PAG-TSN: Ridership Demand Forecasting Model for Shared Travel Services of Smart Transportation
IEEE TRANSACTIONS ON INTELLIGENT TRANSPORTATION SYSTEMS(2023)
摘要
With the increasing popularity of cab services such as Didi and Uber, cities are faced with the challenge of high carbon emissions and traffic congestion. Ride-sharing services, as a novel green mode of transportation, have emerged as a key technology in smart transportation for addressing these problems. The implementation of ride-sharing is predicated on an accurate ridership demand forecasting model, which can effectively prevent vehicle resource waste, alleviate traffic congestion, and reduce carbon emissions. In this paper, a periodic attentional graph convolutional spatio-temporal network model (PAG-TSN) is proposed to predict regional ridership demand. Specifically, the model is trained using a large amount of GPS data and user demand data collected by the travel service provider. PAG-TSN consists of two parts: the bicomponent attention graph convolution model (BAT-GCN) and the periodic attentional gated recurrent unit model (PA-GRU). The former uses GCN to extract spatial features from pointwise and edgewise graphs; the latter uses the spatial feature vectors extracted from the former with external information as input, and uses GRU to extract temporal features from feature data of different periods, and finally uses attention mechanism and POI requirement correlation to integrate the extracted spatio-temporal information to derive prediction results. Extensive experiments and evaluations on the CD2Date and XA2Date datasets show that PAG-TSN outperforms other baseline models in accurately predicting regional ridership demand, with MAPE and RMSE values of 0.1147 and 5.56, respectively.
更多查看译文
关键词
Predictive models,Feature extraction,Correlation,Urban areas,Convolutional neural networks,Data models,Roads,Urban computing,spatio-temporal correlation,graph convolutional networks,ridership demand forecasting,smart transportation
AI 理解论文
溯源树
样例
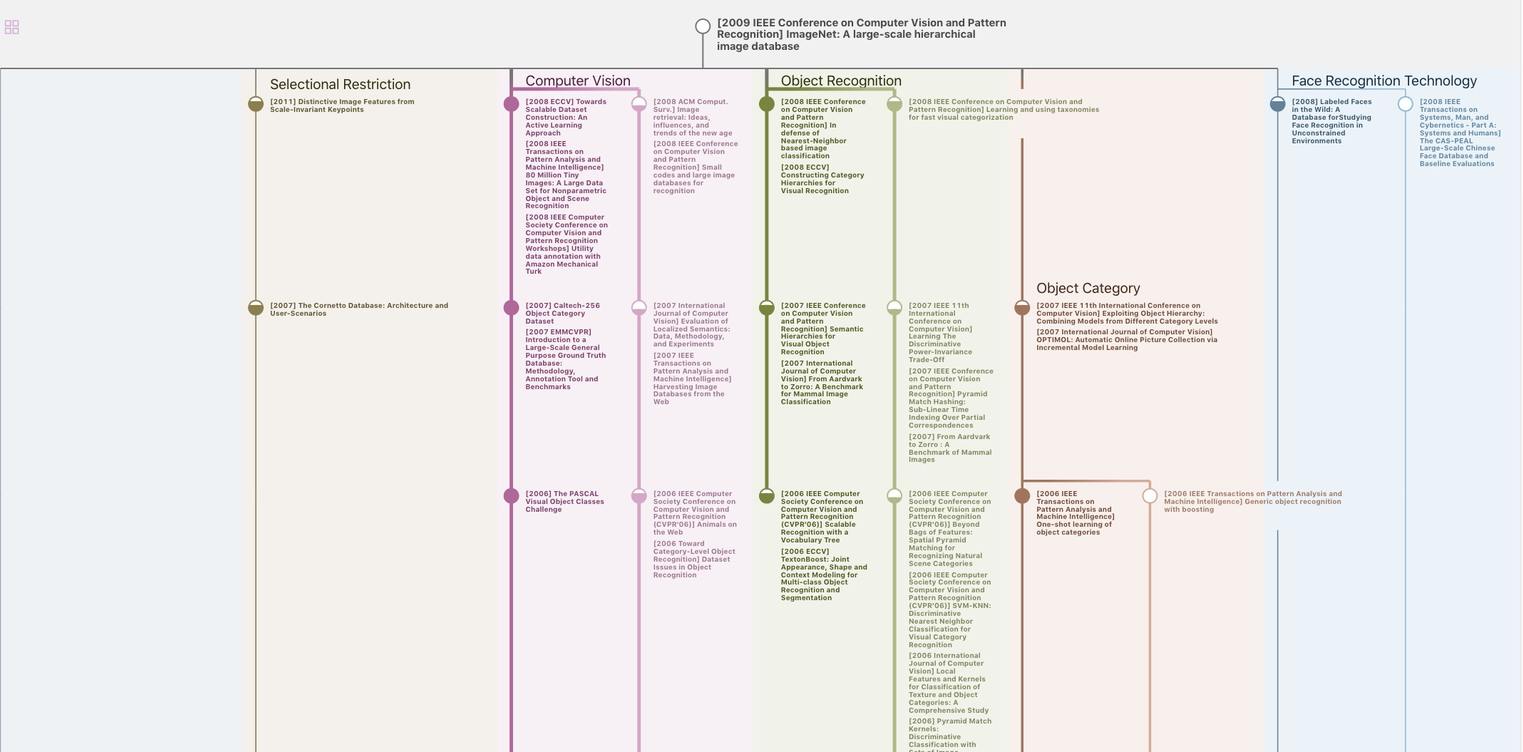
生成溯源树,研究论文发展脉络
Chat Paper
正在生成论文摘要