Country-wide retrieval of forest structure from optical and SAR satellite with ensembles
ISPRS JOURNAL OF PHOTOGRAMMETRY AND REMOTE SENSING(2023)
摘要
Monitoring and managing Earth's forests in an informed manner is an important requirement for addressing challenges like biodiversity loss and climate change. While traditional in situ or aerial campaigns for forest assessments provide accurate data for analysis at regional level, scaling them to entire countries and beyond with high temporal resolution is hardly possible. In this work, we propose a method based on deep ensembles that densely estimates forest structure variables at country-scale with 10-m resolution, using freely available satellite imagery as input. Our method jointly transforms Sentinel-2 optical images and Sentinel-1 synthetic -aperture radar images into maps of five different forest structure variables: 95th height percentile, mean height, density, Gini coefficient, and fractional cover. We train and test our model on reference data from 41 airborne laser scanning missions across Norway and demonstrate that it is able to generalize to unseen test regions, achieving normalized mean absolute errors between 11% and 15%, depending on the variable. Our work is also the first to propose a variant of so-called Bayesian deep learning to densely predict multiple forest structure variables with well-calibrated uncertainty estimates from satellite imagery. The uncertainty information increases the trustworthiness of the model and its suitability for downstream tasks that require reliable confidence estimates as a basis for decision making. We present an extensive set of experiments to validate the accuracy of the predicted maps as well as the quality of the predicted uncertainties. To demonstrate scalability, we provide Norway-wide maps for the five forest structure variables.
更多查看译文
关键词
Bayesian deep learning,Forest structure,Multispectral,SAR,Country-scale,Sentinel
AI 理解论文
溯源树
样例
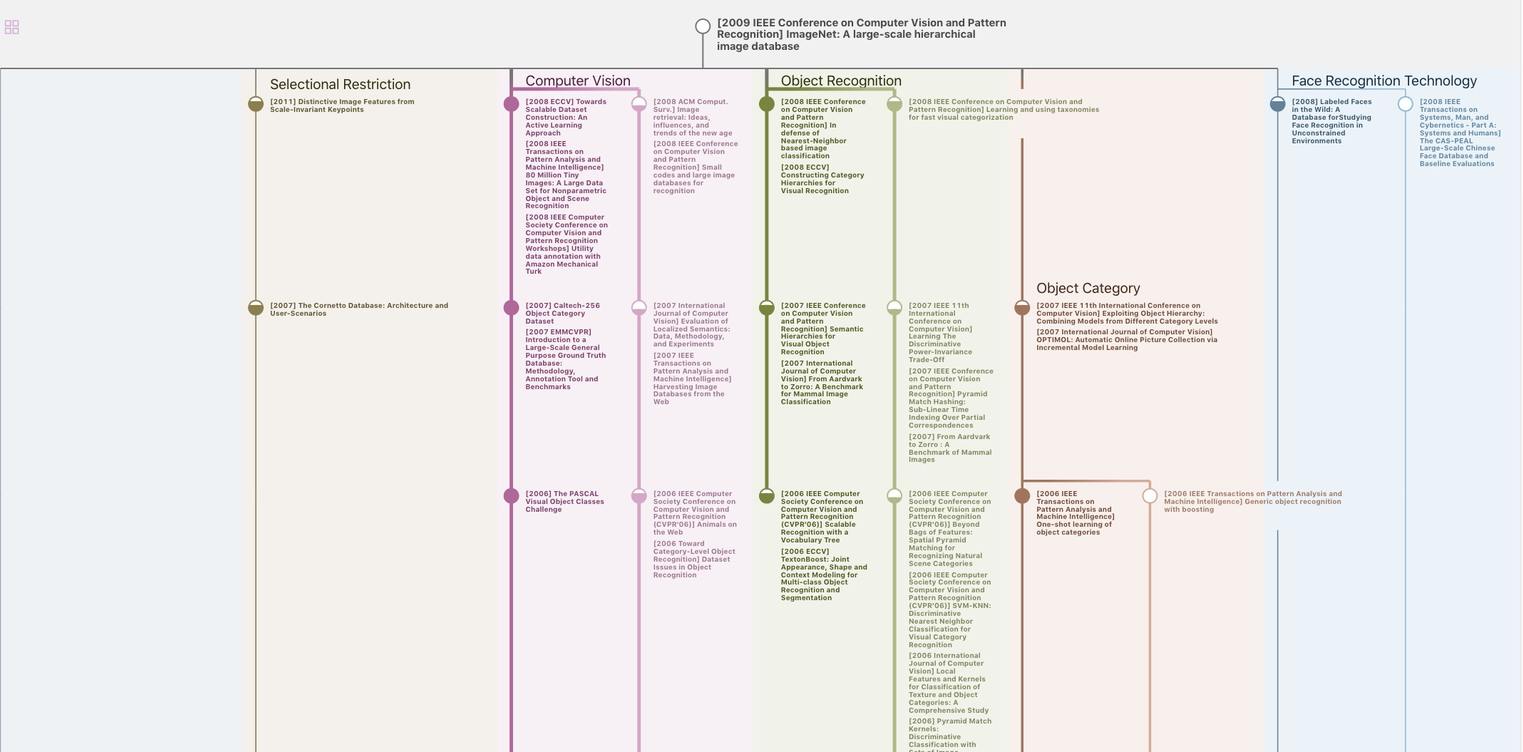
生成溯源树,研究论文发展脉络
Chat Paper
正在生成论文摘要