Self-adaptive seismic data reconstruction and denoising using dictionary learning based on morphological component analysis
FRONTIERS IN EARTH SCIENCE(2023)
摘要
Data reconstruction and data denoising are two critical preliminary steps in seismic data processing. Compressed Sensing states that a signal can be recovered by a series of solving algorithms if it is sparse in a transform domain, and has been well applied in the field of reconstruction, when, sparse representation of seismic data is the key point. Considering the complexity and diversity of seismic data, a single mathematical transformation will lead to incomplete sparse expression and bad restoration effects. Morphological Component Analysis (MCA) decomposes a signal into several components with outstanding morphological features to approximate the complex internal data structure. However, the representation ability of combined dictionaries is constrained by the number of dictionaries, and cannot be self-adaptively matched with the data features. Dictionary learning overcomes the limitation of fixed base function by training dictionaries that are fully suitable for processed data, but requires huge amount of time and considerable hardware cost. To solve the above problems, a new dictionary library (K-Singluar Value Decomposition learning dictionary and Discrete Cosine Transform dictionary) is hereby proposed based on the efficiency of fixed base dictionary and the high precision of learning dictionary. The self-adaptive sparse representation is achieved under the Morphological Component Analysis framework and is successfully applied to the reconstruction and denoising of seismic data. Real data tests have proved that the proposed method performs better than single mathematical transformation and other combined dictionaries.
更多查看译文
关键词
compressed sensing,dictionary learning,morphological component analysis,seismic data reconstruction,seismic data denoising
AI 理解论文
溯源树
样例
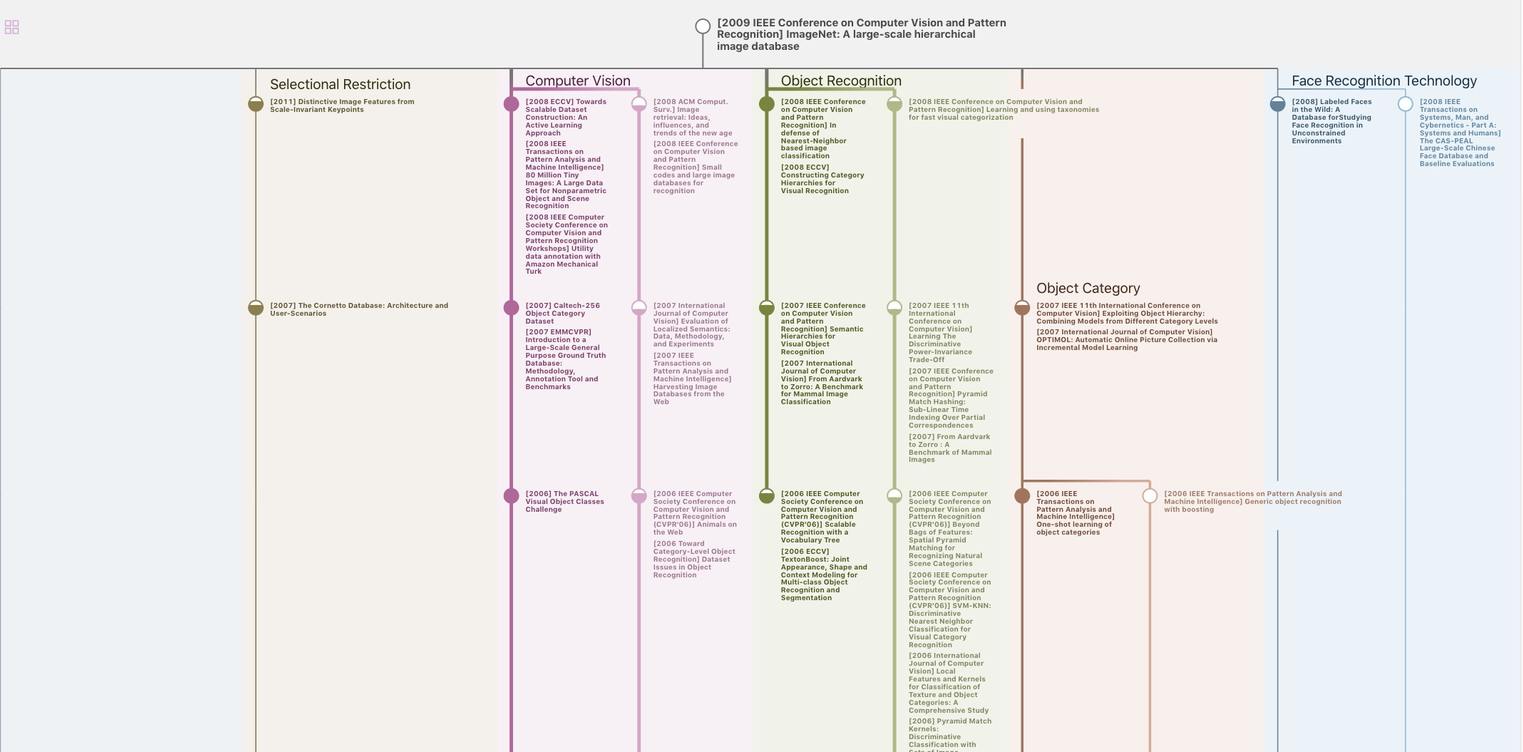
生成溯源树,研究论文发展脉络
Chat Paper
正在生成论文摘要