A 22nm 832Kb Hybrid-Domain Floating-Point SRAM In-Memory-Compute Macro with 16.2-70.2TFLOPS/W for High-Accuracy AI-Edge Devices.
ISSCC(2023)
摘要
Advanced artificial-intelligence (Al) edge devices require high energy-efficiency
$(\eta_{\mathrm{E}})$
and high inference-accuracy [2,4-6]. An SRAM-based compute-in-memory (CIM) based on MAC operations is well-suited for improving the
$\eta_{\mathrm{E}}$
of Al edge devices. However, without support for floating-point (FP) computation, Al chips using integer-based SRAM-CIMs (INT-CIM) [2,4-5] are prone to precision loss when applied to complex datasets or neural network models. Product
$(\text{PD}=\text{IN}\times \mathrm{W})$
. alignment-based FP-MACs align the product's mantissa
$(\text{PD}_{\mathrm{M}})$
prior to accumulation, based on the product's exponent
$(\text{PD}_{\mathrm{E}})$
. This approach is commonly used for digital circuits [3] and for near-memory compute [1], but is not practical for in-memory-compute (IMC) macros: each
$\text{PD}_{\mathrm{E}}$
within a physical row/column is different and thus cannot be accumulated. An INT-IMC with off-macro digital circuits and off-chip software pre-alignment was used in [6] to process the exponents of inputs
$(\text{IN}_{\mathrm{E}})$
and weights
$(\mathrm{W}_{\mathrm{E}})$
externally for the FP-MAC. An INT-CIM with extra FP-to-INT converters can emulate an FP-MAC, but incurs additional area, power consumption, and latency (PPA). Researchers have yet to develop a true FP-IMC macro capable of exponent and mantissa computation. Analog CIMs suffer from a low readout accuracy due to intrinsic transistor variation. Digital CIMs are insensitive to variation, but are limited in terms of compute parallelism due to routing congestion, as Fig. 7.1.1 shows. This paper presents a true FP-IMC macro featuring (1) a hybrid-domain macro structure that enables computation of both the exponent and mantissa in an FP-MAC within the same IMC macro. A high
$\eta_{\mathrm{E}}$
and accuracy are achieved by exploiting advantages of computing in the time, digital, and analog-voltage domain by identifying the proper functional blocks for the FP-MAC [2,4-5]. (2) Time-domain based
$\text{PD}_{\mathrm{E}}$
generation, a
$\text{maximum}-\text{PD}_{\mathrm{E}}(\text{PD}_{\mathrm{E}-\text{MAX}})$
finder (TD-MPEF), and a
$\text{PD}_{\mathrm{E}}-\text{PD}_{\mathrm{E}-\text{MAX}}$
generator
$(\text{TD}-\text{PD}_{\mathrm{E}}-\text{DG})$
to achieve a high
$\eta_{\mathrm{E}}$
for all exponent computation. (3)
$\text{PD}_{\mathrm{E}}$
-based input-mantissa alignment (PEB-IMA) scheme to enable accumulation for
$\text{PD}_{\mathrm{M}}$
in the same column. (4) A place-value dependent digital/analog-hybrid computing scheme for mantissa computation with a high inference accuracy and
$\eta_{\mathrm{E}}$
. A 22-nm 832-kb FP SRAM-IMC macro is fabricated using foundry-provided compact-6T SRAM cells. The FP SRAM-IMC support FP-MACs with 128-accumulators (ACCU) for BF16 inputs (IN) and weights (W) with FP32 outputs (OUT) and achieves the highest reported FP-MAC
$\eta_{\mathrm{E}}$
, 70.2TFLOPS/W.
更多查看译文
AI 理解论文
溯源树
样例
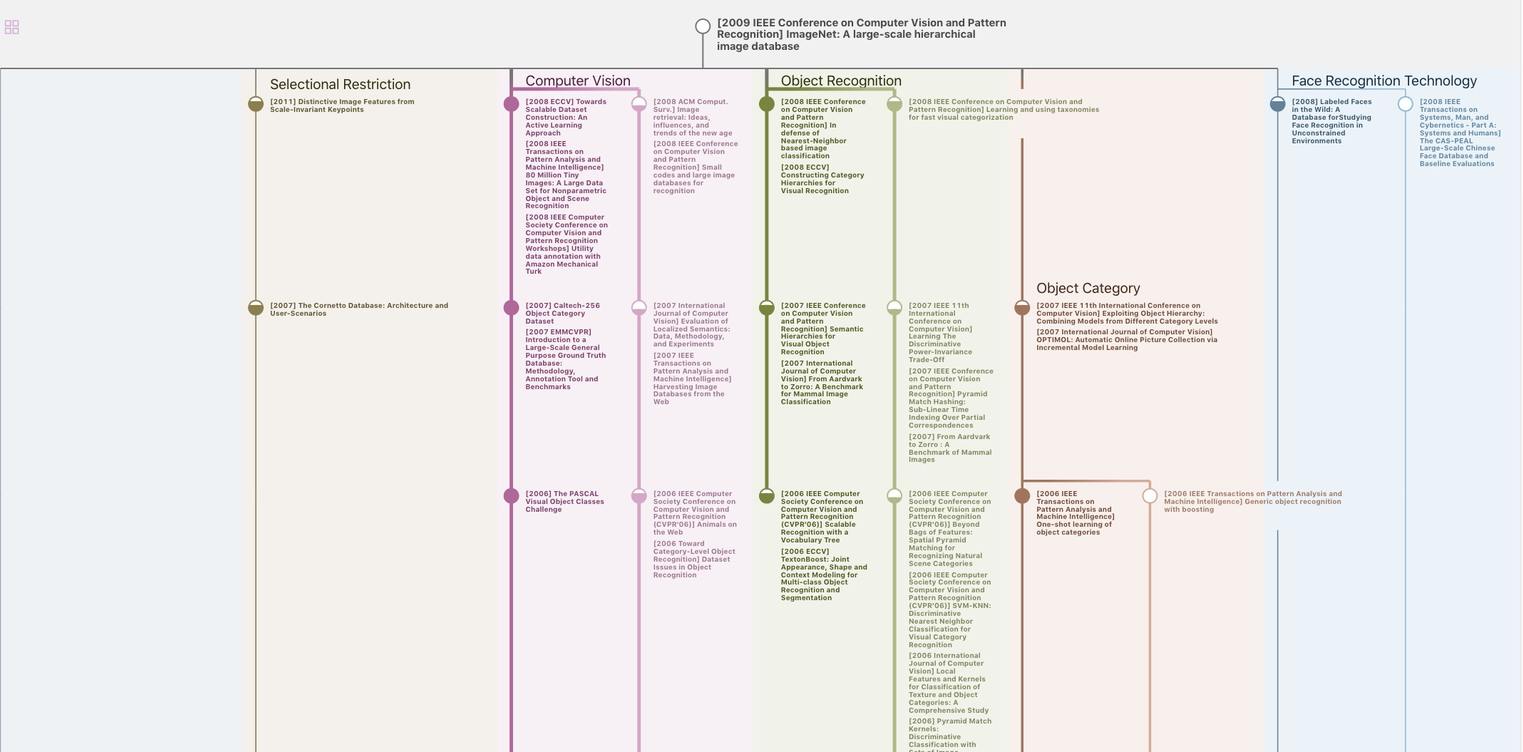
生成溯源树,研究论文发展脉络
Chat Paper
正在生成论文摘要