GLAN: GAN Assisted Lightweight Attention Network for Biomedical Imaging Based Diagnostics
COGNITIVE COMPUTATION(2023)
摘要
Manual assessment of biomedical imaging based diagnostics is limited as it is time-consuming and subjective. Bio-inspired diagnostics applications on embedded and mobile devices are becoming more popular as they overcome these limitations and aid in early detection and diagnosis. The neural theory of visual attention puts forward that the processing resources are dedicated to more important information, for resource saving and performance improvement. Moreover, adversarial learning can potentially alleviate the various biases of the human cognitive system. The limited performance of the current lightweight network approaches can be attributed to the absence of above-mentioned properties of the human cognition. Accordingly, we introduce GLAN, a lightweight attention based network that is particularly well suited to embedded and mobile devices. GLAN’s design follows lightweight architecture design principles for encoder-decoder design. To improve performance a twofold novel strategy is adopted. Firstly, we equip the encoder and the decoder with lightweight attention mechanisms to increase their focus and improve segmentation performance. Secondly, adversarial learning is employed with augmentation to increase the generalization ability of the lightweight attention network. e have evaluated our GLAN on three different applications including lung segmentation, digestive tract polyp segmentation and optic disc segmentation. GLAN is quite competitive in terms of segmentation performance while comprehensively outperforming recent alternatives in terms of computational requirements. Specifically, GLAN requires 94.17%, 78.18%, 80.95% and 67.56% less computational parameters as compared with four recent lightweight alternatives. This advocates its application in real-time biomedical imaging diagnostics on embedded and mobile devices in clinical settings.
更多查看译文
关键词
Biomedical imaging,Diagnostics,Real-time applications,Medical image segmentation,Computational requirements
AI 理解论文
溯源树
样例
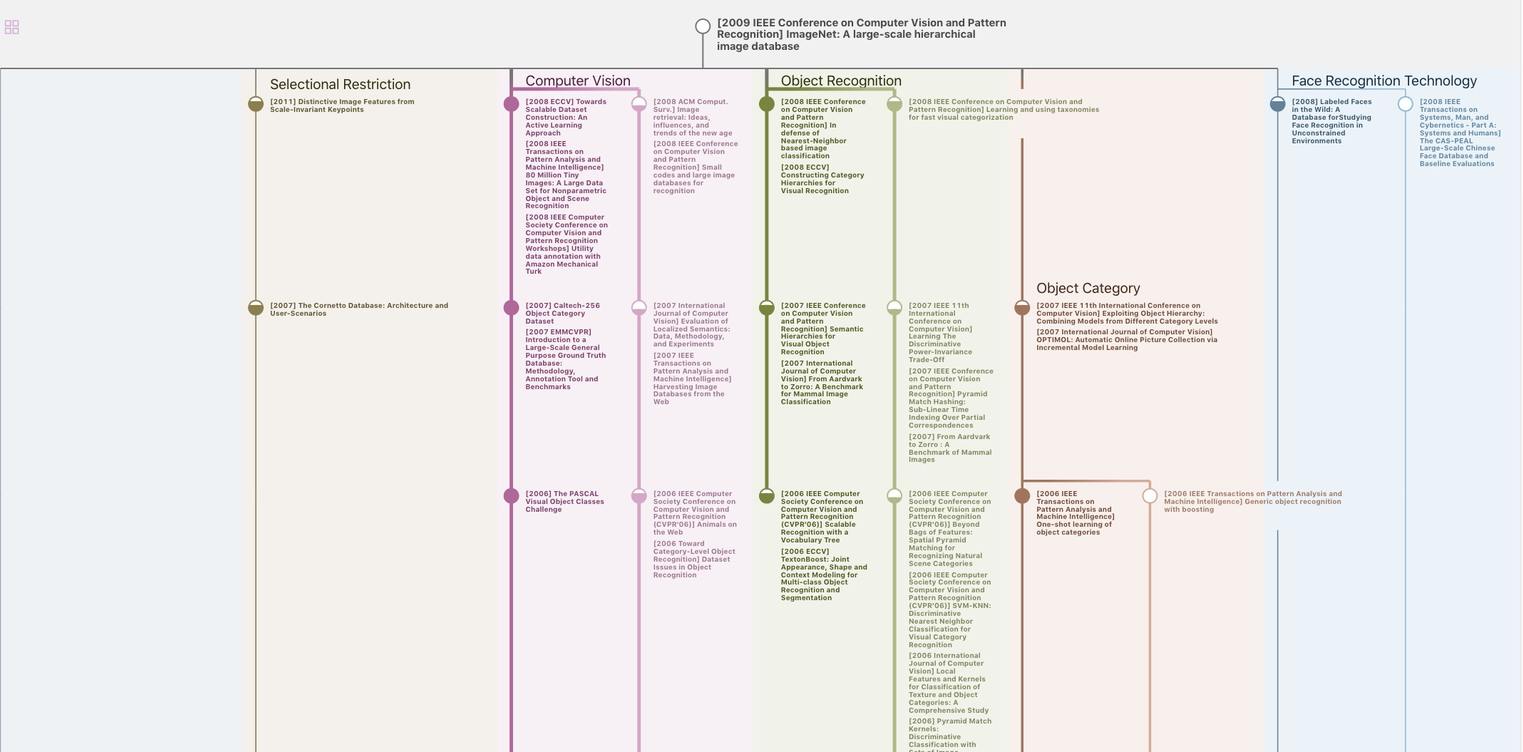
生成溯源树,研究论文发展脉络
Chat Paper
正在生成论文摘要