Functional Knowledge Transfer with Self-supervised Representation Learning
2023 IEEE INTERNATIONAL CONFERENCE ON IMAGE PROCESSING, ICIP(2023)
摘要
This work investigates the unexplored usability of self-supervised representation learning in the direction of functional knowledge transfer. In this work, functional knowledge transfer is achieved by joint optimization of self-supervised learning pseudo task and supervised learning task, improving supervised learning task performance. Recent progress in self-supervised learning uses a large volume of data, which becomes a constraint for its applications on small-scale datasets. This work shares a simple yet effective joint training framework that reinforces human-supervised task learning by learning self-supervised representations just-in-time and vice versa. Experiments on three public datasets from different visual domains, Intel Image, CIFAR, and APTOS, reveal a consistent track of performance improvements on classification tasks during joint optimization. Qualitative analysis also supports the robustness of learnt representations. Source code and trained models are available on GitHub (1).
更多查看译文
关键词
self-supervised learning,functional knowledge transfer,joint training,representation learning,computer vision
AI 理解论文
溯源树
样例
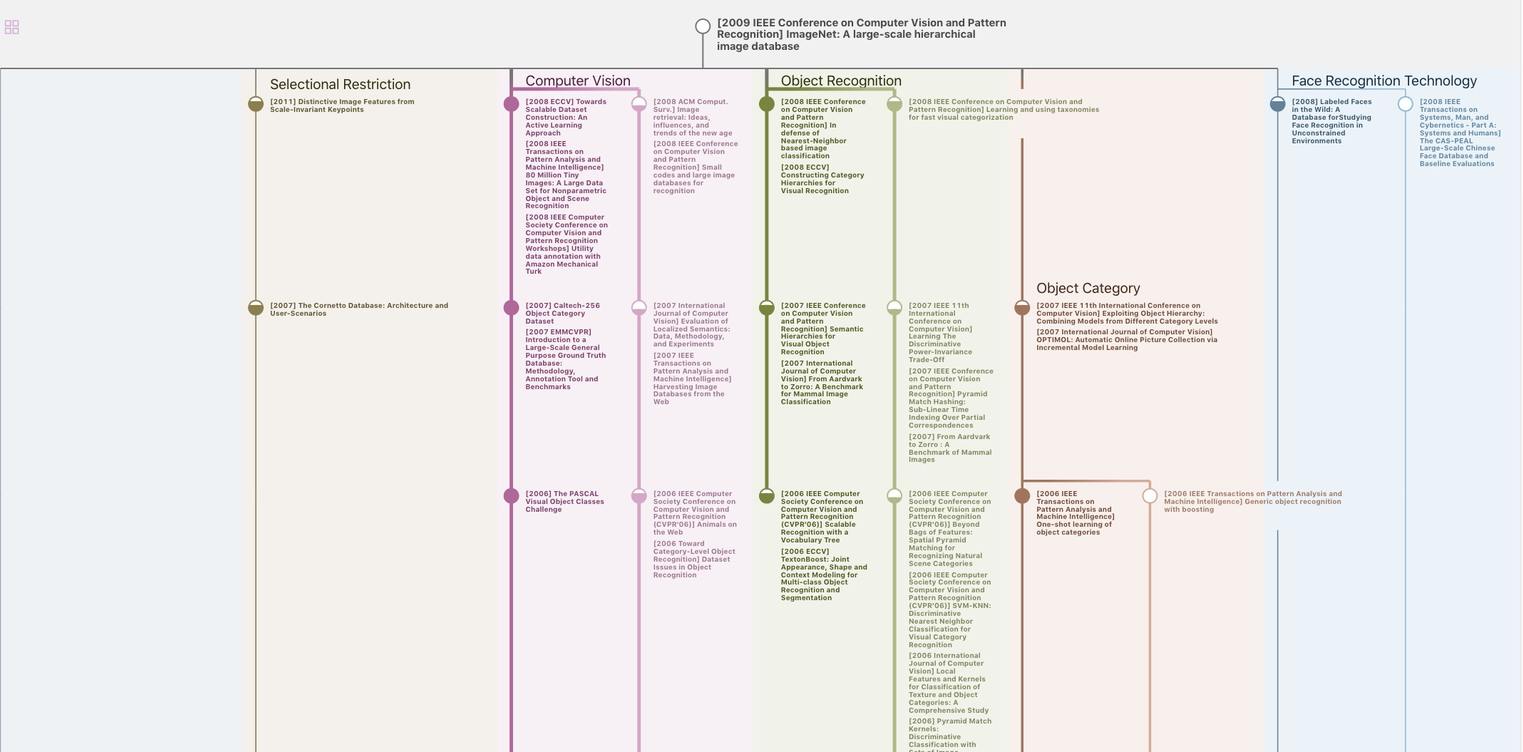
生成溯源树,研究论文发展脉络
Chat Paper
正在生成论文摘要