Effective LSTM Neural Network with Adam Optimizer for Improving Frost Prediction in Agriculture Data Stream.
MDIS(2022)
摘要
A country’s economic progress would be impossible without the agriculture sector. The primary source of income for most countries is agricultural products and related enterprises. Farming is heavily influenced by weather factors like the amount of sunlight, kind of precipitation, temperature of the air, relative humidity, and wind speed, as well as fluctuations in these factors. The temperature directly influences the metabolic reactions that take place in plants. For example, freezing damages plants to the point where they cannot grow (frost). Farmers have traditionally monitored the weather in the spring by watching television, reading the newspaper, or following a detailed weather forecast. Farm sustainability and output can be improved through smart agriculture technologies. Precision Farming (PF) can help farmers deal with various environmental issues instead of traditional agricultural approaches. It is possible to monitor farming conditions using sensors installed in the farmland area. This system requires predictive systems to increase yield. There is much interest in the field of prediction. For example, farmers can prevent crop frost by using anti-frost measures, and this research has developed a smart deep learning-based system for agricultural frost forecasting. This paper uses Long-Short Term Memory (LSTM) neural networks for the time series prediction of low temperatures. Additionally, the LSTM model is combined with an Adam optimizer to intensify the prediction model’s performance. The suggested approach is also compared with LSTM when combined with other optimizers. The findings indicate that the proposed model excels in base LSTM and LSTM with other optimizers.
更多查看译文
关键词
frost prediction,adam optimizer,effective lstm neural network,agriculture data stream
AI 理解论文
溯源树
样例
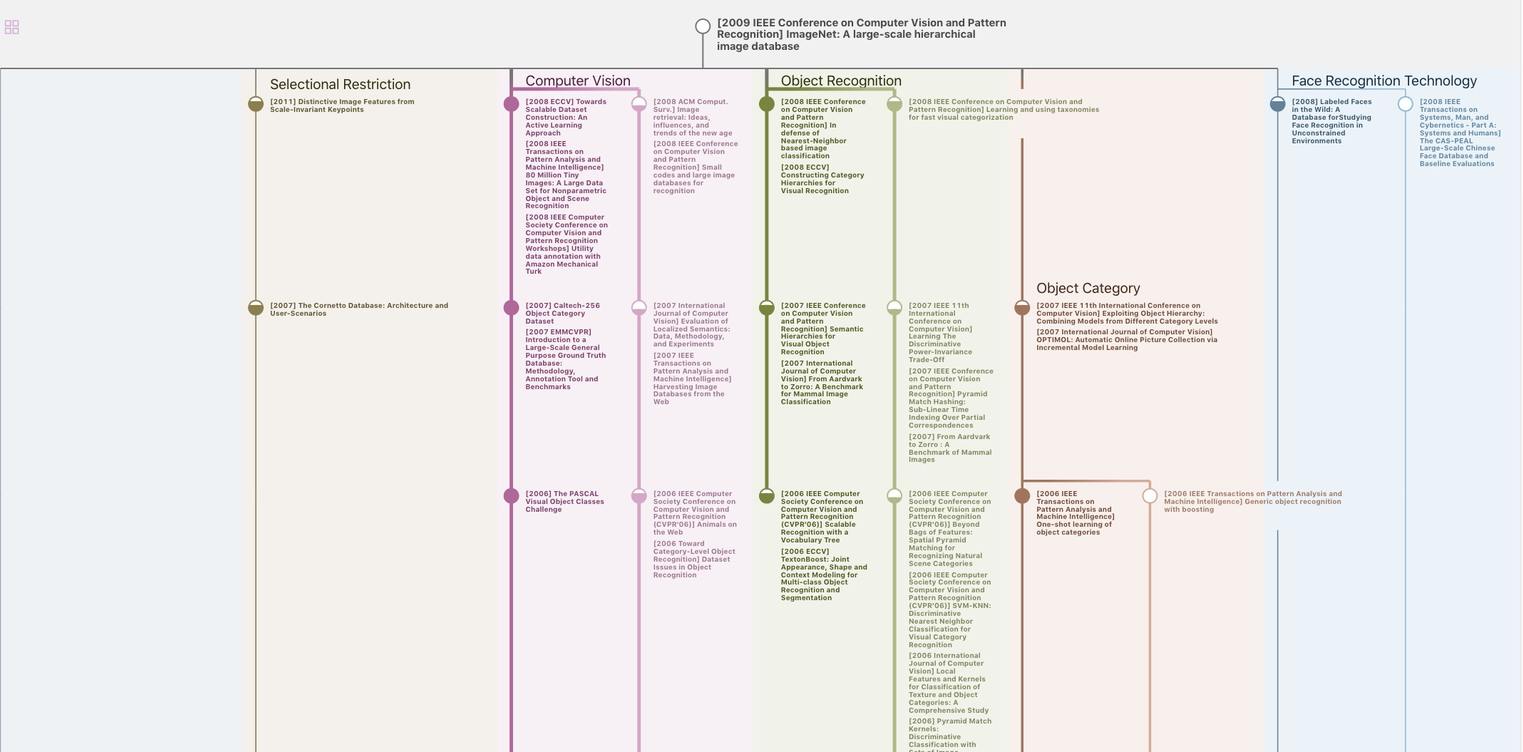
生成溯源树,研究论文发展脉络
Chat Paper
正在生成论文摘要