Estimation of ECG Parameters via Deep Learning based ECG Segmentation Tool for Non-invasive Detection of Glycaemic Events
crossref(2023)
摘要
The ECG segmentation tool has been pivotal in determination of fidu-cial points of the ECG beat which can be used to determine the ECG parameters representing the ECG beat and short-term ECG signal. Most of existing studies suggest the filtering of the ECG signal followed by thresholding via rule-based approach. However, these approaches are highly dependent on certain aspects such as signal noise, inter/intra subject variability across segments constructing the ECG beat (e.g., P, QRS and T). Recently, deep learning methods have been evolved due to their capability to represent the signal at micro level and ability to perform classification based on their spatial as well as temporal context. In this study, we implement the attention based Convolutional Bidirectional Long Short Term Memory (Conv-BiLSTM) network which can perform ECG segmentation followed by detection of fiducial points based on local beat features and temporal sequencing while correlating ECG beat across different positions. The perfor-mance of ECG segmentation has been tested against QT database for fiducial point detection sensitivity which found to be 99.41%. The ECG parameters have been compared on the D1NAMO dataset for Type 1 diabetes patient for glycae-mic event detection. The results show significant correlation of the ECG param-eters with the blood glucose levels and high variance across different glycaemic events.
更多查看译文
AI 理解论文
溯源树
样例
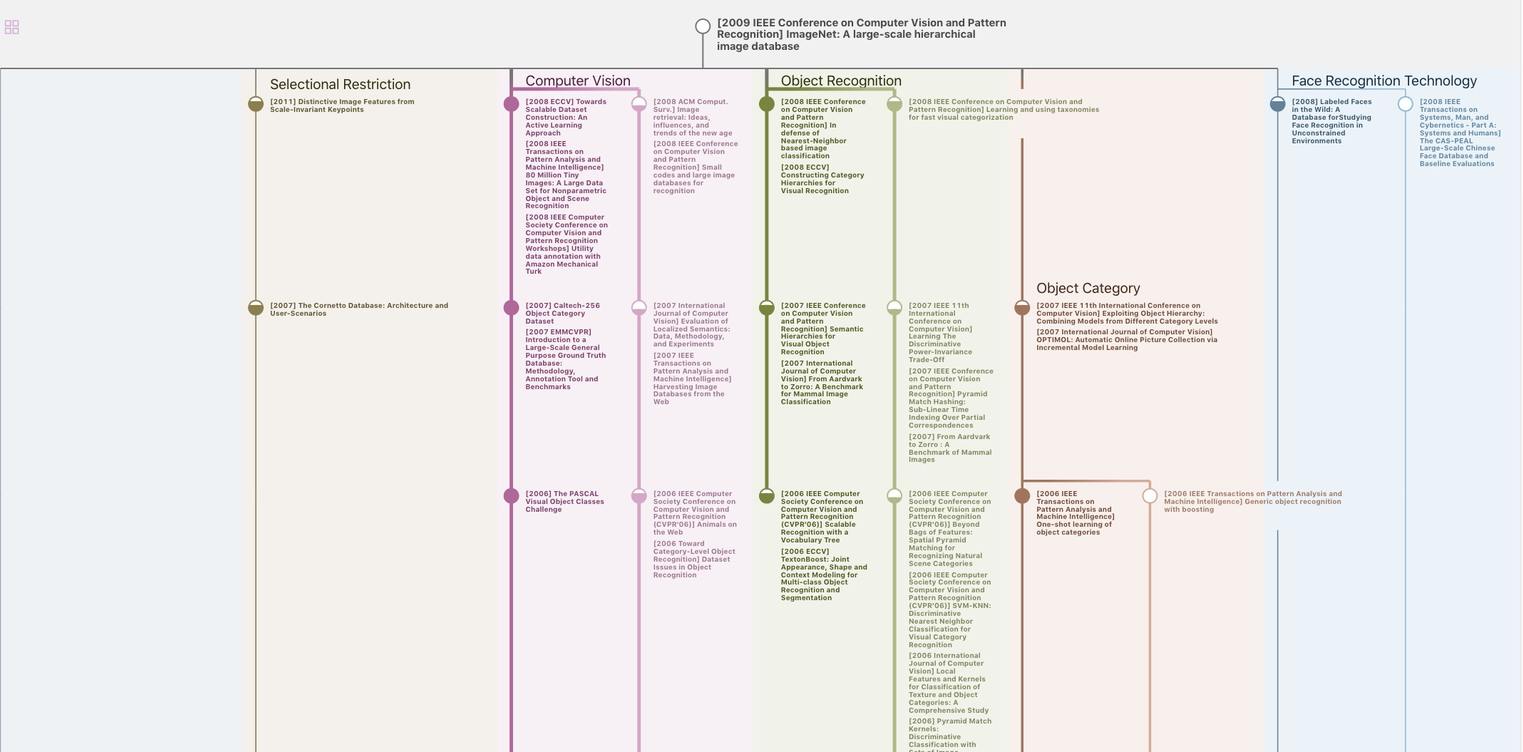
生成溯源树,研究论文发展脉络
Chat Paper
正在生成论文摘要