Surrogate Evaluation Model for Assessing Lane Detection Reliability of Automated Vehicles in Complex Road Environments
TRANSPORTATION RESEARCH RECORD(2023)
摘要
The reliability of environmental perception is the key to ensuring the stability and safety of autonomous vehicles. Complex road environments pose attentional challenges to automated driving, especially for vehicle perception systems. Therefore, evaluating the performance of autonomous driving algorithms in complex road environments is of great significance. However, as road geometry is complex, with many factors, including curvature, slope, lane marking conditions, and so on, the existing approach in assessing an algorithm through detection tests is of low efficiency. Therefore, this study relies on failure prediction modeled by extreme gradient boosting and recursive feature elimination. An evaluation model that could accomplish a quick set of road evaluations is developed. The proposed model generates recognition reliability merely by such features as road design parameters. In addition, road curvature is the most significant environmental factor affecting the perception of autonomous vehicles. The failure rates of the algorithm in different scenarios demonstrate a negative correlation between the radius of curvature and failure perception. The partial dependence involving the radius of curvature and the perception ability is obtained. Furthermore, other features, such as missing lane lines and illumination conditions, are taken into consideration to explore the potential failure perception mode. The proposed method has a particular significance for finding the failure mode of an autonomous driving perception algorithm.
更多查看译文
关键词
road design,autonomous driving,horizontal alignment,ensemble learning,surrogate evaluation model
AI 理解论文
溯源树
样例
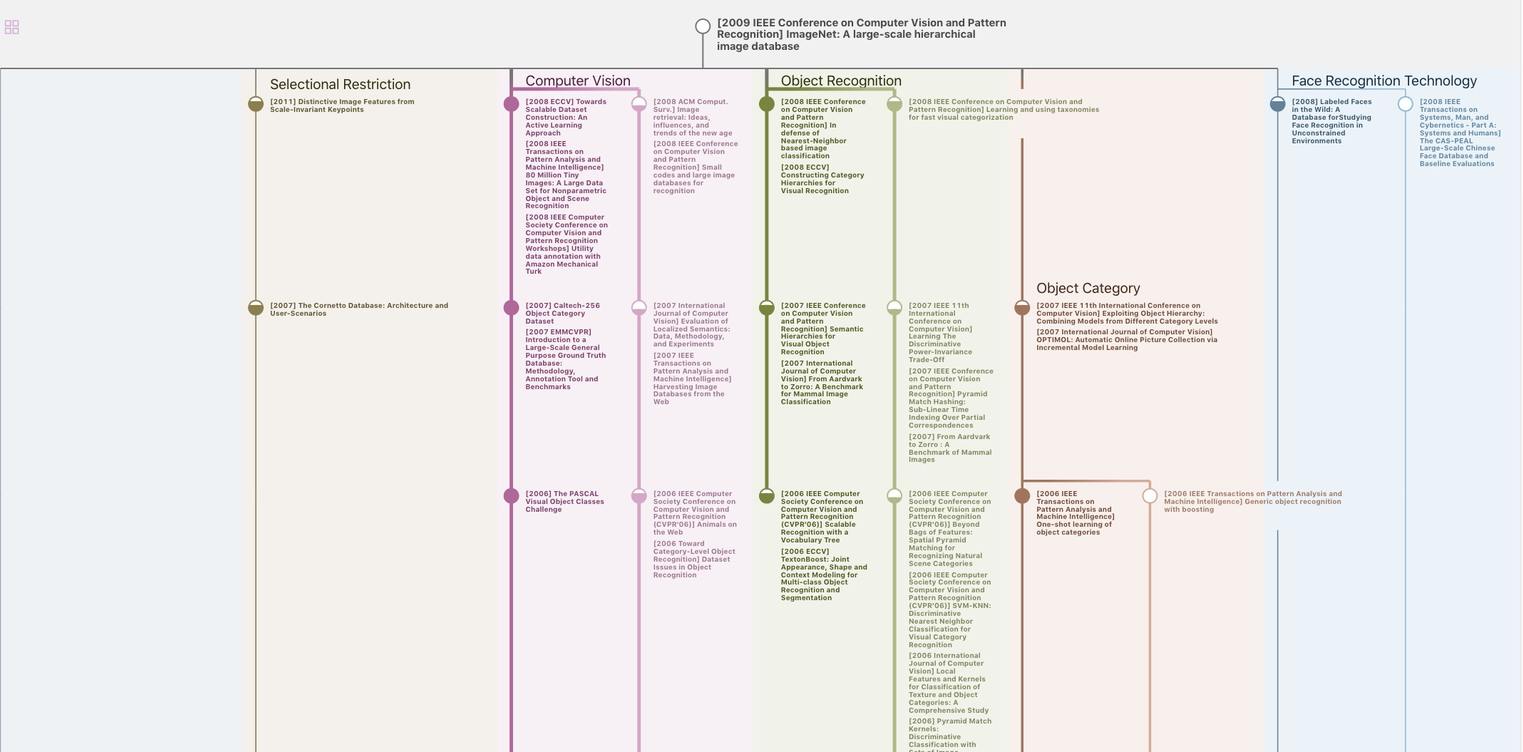
生成溯源树,研究论文发展脉络
Chat Paper
正在生成论文摘要