Improving knowledge distillation via pseudo-multi-teacher network
Mach. Vis. Appl.(2023)
摘要
Existing knowledge distillation methods usually directly push the student model to imitate the features or probabilities of the teacher model. However, the knowledge capacity of teachers limits students to learn undiscovered knowledge. To address this issue, we propose a pseudo-multi-teacher knowledge distillation method to augment the learning of undiscovered knowledge. Specifically, we propose a well-designed auxiliary classifier to capture semantic information in cross-layer that enables our network to obtain more abundant supervised information. Besides, we propose an ensemble module to combine the feature maps of each sub-network, which generates a more significant ensemble of features to guide the network. Furthermore, the auxiliary classifier and ensemble module are discarded after training, and thus there are no additional parameters introduced to the final model. Comprehensive experiments on benchmark datasets demonstrate the effectiveness of our proposed method.
更多查看译文
关键词
Convolutional neural networks,Knowledge distillation,Online distillation,Mutual learning
AI 理解论文
溯源树
样例
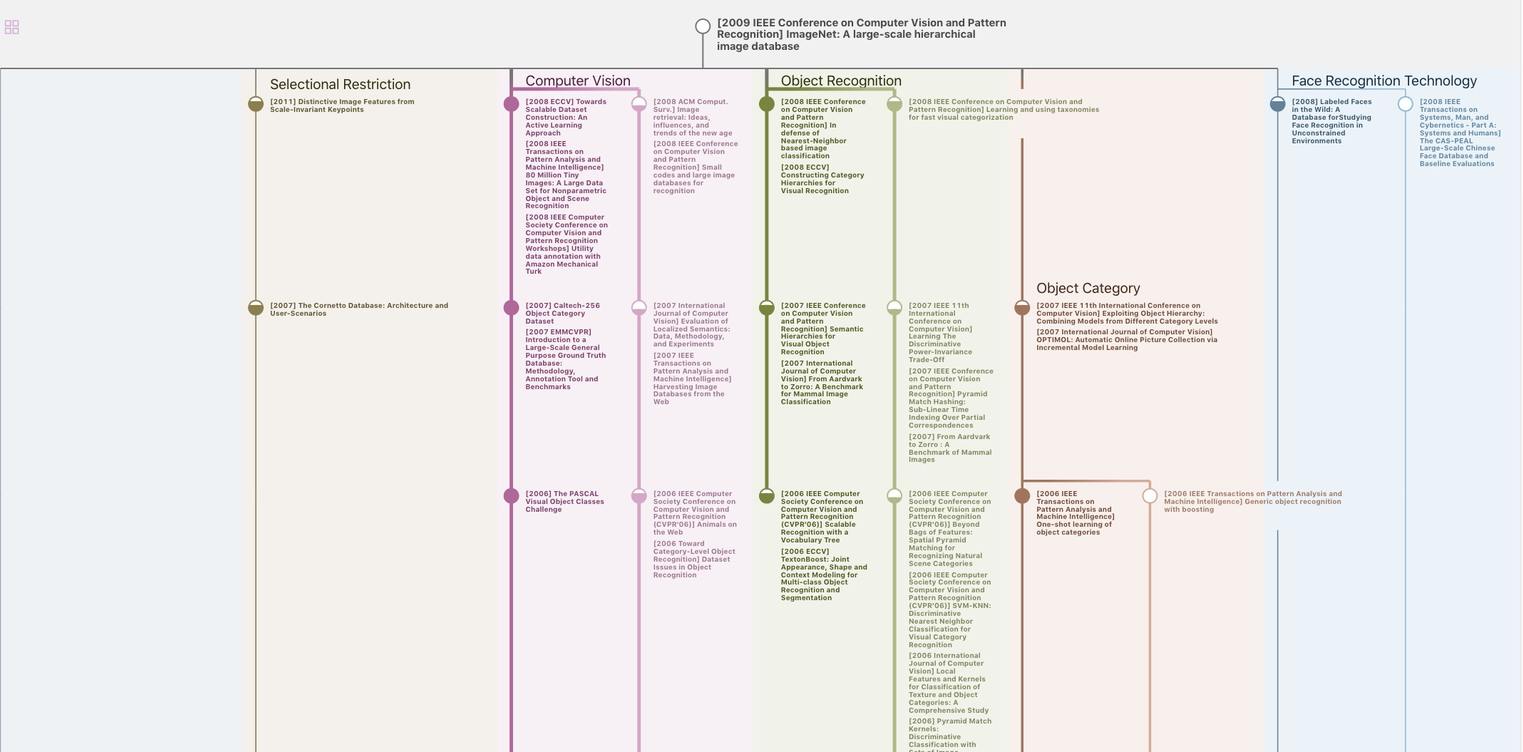
生成溯源树,研究论文发展脉络
Chat Paper
正在生成论文摘要