A two-head loss function for deep Average-K classification
CoRR(2023)
摘要
Average-K classification is an alternative to top-K classification in which the number of labels returned varies with the ambiguity of the input image but must average to K over all the samples. A simple method to solve this task is to threshold the softmax output of a model trained with the cross-entropy loss. This approach is theoretically proven to be asymptotically consistent, but it is not guaranteed to be optimal for a finite set of samples. In this paper, we propose a new loss function based on a multi-label classification head in addition to the classical softmax. This second head is trained using pseudo-labels generated by thresholding the softmax head while guaranteeing that K classes are returned on average. We show that this approach allows the model to better capture ambiguities between classes and, as a result, to return more consistent sets of possible classes. Experiments on two datasets from the literature demonstrate that our approach outperforms the softmax baseline, as well as several other loss functions more generally designed for weakly supervised multi-label classification. The gains are larger the higher the uncertainty, especially for classes with few samples.
更多查看译文
关键词
classification,loss,two-head
AI 理解论文
溯源树
样例
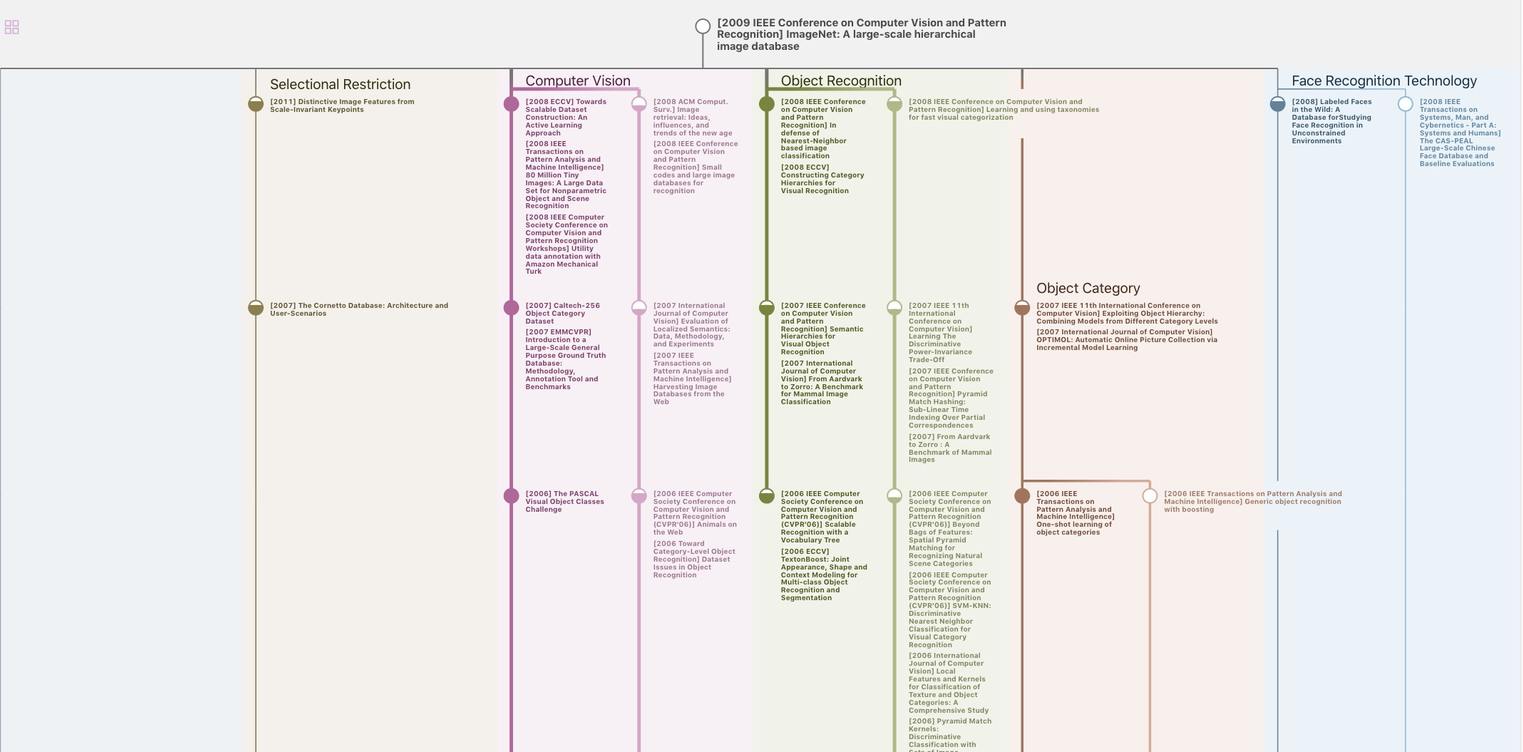
生成溯源树,研究论文发展脉络
Chat Paper
正在生成论文摘要