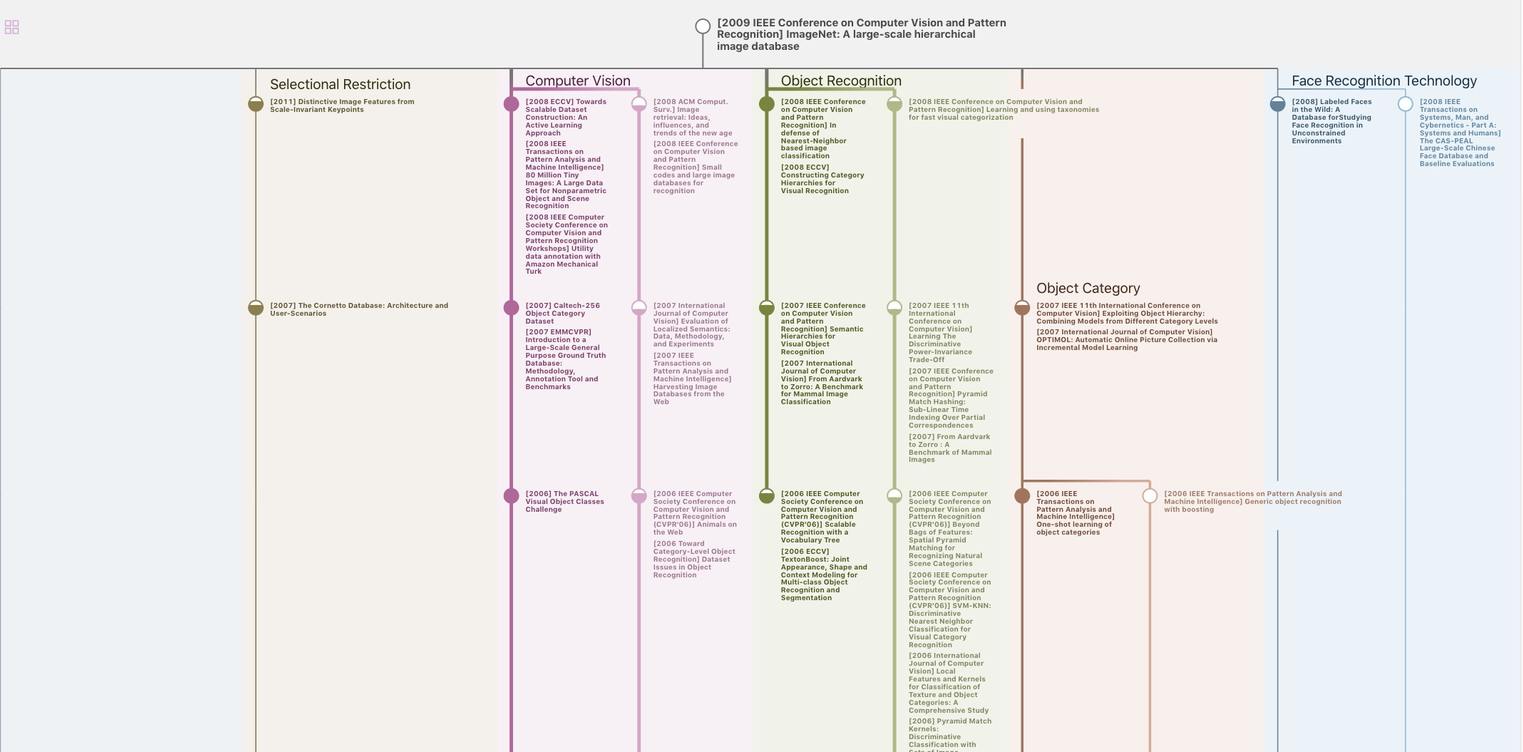
生成溯源树,研究论文发展脉络
Chat Paper
正在生成论文摘要