Large Language Models as Master Key: Unlocking the Secrets of Materials Science with GPT
CoRR(2023)
摘要
This article presents a new NLP task called structured information inference (SIS) to address the complexities of information extraction at the device level in materials science. We accomplished this task by finetuning GPT-3 on a exsiting perovskite solar cell FAIR dataset with 91.8 F1-score and we updated the dataset with all related scientific papers up to now. The produced dataset is formatted and normalized, enabling its direct utilization as input in subsequent data analysis. This feature will enable materials scientists to develop their own models by selecting high-quality review papers within their domain. Furthermore, we designed experiments to predict PCE and reverse-predict parameters and obtained comparable performance with DFT, which demonstrates the potential of large language models to judge materials and design new materials like a materials scientist.
更多查看译文
AI 理解论文
溯源树
样例
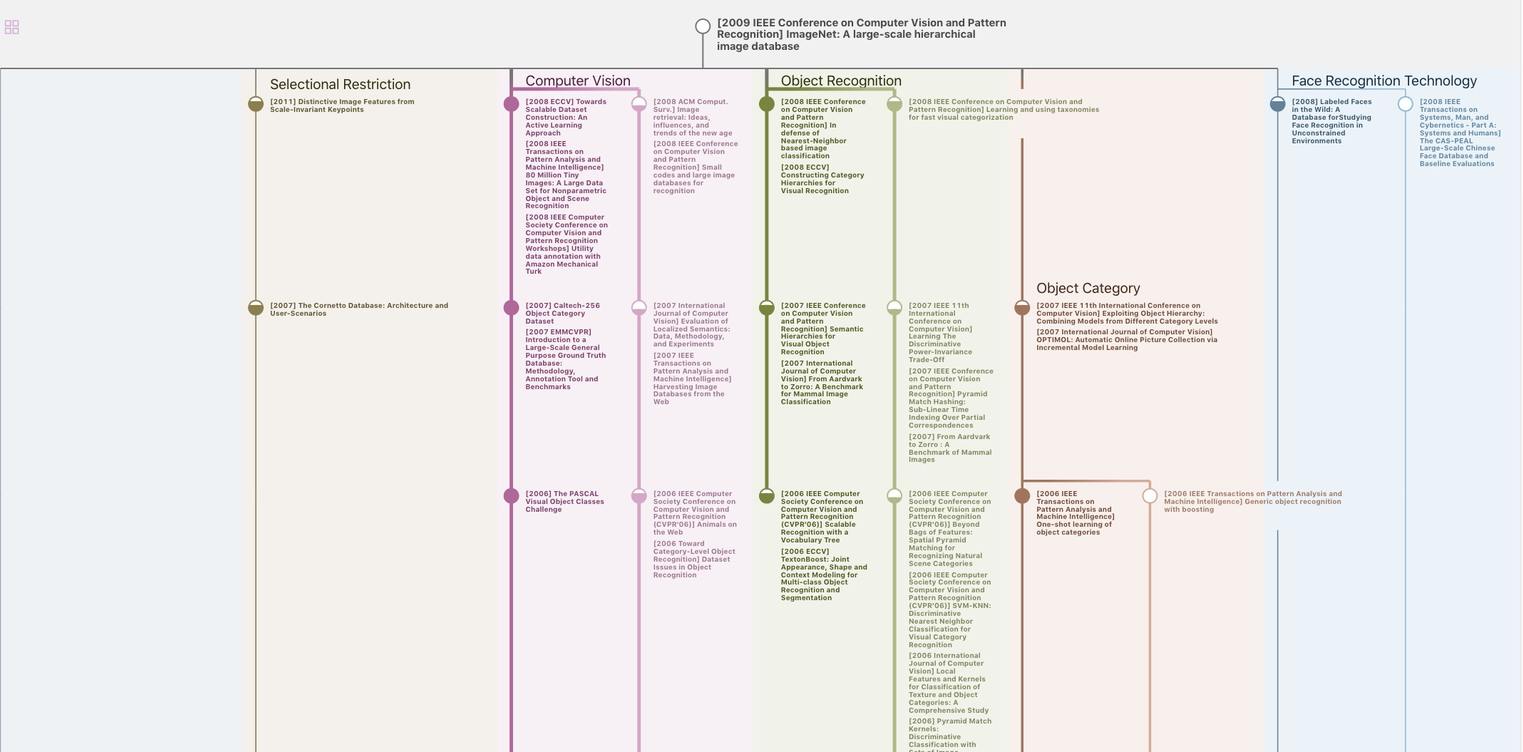
生成溯源树,研究论文发展脉络
Chat Paper
正在生成论文摘要