Click-Aware Structure Transfer with Sample Weight Assignment for Post-Click Conversion Rate Estimation
MACHINE LEARNING AND KNOWLEDGE DISCOVERY IN DATABASES: RESEARCH TRACK, ECML PKDD 2023, PT V(2023)
摘要
Post-click Conversion Rate (CVR) prediction task plays an essential role in industrial applications, such as recommendation and advertising. Conventional CVR methods typically suffer from the data sparsity problem as they rely only on samples where the user has clicked. To address this problem, researchers have introduced the method of multi-task learning, which utilizes non-clicked samples and shares feature representations of the Click-Through Rate (CTR) task with the CVR task. However, it should be noted that the CVR and CTR tasks are fundamentally different and may even be contradictory. Therefore, introducing a large amount of CTR information without distinction may drown out valuable information related to CVR. This phenomenon is called the curse of knowledge problem in this paper. To tackle this issue, we argue that a trade-off should be achieved between the introduction of large amounts of auxiliary information and the protection of valuable information related to CVR. Hence, we propose a Click-aware Structure Transfer model with sample Weight Assignment, abbreviated as CSTWA. It pays more attention to the latent structure information, which could refine the input information related to CVR, instead of directly sharing feature representations. Meanwhile, to capture the representation conflict between CTR and CVR, we calibrate the representation layer and reweight the discriminant layer to excavate the click bias information from the CTR tower. Moreover, it incorporates a sample weight assignment algorithm biased towards CVR modeling, to make the knowledge from CTR would not mislead the CVR. Extensive experiments on industrial and public datasets have demonstrated that CSTWA significantly outperforms widely used and competitive models.
更多查看译文
关键词
Post-click Conversion Rate,Curse of Knowledge
AI 理解论文
溯源树
样例
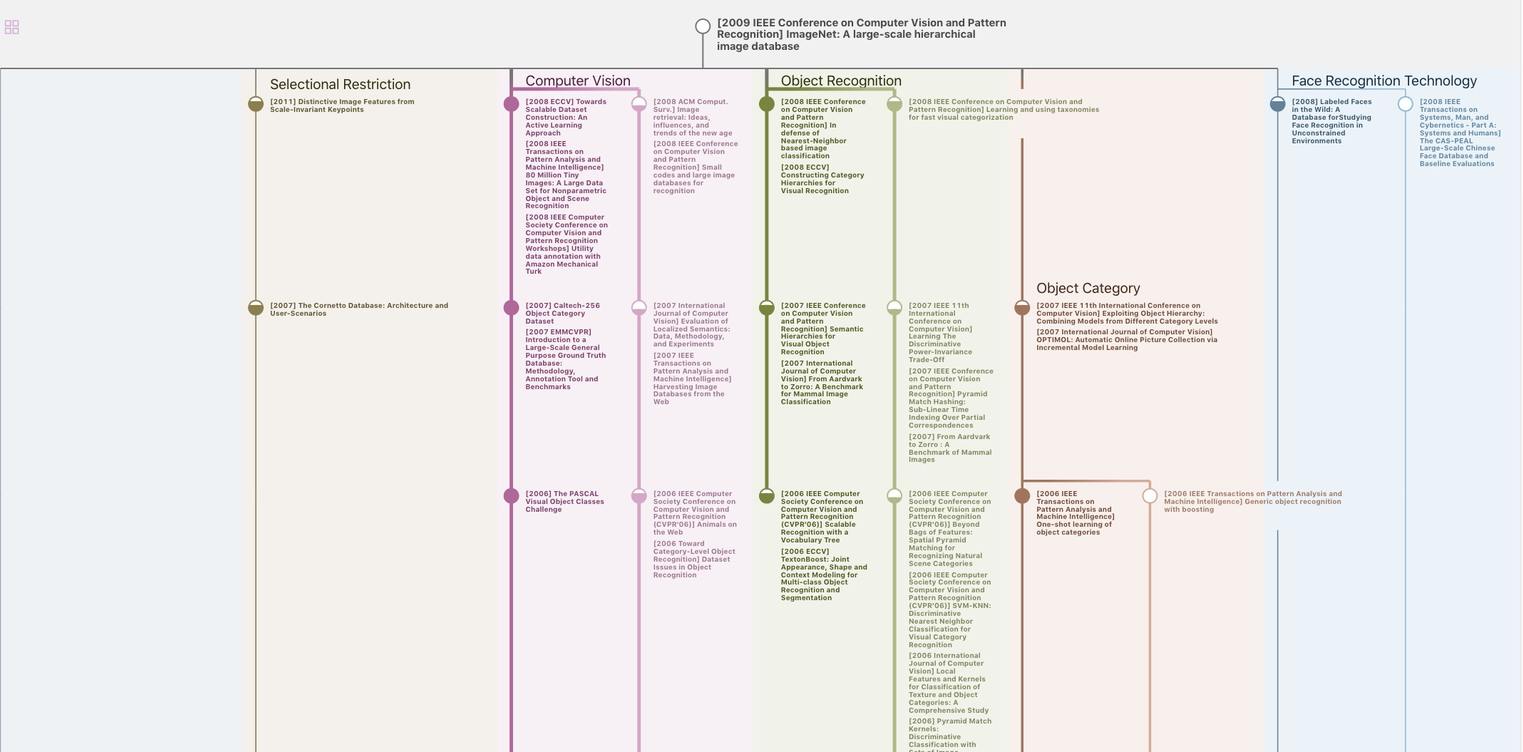
生成溯源树,研究论文发展脉络
Chat Paper
正在生成论文摘要