Data-Driven Covariance Steering Control Design
2023 62ND IEEE CONFERENCE ON DECISION AND CONTROL, CDC(2023)
摘要
This paper studies the problem of steering the distribution of a linear time-invariant system from an initial normal distribution to a terminal normal distribution under no knowledge of the system dynamics. This data-driven control framework uses data collected from the input and the state and utilizes the seminal work by Willems et al. to construct a databased parametrization of the mean and the covariance control problems. These problems are then solved to optimality as convex programs using standard techniques from the covariance control literature. We also discuss the equivalence of indirect and direct data-driven covariance steering designs, as well as a regularized version of the problem that provides a balance between the two. We illustrate the proposed framework through a set of randomized trials. We then analyze the robustness properties of the data-free and data-driven covariance steering methods and demonstrate the trade-offs between performance and optimality among these methods in the presence of data corrupted with exogenous noise.
更多查看译文
AI 理解论文
溯源树
样例
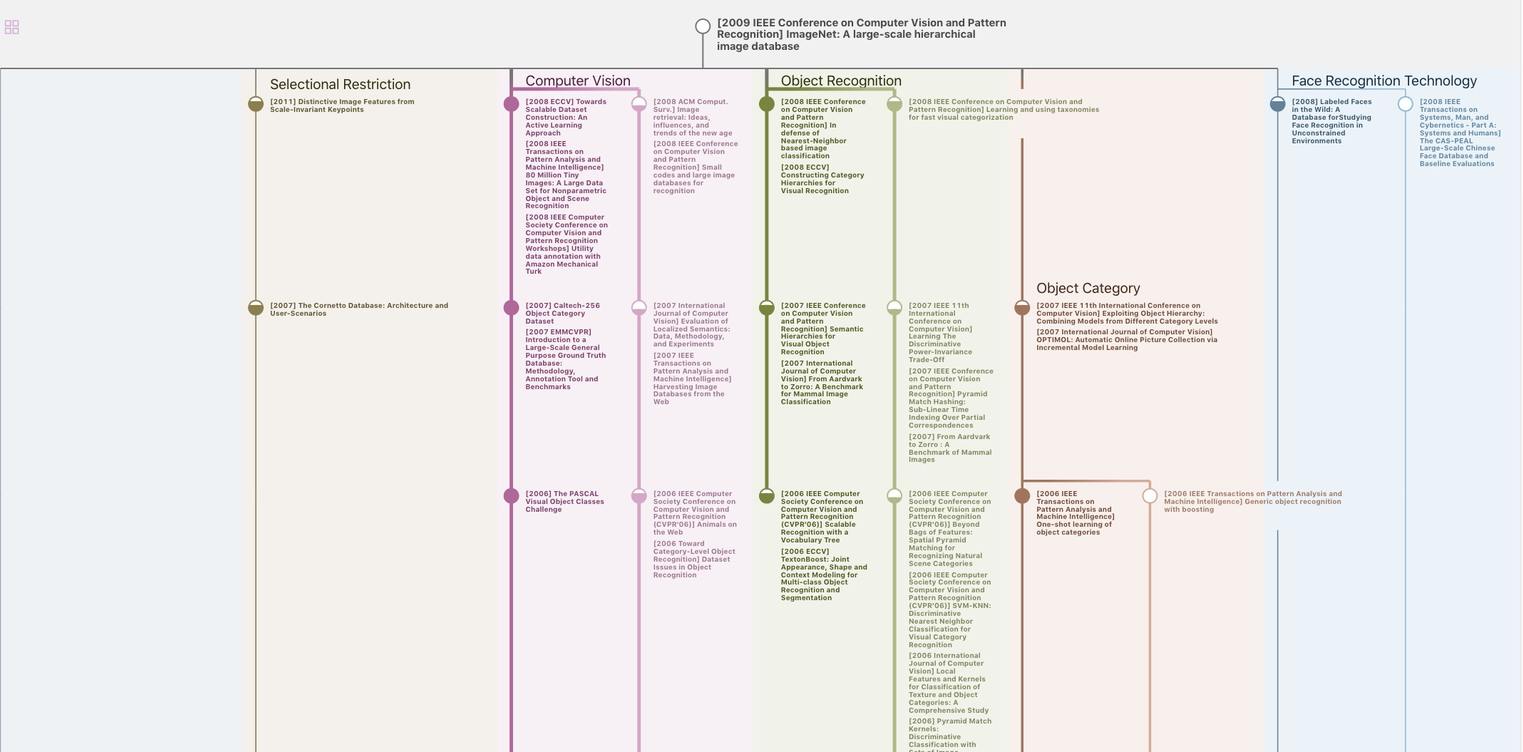
生成溯源树,研究论文发展脉络
Chat Paper
正在生成论文摘要