Probabilistic instance shape reconstruction with sparse LiDAR for monocular 3D object detection.
Neurocomputing(2023)
摘要
Monocular 3D object detection aims to localize objects in 3D space from a single image. This is a difficult problem due to the lack of accurate depth measurements. Many methods predict depths upfront using a pre-trained vision-based depth estimator to assist in 3D object detection. However, the methods show limited improved performances because of the depth inaccuracy and the neglect of depth confidence. In this paper, we propose a new end-to-end 3D object detection framework by combining a monocular camera with a cheap 4-beam LiDAR. The minimal LiDAR signal is leveraged as an additional input to predict high-quality and dense depth maps from monocular images. Meanwhile, several 3D proposals are generated by a keypoint-based detector. The key challenge is encoding the depth confidence to capture the depth estimation uncertainty. Therefore, we propose probabilistic instance shape reconstruction to exploit instance shape information for box refinement. Our method employs a fully differentiable end-to-end framework, making it simple and efficient. The experimental results on the KITTI dataset demonstrate that the proposed method achieves state-of-the-art performance, thus validating the effectiveness of the sparse LiDAR data and the probabilistic instance shape reconstruction. Code is available at https://github.com/xjtuwh/SparseLiDAR_fusion.
更多查看译文
关键词
3D object detection,Deep learning,Monocular images,Autonomous driving,Sparse LiDAR
AI 理解论文
溯源树
样例
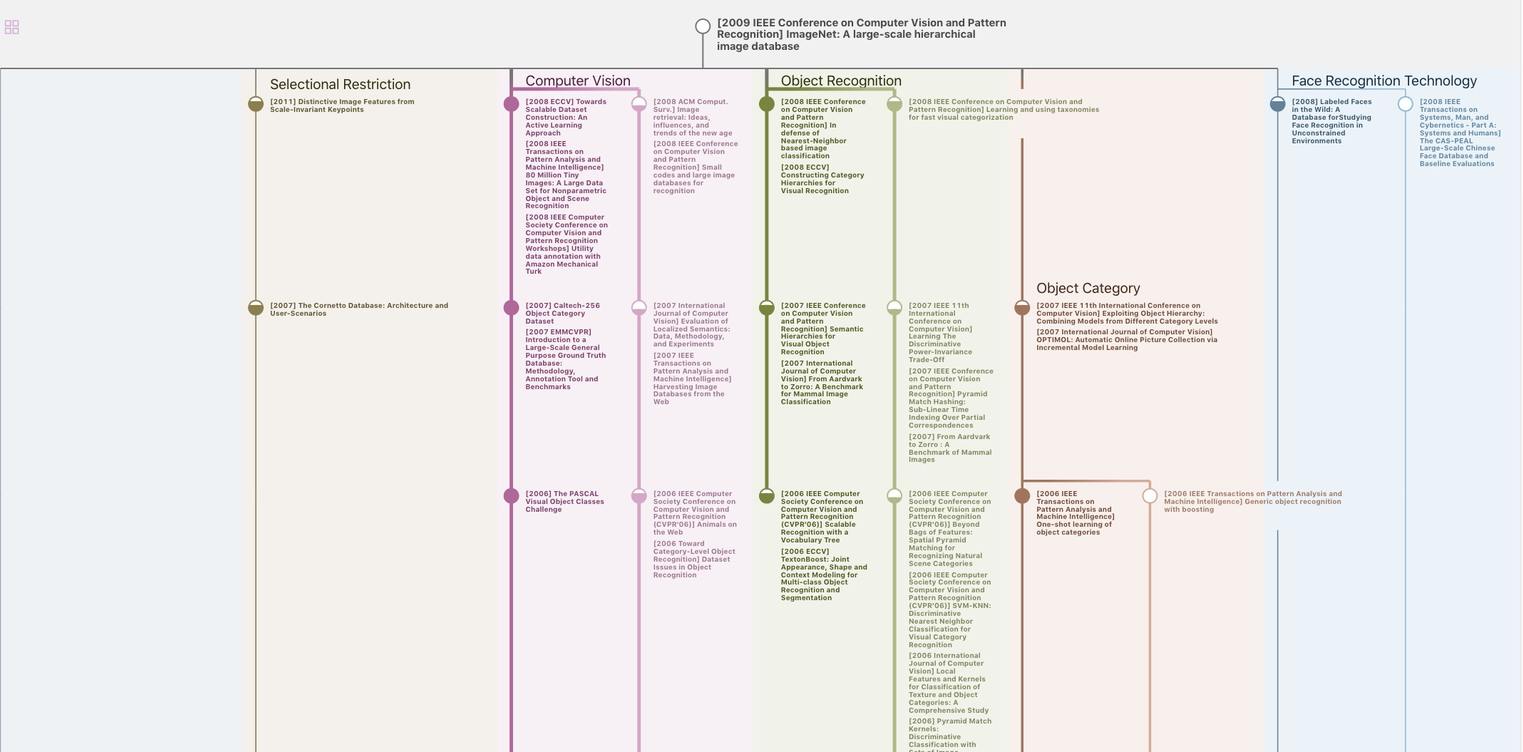
生成溯源树,研究论文发展脉络
Chat Paper
正在生成论文摘要