Exploratory Analysis of the Crash Severity between Vehicular Automation (SAE L2-5) with Multi-Source Data
arxiv(2023)
摘要
Autonomous driving tends to be an imperative technology to facilitate smart transportation, where vehicular automation plays a prominent role. To accelerate its development, the Society of Automotive Engineers (SAE) regularized the automation to different SAE levels (L0 - L5) and successfully promoted the research and products to improve road safety, efficiency, and convenience. Meanwhile, advanced driver assistance systems (ADAS) and automated driving systems (ADS) are widely developed to support vehicular automation and achieve success in automation technology. However, the safety risks always concern the developers and users and hinder the deployment of autonomous vehicles. Although the studies on the injury risk from autonomous vehicles are ongoing and extensive, limited current research compares ADAS and ADS, especially from a systematic perspective. We conduct this comparison study comprehensively. Different from existing works, we first incorporate multi-source data to ensure higher reliability of analysis results. Next, we conduct both descriptive statistics and statistical inference with random parameters multinomial logit model to analyze the interaction between investigated factors and observed crash data. Moreover, we compare the crash severity across different automation levels SAE L2-5 to further reveal the interaction between different factors. Given the analysis results, we find that different factors impact the injury severity between ADS and ADAS. The crashes from ADAS are more correlated to lightning, surface, the object collided with, and the impact area. The ADS crashes are more associated with road type, drive type, impact area, and weather conditions. Our findings provide the insights into safety outcomes of current ADS and ADAS, which helps stakeholders better explore automated vehicle safety for accelerating the deployment of autonomous vehicles.
更多查看译文
AI 理解论文
溯源树
样例
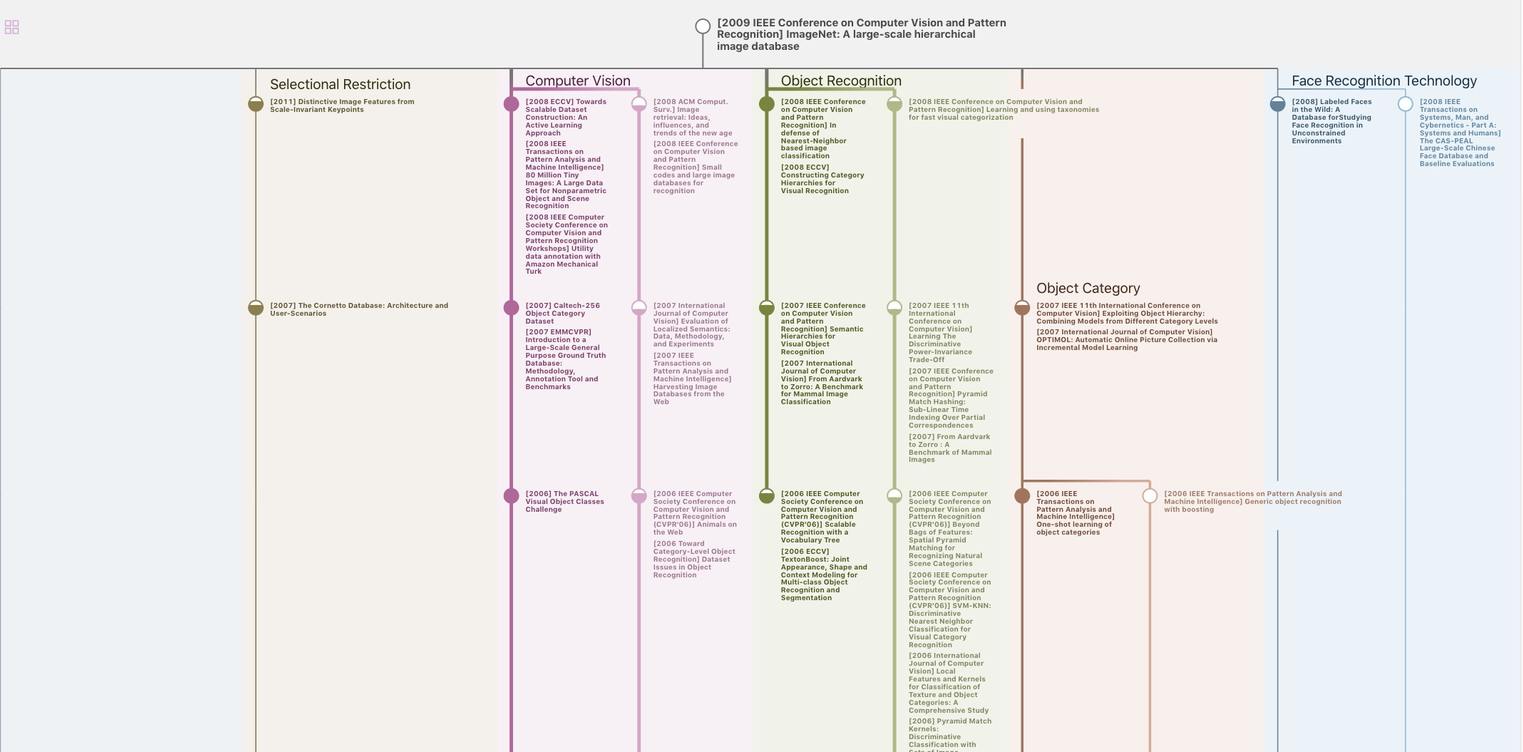
生成溯源树,研究论文发展脉络
Chat Paper
正在生成论文摘要