Augmented FCN: rethinking context modeling for semantic segmentation
SCIENCE CHINA-INFORMATION SCIENCES(2023)
摘要
The effectiveness of modeling contextual information has been empirically shown in numerous computer vision tasks. In this paper, we propose a simple yet efficient augmented fully convolutional network (AugFCN) by aggregating content- and position-based object contexts for semantic segmentation. Specifically, motivated because each deep feature map is a global, class-wise representation of the input, we first propose an augmented nonlocal interaction (AugNI) to aggregate the global content-based contexts through all feature map interactions. Compared to classical position-wise approaches, AugNI is more efficient. Moreover, to eliminate permutation equivariance and maintain translation equivariance, a learnable, relative position embedding branch is then supportably installed in AugNI to capture the global position-based contexts. AugFCN is built on a fully convolutional network as the backbone by deploying AugNI before the segmentation head network. Experimental results on two challenging benchmarks verify that AugFCN can achieve a competitive 45.38% mIoU (standard mean intersection over union) and 81.9% mIoU on the ADE20K val set and Cityscapes test set, respectively, with little computational overhead. Additionally, the results of the joint implementation of AugNI and existing context modeling schemes show that AugFCN leads to continuous segmentation improvements in state-of-the-art context modeling. We finally achieve a top performance of 45.43% mIoU on the ADE20K val set and 83.0% mIoU on the Cityscapes test set.
更多查看译文
关键词
semantic segmentation,context modeling,long-range dependencies,attention mechanism
AI 理解论文
溯源树
样例
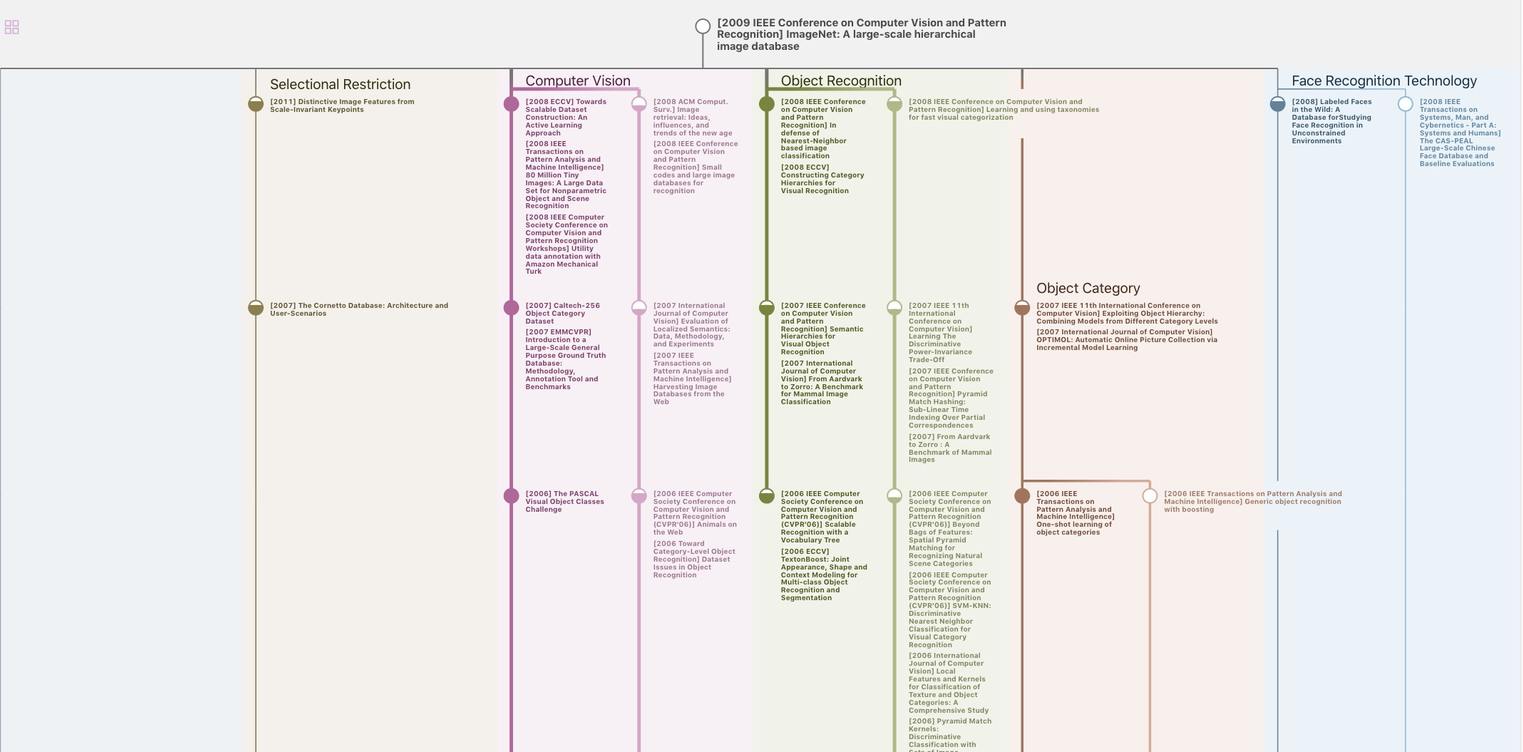
生成溯源树,研究论文发展脉络
Chat Paper
正在生成论文摘要