EEG-Based Sleep Stage Classification via Neural Architecture Search
IEEE TRANSACTIONS ON NEURAL SYSTEMS AND REHABILITATION ENGINEERING(2023)
摘要
With the improvement of quality of life, people are more and more concerned about the quality of sleep. The electroencephalogram (EEG)-based sleep stage classification is a good guide for sleep quality and sleep disorders. At this stage, most automatic staging neural networks are designed by human experts, and this process is time-consuming and laborious. In this paper, we propose a novel neural architecture search (NAS) framework based on bilevel optimization approximation for EEG-based sleep stage classification. The proposed NAS architecture mainly performs the architectural search through a bilevel optimization approximation, and the model is optimized by search space approximation and search space regularization with parameters shared among cells. Finally, we evaluated the performance of the model searched by NAS on the Sleep-EDF-20, Sleep-EDF-78 and SHHS datasets with an average accuracy of 82.7%, 80.0% and 81.9%, respectively. The experimental results show that the proposed NAS algorithm provides some reference for the subsequent automatic design of networks for sleep classification.
更多查看译文
关键词
Sleep,Electroencephalography,Brain modeling,Feature extraction,Search problems,Training,Convolutional neural networks,Electroencephalogram (EEG),sleep stage classification,neural architecture search (NAS),bilevel optimization approximation
AI 理解论文
溯源树
样例
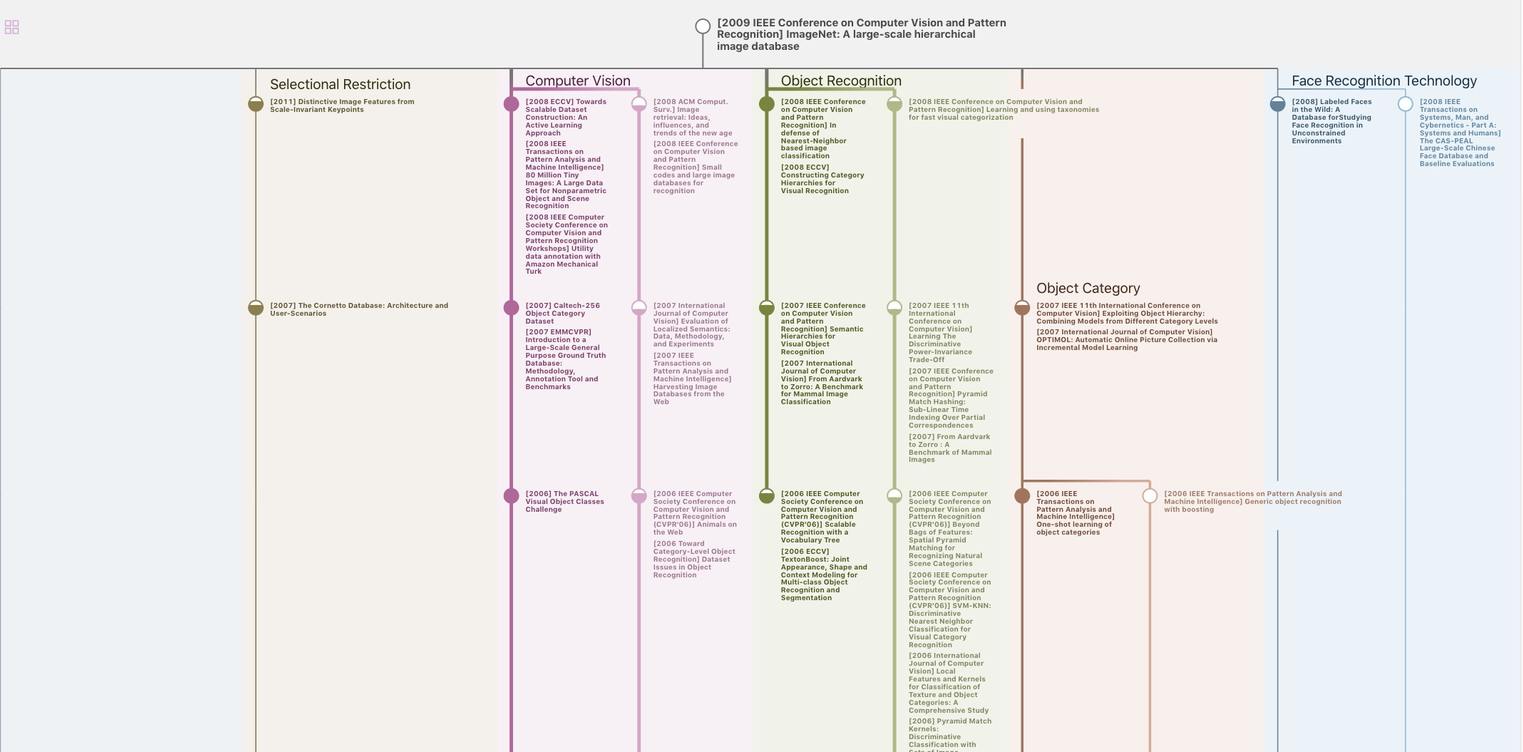
生成溯源树,研究论文发展脉络
Chat Paper
正在生成论文摘要