Constrained optimization of rank-one functions with indicator variables
Mathematical Programming(2024)
摘要
Optimization problems involving minimization of a rank-one convex function over constraints modeling restrictions on the support of the decision variables emerge in various machine learning applications. These problems are often modeled with indicator variables for identifying the support of the continuous variables. In this paper we investigate compact extended formulations for such problems through perspective reformulation techniques. In contrast to the majority of previous work that relies on support function arguments and disjunctive programming techniques to provide convex hull results, we propose a constructive approach that exploits a hidden conic structure induced by perspective functions. To this end, we first establish a convex hull result for a general conic mixed-binary set in which each conic constraint involves a linear function of independent continuous variables and a set of binary variables. We then demonstrate that extended representations of sets associated with epigraphs of rank-one convex functions over constraints modeling indicator relations naturally admit such a conic representation. This enables us to systematically give perspective formulations for the convex hull descriptions of these sets with nonlinear separable or non-separable objective functions, sign constraints on continuous variables, and combinatorial constraints on indicator variables. We illustrate the efficacy of our results on sparse nonnegative logistic regression problems.
更多查看译文
关键词
Mixed-integer nonlinear optimization,Indicator variables,Perspective function,Combinatorial constraints,Convex hull
AI 理解论文
溯源树
样例
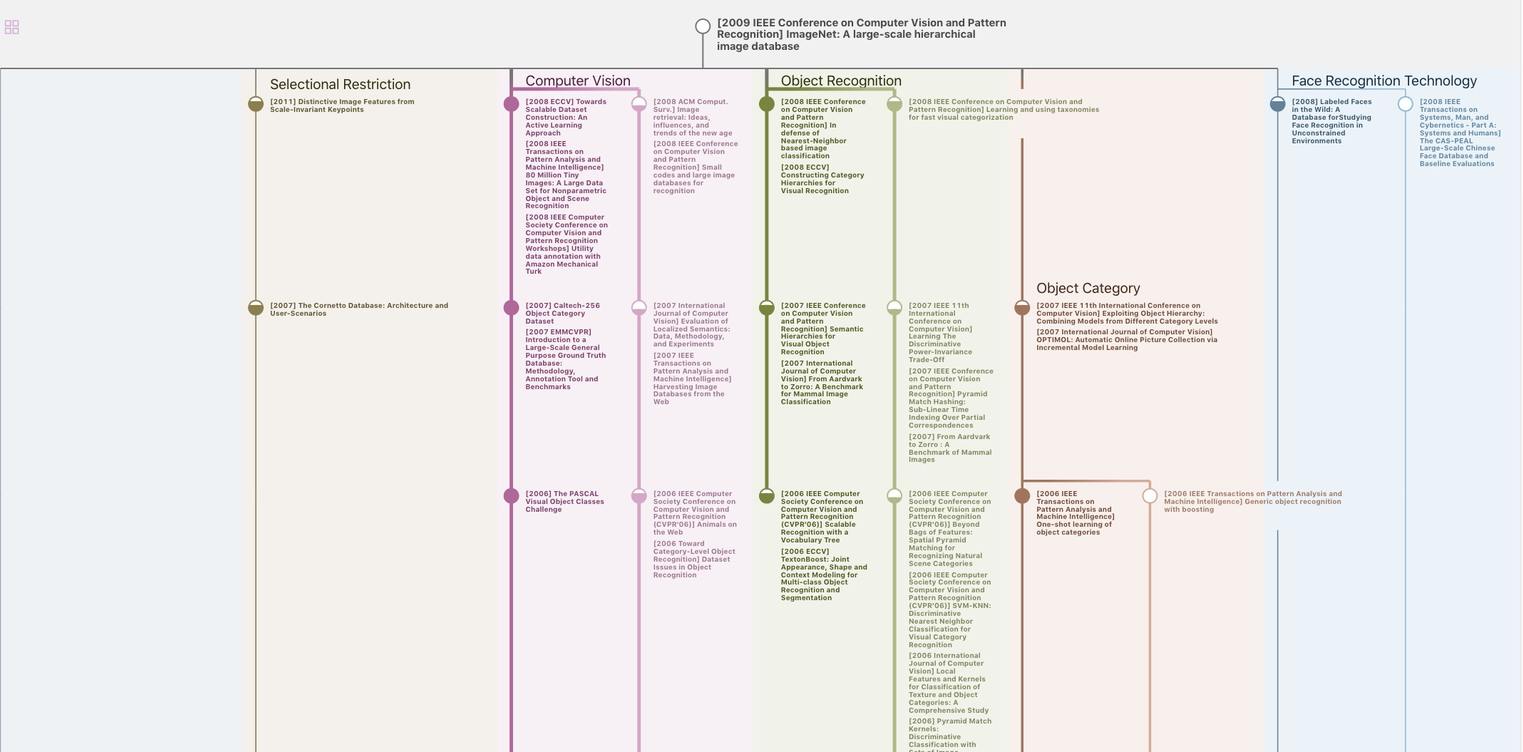
生成溯源树,研究论文发展脉络
Chat Paper
正在生成论文摘要