A Twinning Memory Bare-Bones Particle Swarm Optimization Algorithm for No-Linear Functions.
IEEE Access(2023)
摘要
Been trapped by local minimums is an important problem in no-linear optimization problems, which is blocking evolutionary algorithms to find the global optimum. Normally, to increase the optimization accuracy, evolutionary algorithms implement search around the best individual. However, overuse of information from a single individual can lead to a rapid diversity losing of the population, and thus reduce the search ability. To overcome this problem, a twinning memory bare-bones particle swarm optimization (TMBPSO) algorithm is presented in this work. The TMBPSO contains a twining memory storage mechanism (TMSM) and a multiple memory retrieval strategy (MMRS). The TMSM enables an extra storage space to extend the search ability of the particle swarm and the MMRS enhances the local minimum escaping ability of the particle swarm. The particle swarm is endowed with the ability of self-rectification by the cooperation of the TMSM and the MMRS. To verify the search ability of the TMBPSO, the CEC2017 benchmark functions and five state-of-the-art population-based optimization algorithms are selected in experiments. Finally, experimental results confirmed that the TMBPSO can obtain high accurate results for no-linear functions.
更多查看译文
关键词
Particle swarm optimization,Optimization,Statistics,Sociology,Heuristic algorithms,Gaussian distribution,Convergence,Twinning memory,particle swarm optimization,self-rectification
AI 理解论文
溯源树
样例
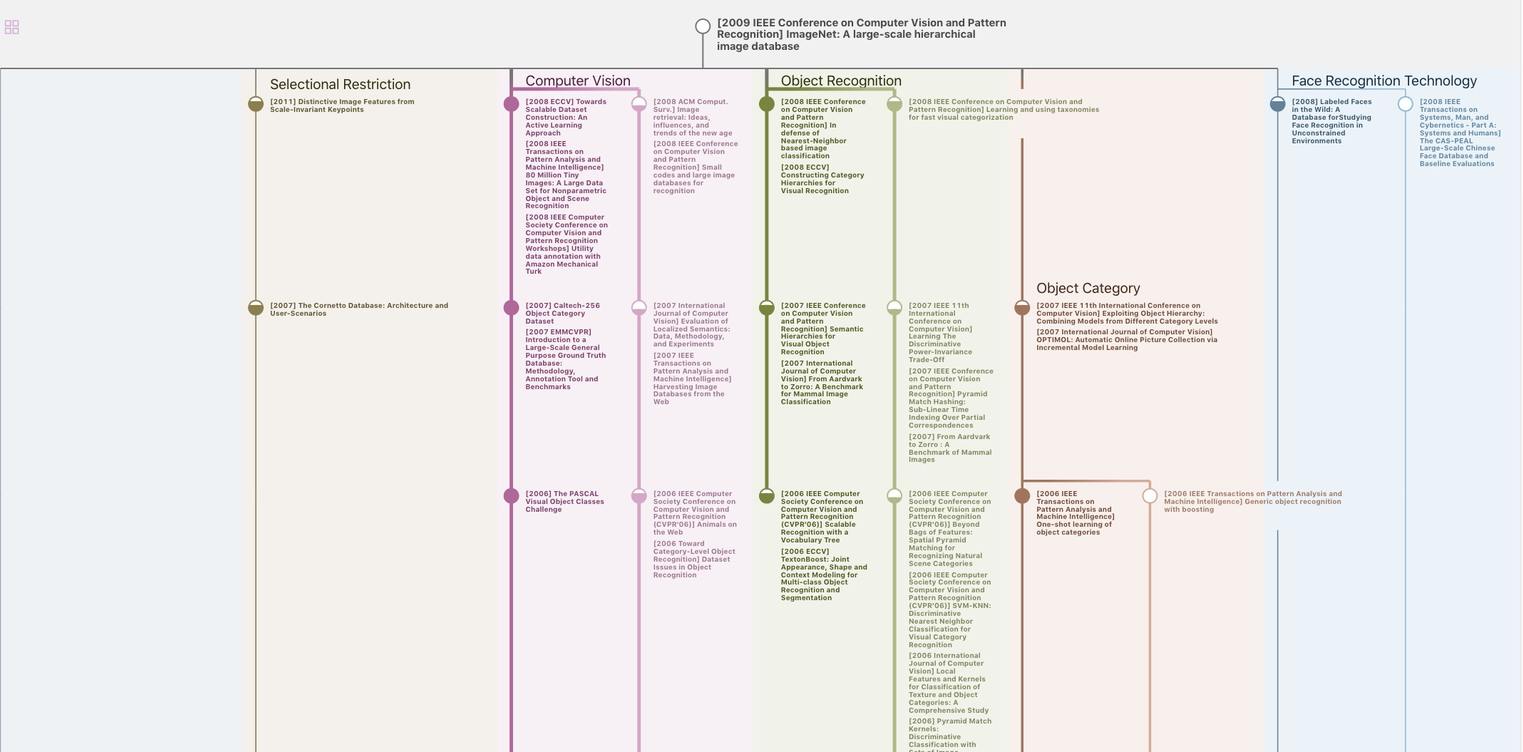
生成溯源树,研究论文发展脉络
Chat Paper
正在生成论文摘要