On the impact of regularization in data-driven predictive control
2023 62ND IEEE CONFERENCE ON DECISION AND CONTROL, CDC(2023)
摘要
Model predictive control (MPC) is a control strategy widely used in industrial applications. However, its implementation typically requires a mathematical model of the system being controlled, which can be a time-consuming and expensive task. Data-driven predictive control (DDPC) methods offer an alternative approach that does not require an explicit mathematical model, but instead optimize the control policy directly from data. In this paper, we study the impact of two different regularization penalties on the closed-loop performance of a recently introduced data-driven method called.-DDPC. Moreover, we discuss the tuning of the related coefficients in different data and noise scenarios, to provide some guidelines for the end user.
更多查看译文
关键词
Data driven control,Predictive control for linear systems,Uncertain systems
AI 理解论文
溯源树
样例
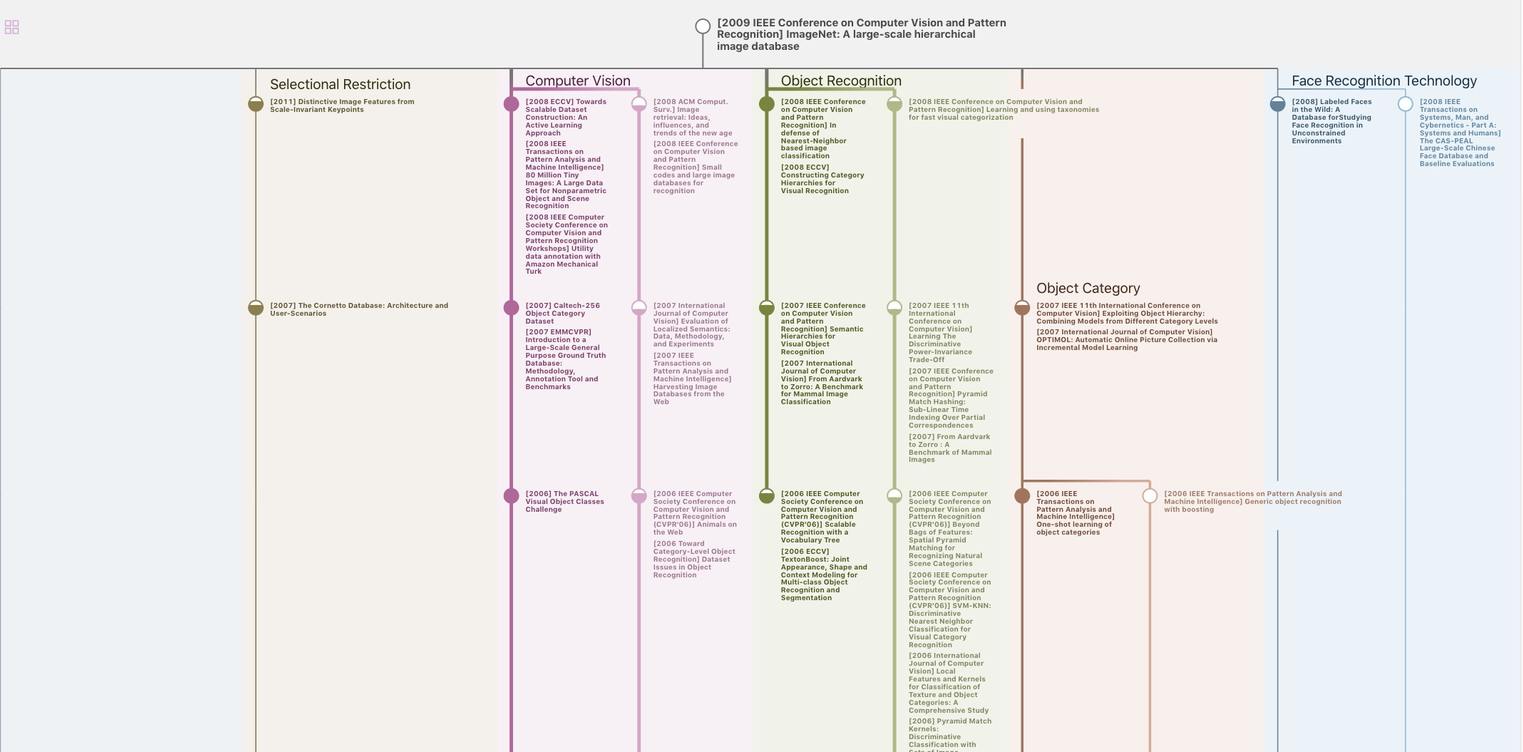
生成溯源树,研究论文发展脉络
Chat Paper
正在生成论文摘要