AAIA: an efficient aggregation scheme against inverting attack for federated learning
INTERNATIONAL JOURNAL OF INFORMATION SECURITY(2023)
摘要
Federated learning is emerged as an attractive paradigm regarding the data privacy problem, clients train the deep neural network on their local datasets, there is no need to upload their local data to a center server, and gradients are shared instead. However, recent studies show that adversaries can reconstruct the training images at high resolution from the gradients, such a break of data privacy is possible even in trained deep networks. To protect data privacy, a secure aggregation scheme against inverting attack is proposed for federated learning. The gradients are encrypted before sharing, and an adversary is unable to launch various attacks based on gradients. To improve the efficiency of data aggregation schemes, a new way of building shared keys is proposed, and a client build shared keys with 2 a other clients, but not all the clients in the system. Besides, the gradient inversion attacks are also tested, and a gradient inversion attack is proposed, which enable the adversary to reconstruct the training data based on gradient. The simulation results show the proposed scheme can protect an honest but curious parameter server from reconstructing the training data.
更多查看译文
关键词
Federated learning,Deep learning,Data privacy,Data security
AI 理解论文
溯源树
样例
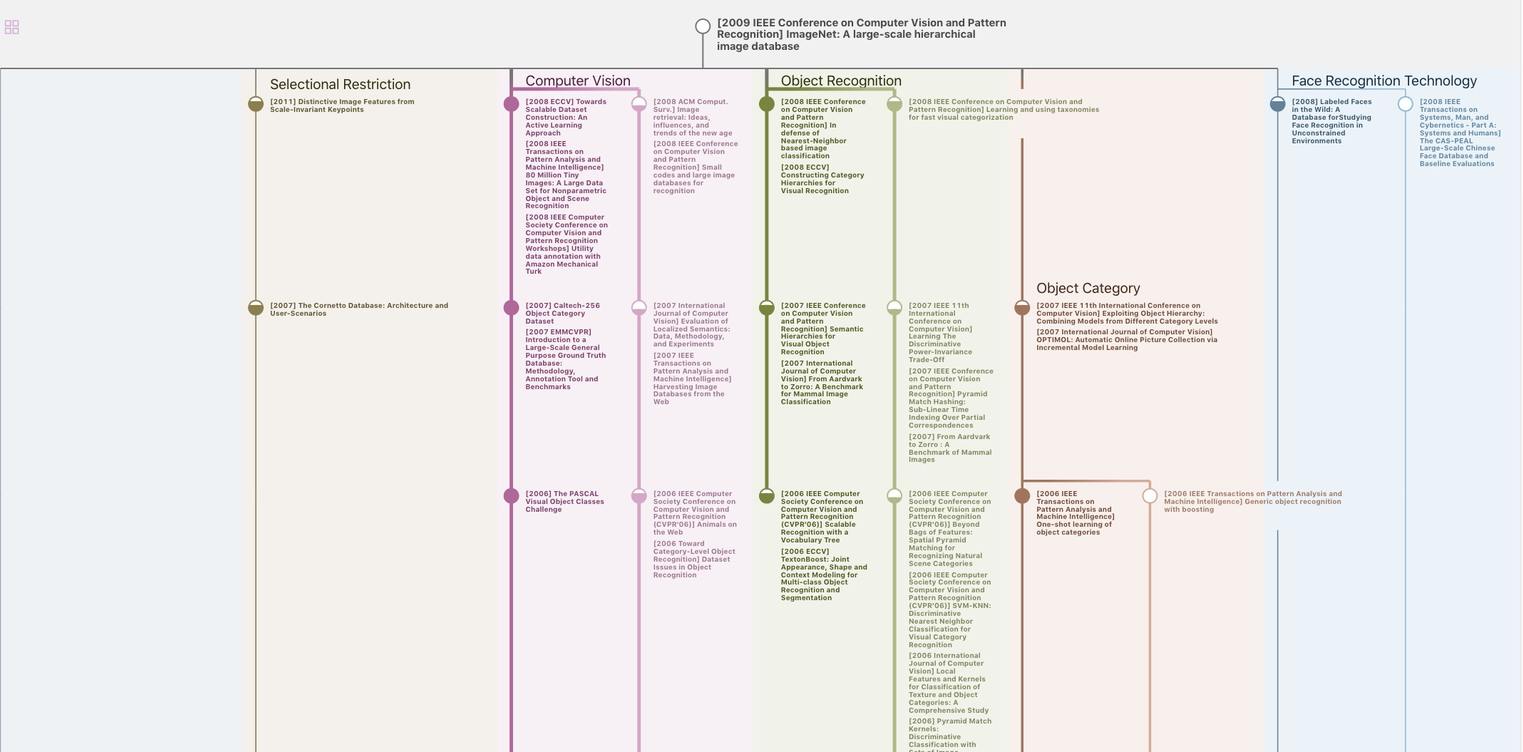
生成溯源树,研究论文发展脉络
Chat Paper
正在生成论文摘要