Leveraging Model Poisoning Attacks on License Plate Recognition Systems.
TrustCom(2022)
摘要
Computer vision-based license plate recognition (LPR) has been widely deployed for automatic vehicle identity inspection due to the offered convenience and efficiency. However, the practical LPR systems are potentially vulnerable to malicious attacks, which may lead to incorrect recognition and impact the safety of transportation. Previous studies of attacking strategies targeting LPR systems mainly focused on evasion attacks, which are less efficient than model poisoning attacks that can cause misclassification through directly manipulating the parameters of the victim model other than perturbing each testing sample. To fill this gap, we conduct the first systematic study on the vulnerability of LPR systems against model poisoning attacks. In specific, we aim to compromise the integrity of the model training such that the attacked LPR system would mis-classify all the samples from the victim class to the attacker-chosen class. To achieve this, we fine-tune the feature extractor layers of the LPR model such that it can obtain similar feature representations given samples belong to victim and attacker-chosen classes. This is implemented in a generator-discriminator fashion, where a discriminator learns to classify the victim and attacker-chosen classes given the input samples. Subsequently, the feature extractor is fine-tuned to generate manipulated features that can confuse the discriminator. Our empirical results on the CCPD dataset demonstrate that the proposed attacking strategy can substantially compromise LPR systems with high success rates.
更多查看译文
关键词
Model poisoning attack, class-targeted attack, license plate recognition
AI 理解论文
溯源树
样例
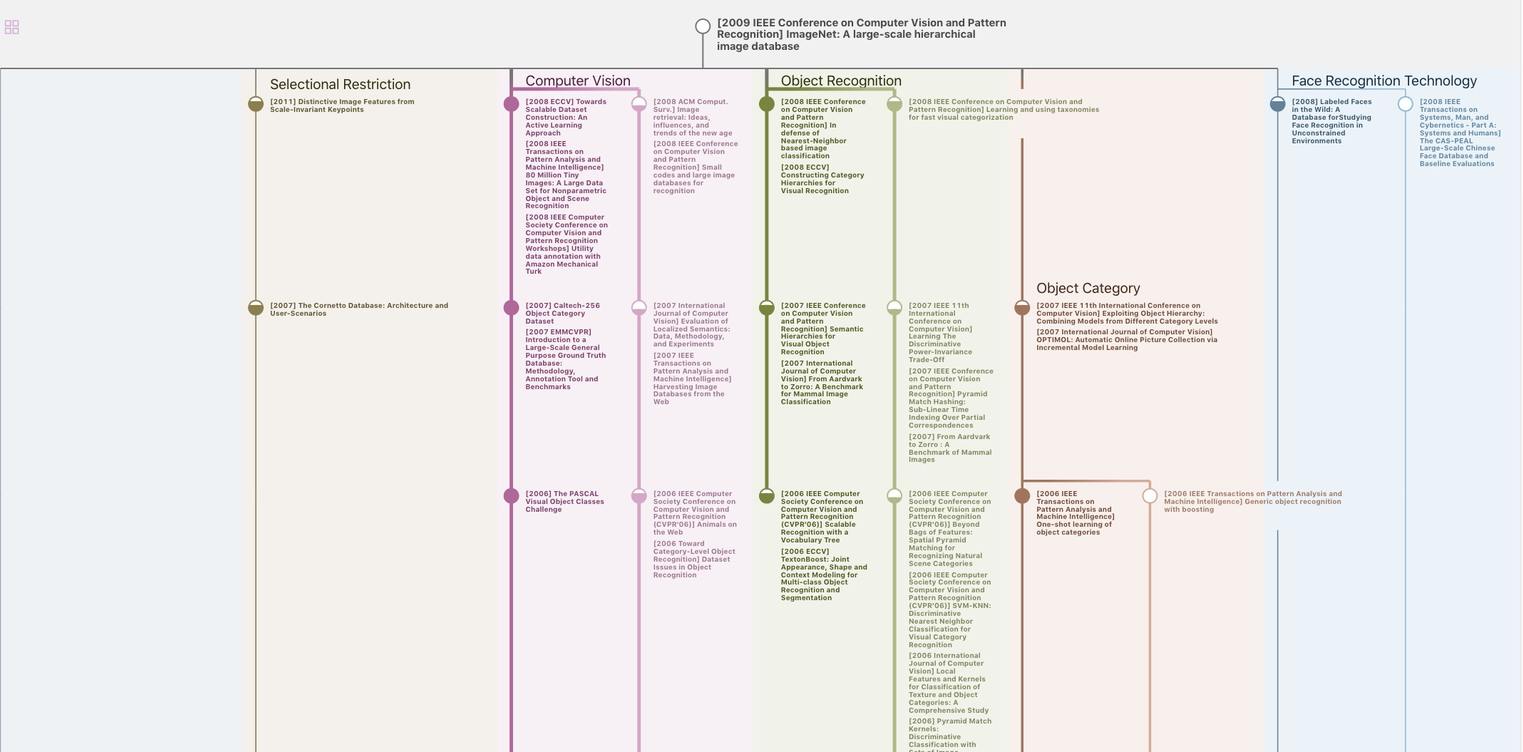
生成溯源树,研究论文发展脉络
Chat Paper
正在生成论文摘要