Smart Disease Detection System for Citrus Fruits Using Deep Learning with Edge Computing
SUSTAINABILITY(2023)
摘要
In recent decades, deep-learning dependent fruit disease detection and classification techniques have evinced outstanding results in technologically advanced horticulture investigation. Due to the comparatively limited image processing capabilities of edge computing devices, implementing deep learning methods in actual field scenarios is currently difficult. The use of intelligent machines in contemporary horticulture is being hampered by these restrictions, which are emerging as a new barrier. In this research, we present an efficient model for citrus fruit disease prediction. The proposed model utilizes the fusion of deep learning models CNN and LSTM with edge computing. The proposed model employs an enhanced feature-extraction mechanism, with a down-sampling approach, and then a feature-fusion subsystem to ensure significant recognition on edge computing devices with retaining citrus fruit disease detection accuracy. This research utilizes the online Kaggle and plan village dataset which contains 2950 citrus fruit images with disease categories black spots, cankers, scabs, Melanosis, and greening. The proposed model and existing model are tested with two features with pruning and without pruning and compared based on various performance measuring parameters, i.e., precision, recall, f-measure, and support. In the first phase experimental analysis is performed using Magnitude Based Pruning and in the second phase Magnitude Based Pruning with Post Quantization. The proposed CNN-LSTM model achieves an accuracy rate of 97.18% with Magnitude-Based Pruning and 98.25% with Magnitude-Based Pruning with Post Quantization, which is better as compared to the existing CNN method.
更多查看译文
关键词
citrus fruit disease,deep learning method,edge computing,pruning feature,CNN,LSTM
AI 理解论文
溯源树
样例
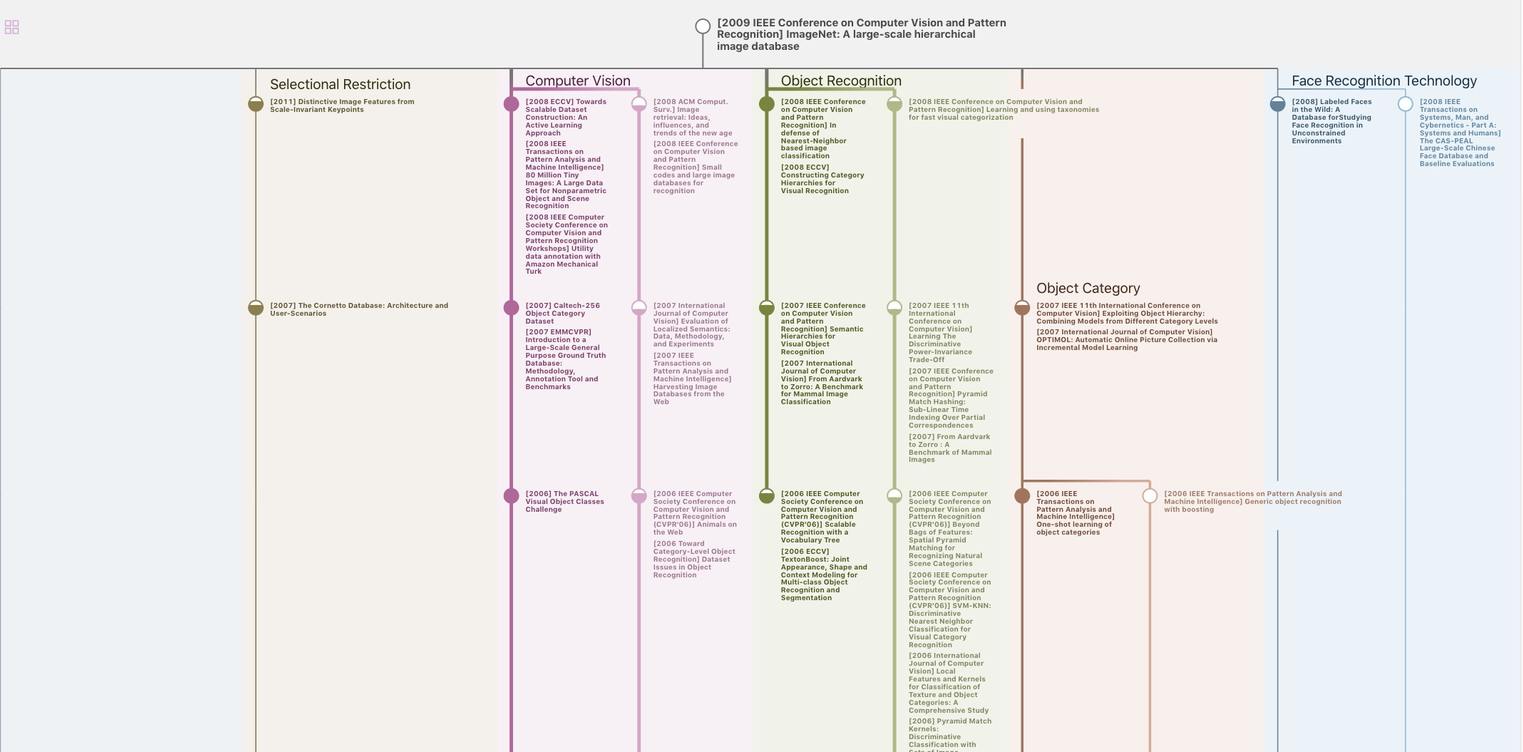
生成溯源树,研究论文发展脉络
Chat Paper
正在生成论文摘要