Image Classification Based on Layered Gradient Clipping Under Differential Privacy.
IEEE Access(2023)
摘要
Convolutional neural networks (CNNs) are widely used in the field of image classification. At the same time, users face the risk of privacy leakage because adversaries can reverse private information from the training parameters of CNNs. Adding Gaussian noise to the training parameters is an effective means to prevent adversaries from stealing private, but this tends to reduce the utility of the models. Therefore, how to find a balance between privacy and utility has become a hot research topic. In this paper, to improve the image classification ability of CNN models under differential privacy protection, we propose an image classification algorithm based on layered gradient clipping under differential privacy, ICGC-DP for short. Firstly, the gradient tensor is layered according to the neural network model. Secondly, for each layered gradient tensor, the median of L-2 norms is used as the clipping threshold. Moreover, to prevent the sensitivity from converging to zero, we add a bound on the sensitivity to ensure that all gradients can be protected by differential privacy. To further improve the classification utility of ICGC-DP, we design an adaptive weighted fusion module for it. The module assigns weights to prediction tensors according to the variance between them. We conduct comprehensive experiments on the Mnist, FashionMnist and CIFAR10 datasets, respectively. The experimental results show that, when the privacy budget epsilon = 2.0, which indicates that the algorithm adds a large noise, ICGC-DP achieves 97.36%, 88.72% and 72.63% classification accuracy for the Minist, FasionMnist and CIFAR10 datasets, respectively; when the privacy budget epsilon = 8.0, which means the algorithm adds less noise, the classification accuracy of ICGC-DP for Minist, FasionMnist and CIFAR10 datasets reaches 97.81%, 89.49% and 74.41%, respectively.
更多查看译文
关键词
Privacy preservation,deep learning,differential privacy,gradient clipping
AI 理解论文
溯源树
样例
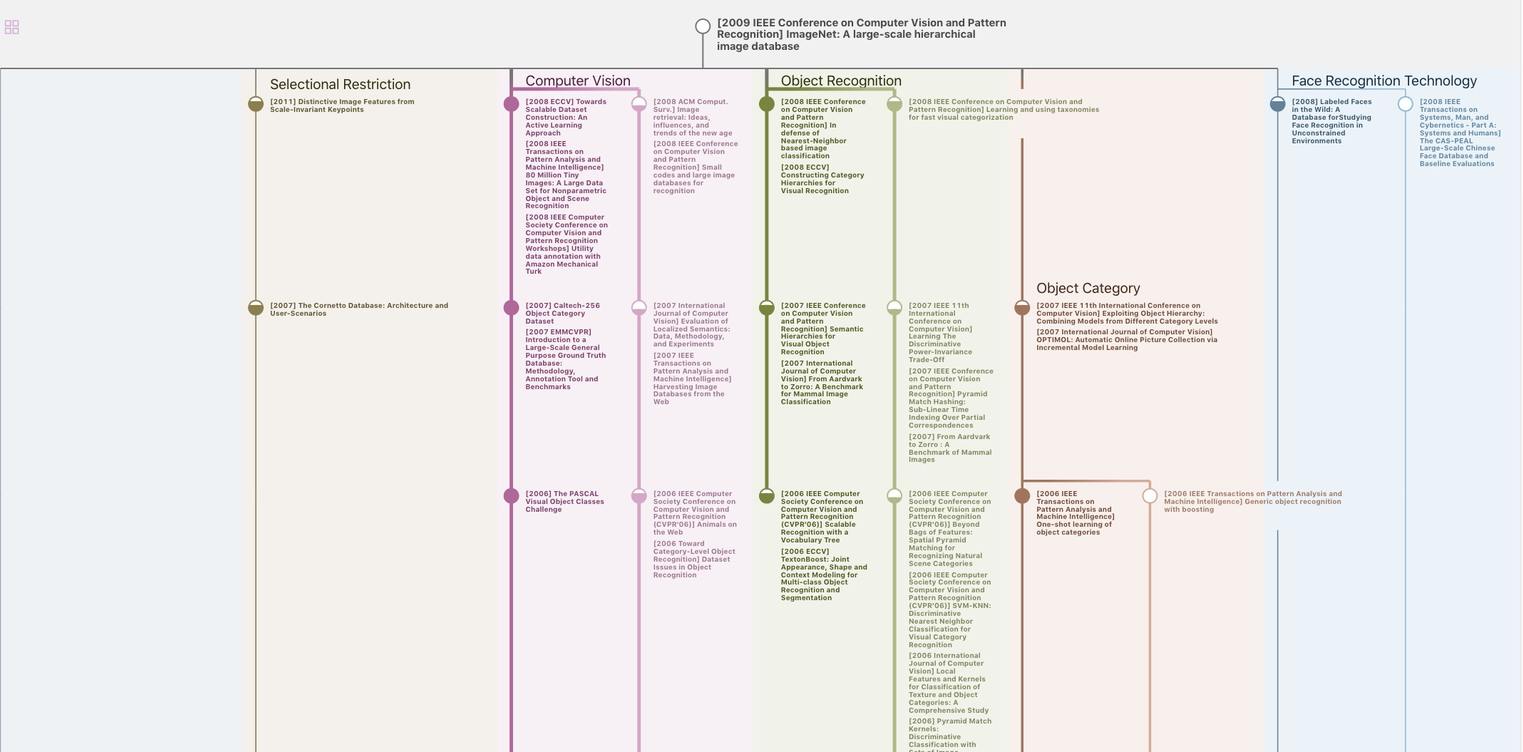
生成溯源树,研究论文发展脉络
Chat Paper
正在生成论文摘要