Diffusion map particle systems for generative modeling
CoRR(2023)
摘要
We propose a novel diffusion map particle system (DMPS) for generative modeling, based on diffusion maps and Laplacian-adjusted Wasserstein gradient descent (LAWGD). Diffusion maps are used to approximate the generator of the Langevin diffusion process from samples, and hence to learn the underlying data-generating manifold. On the other hand, LAWGD enables efficient sampling from the target distribution given a suitable choice of kernel, which we construct here via a spectral approximation of the generator, computed with diffusion maps. Numerical experiments show that our method outperforms others on synthetic datasets, including examples with manifold structure.
更多查看译文
关键词
generative modeling,diffusion
AI 理解论文
溯源树
样例
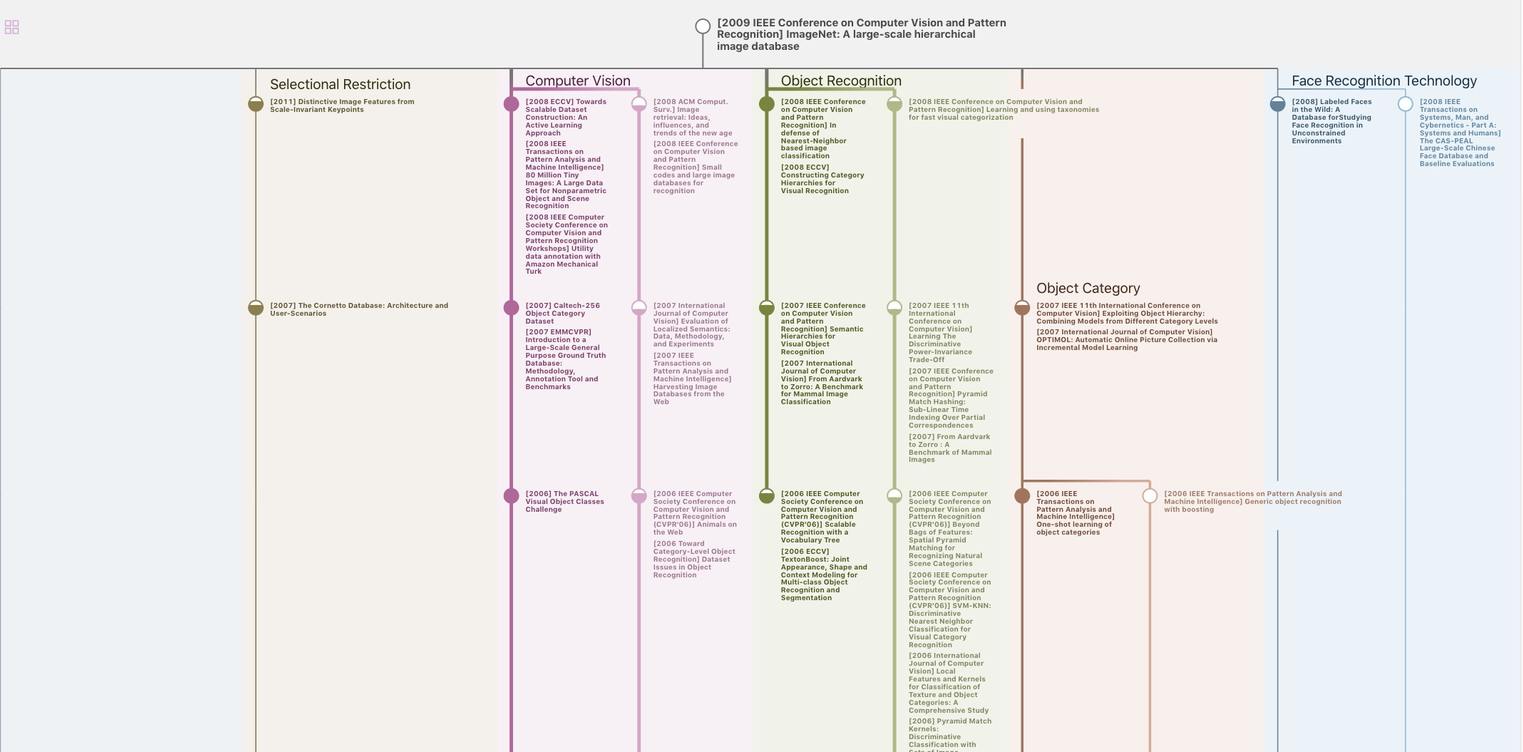
生成溯源树,研究论文发展脉络
Chat Paper
正在生成论文摘要