Projection-preserving block-diagonal low-rank representation for subspace clustering.
Neurocomputing(2023)
摘要
In this paper, a novel model named projection-preserving block-diagonal low-rank representation (PBDIR) is proposed and can obtain a more distinguishable representation matrix for clustering. PBDIR acquires a more advantageous representation by extracting the essential features. Specifically, we introduce a projection matrix to our model to learn a new feature space that can capture more significant features. Therefore, our model learns a more robust representation, which can reduce noise interference. Meanwhile, we introduce a block-diagonal regularization to ensure that the obtained representation matrix involves a k-block diagonal, where k denotes the number of clusters. This term brings more benefits for clustering tasks. Experimental results on real datasets show that our model can significantly improve the clustering performance and the proposed approach is robust against Gaussian noise, Multiplicative noise, and Salt-and-Pepper noise.
更多查看译文
关键词
Subspace clustering,Projection preserve,Block diagonal regularity,Low-rank representation
AI 理解论文
溯源树
样例
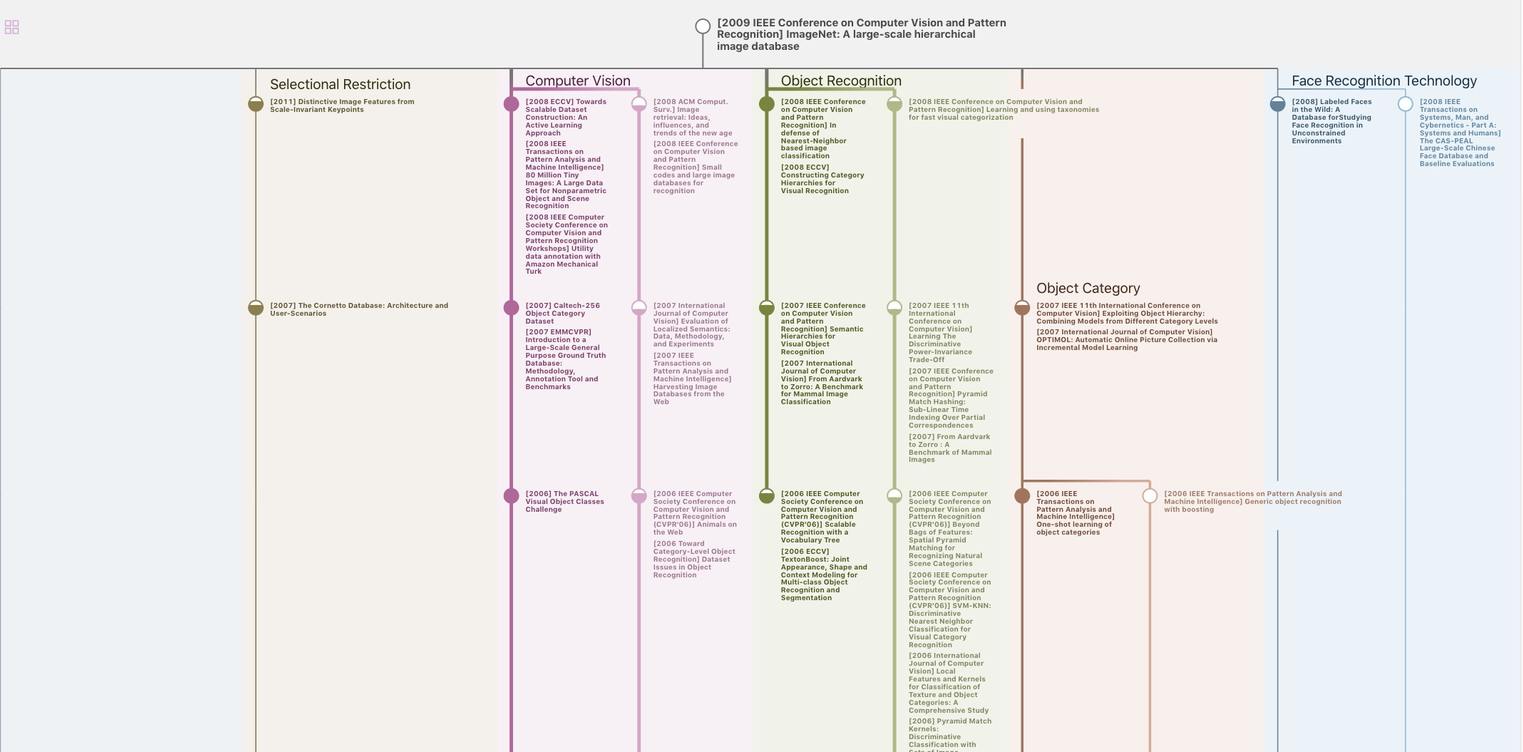
生成溯源树,研究论文发展脉络
Chat Paper
正在生成论文摘要