Online Learning with Adversaries: A Differential-Inclusion Analysis
2023 62ND IEEE CONFERENCE ON DECISION AND CONTROL, CDC(2023)
摘要
We introduce an observation-matrix-based framework for fully asynchronous online Federated Learning (FL) with adversaries. In this work, we demonstrate its effectiveness in estimating the mean of a random vector. Our main result is that the proposed algorithm almost surely converges to the desired mean mu. This makes ours the first asynchronous FL method to have an a.s. convergence guarantee in the presence of adversaries. We derive this convergence using a novel differential-inclusion-based two-timescale analysis. Two other highlights of our proof include (a) the use of a novel Lyapunov function to show that mu is the unique global attractor for our algorithm's limiting dynamics, and (b) the use of martingale and stopping-time theory to show that our algorithm's iterates are almost surely bounded.
更多查看译文
AI 理解论文
溯源树
样例
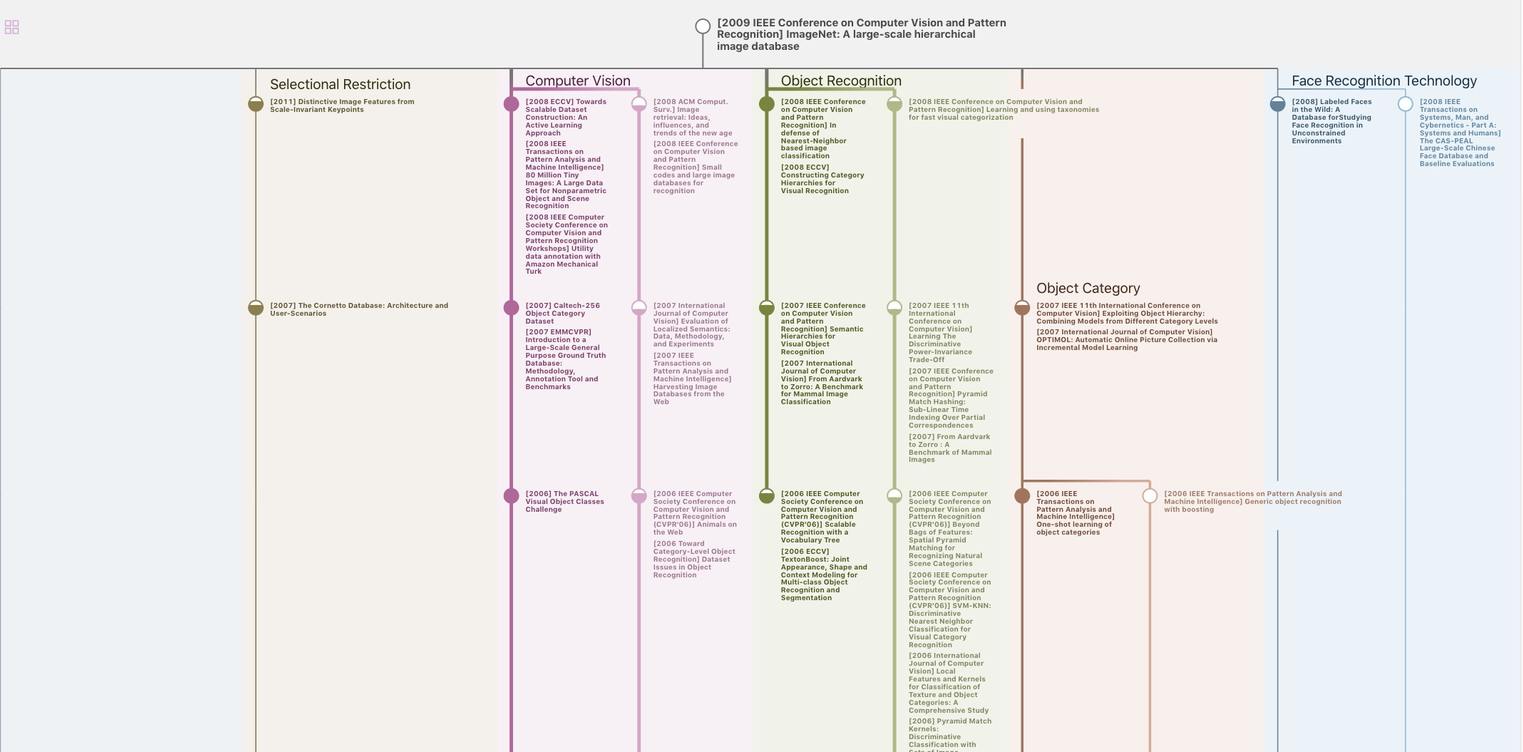
生成溯源树,研究论文发展脉络
Chat Paper
正在生成论文摘要