Deep learning estimation of modified Zernike coefficients for image point spread functions
arXiv (Cornell University)(2023)
摘要
Recovering the turbulence-degraded point spread function from a single intensity image is important for a variety of imaging applications. Here, a deep learning model based on a convolution neural network is applied to intensity images to predict a modified set of Zernike polynomial coefficients corresponding to wavefront aberrations in the pupil due to turbulence. The modified set assigns an absolute value to coefficients of even radial orders due to a sign ambiguity associated with this problem and is shown to be sufficient for specifying the intensity point spread function. Simulated image data of a point object and simple extended objects over a range of turbulence and detection noise levels are created for the learning model. The MSE results for the learning model show that the best prediction is found when observing a point object, but it is possible to recover a useful set of modified Zernike coefficients from an extended object image that is subject to detection noise and turbulence.
更多查看译文
关键词
zernike coefficients,deep learning
AI 理解论文
溯源树
样例
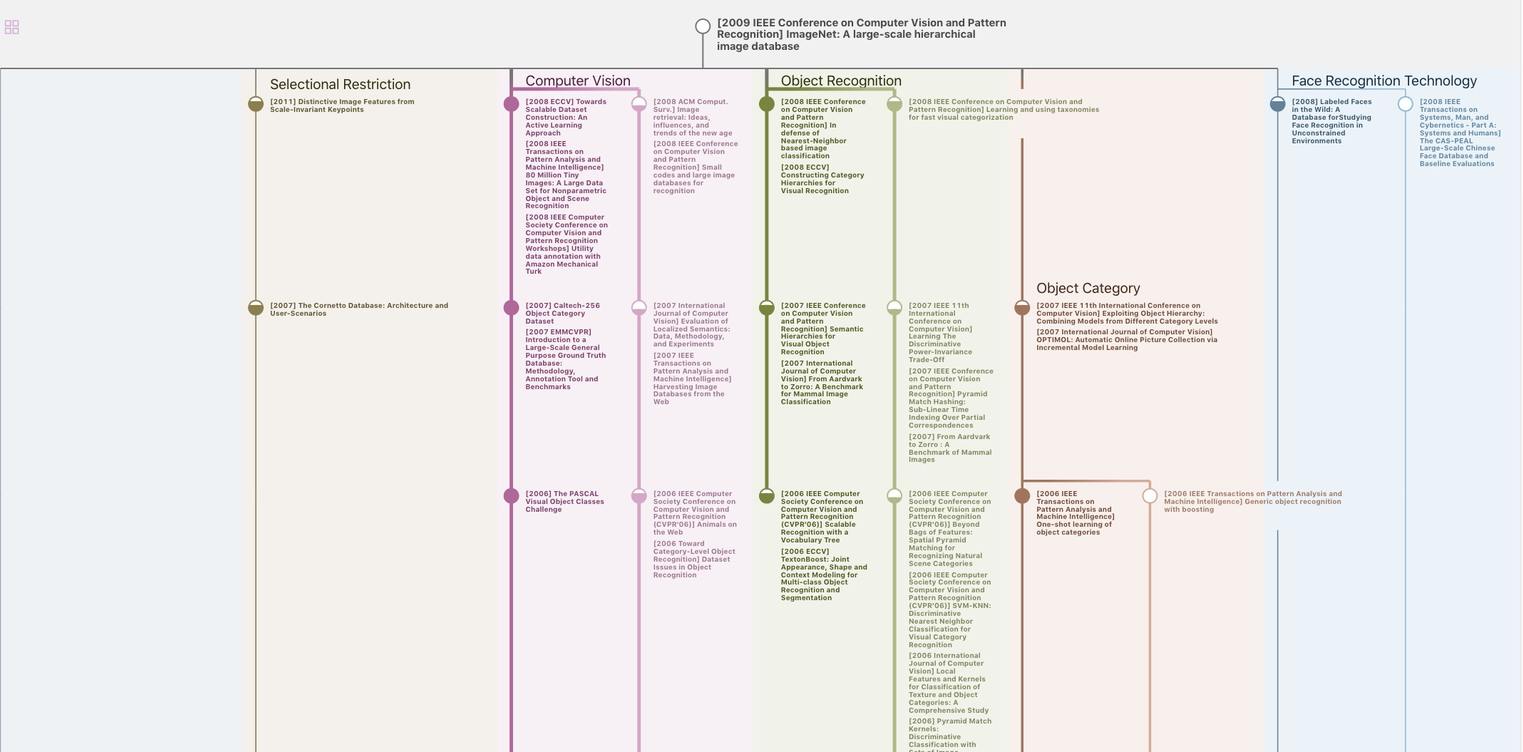
生成溯源树,研究论文发展脉络
Chat Paper
正在生成论文摘要