Data-Driven Tracking Control for Multi-Agent Systems With Unknown Dynamics via Multithreading Iterative Q-Learning.
IEEE Trans. Syst. Man Cybern. Syst.(2023)
摘要
This article addresses the tracking control problem of multiagent systems (MASs) with unknown dynamics. First, by designing a compensator, an augmented neighborhood error system is proposed. Then, a multithreading iterative
$Q$
-learning algorithm is developed, which can transform the tracking control problem into the optimal regulation of the augmented error system. In this algorithm, the agent controller is multithread, which consists of thread unit, global unit, and replay buffer. The thread unit is used to create the thread to collect the transitions with different control policies, which can reduce data relativity and improve data collection speed and exploration capability. The replay buffer is used to store the generated transitions. The global unit is used to generate the control policy. Moreover, the convergence analysis of the proposed algorithm is given, which shows that the neighborhood error converges to zero and the tracking control problem can be solved. Finally, the numerical example not only verifies the effectiveness of the obtained results but also shows the proposed algorithm performs better than the
$Q$
-iterative algorithm.
更多查看译文
关键词
Iterative Q-learning,multiagent systems (MASs),multithreading,tracking control
AI 理解论文
溯源树
样例
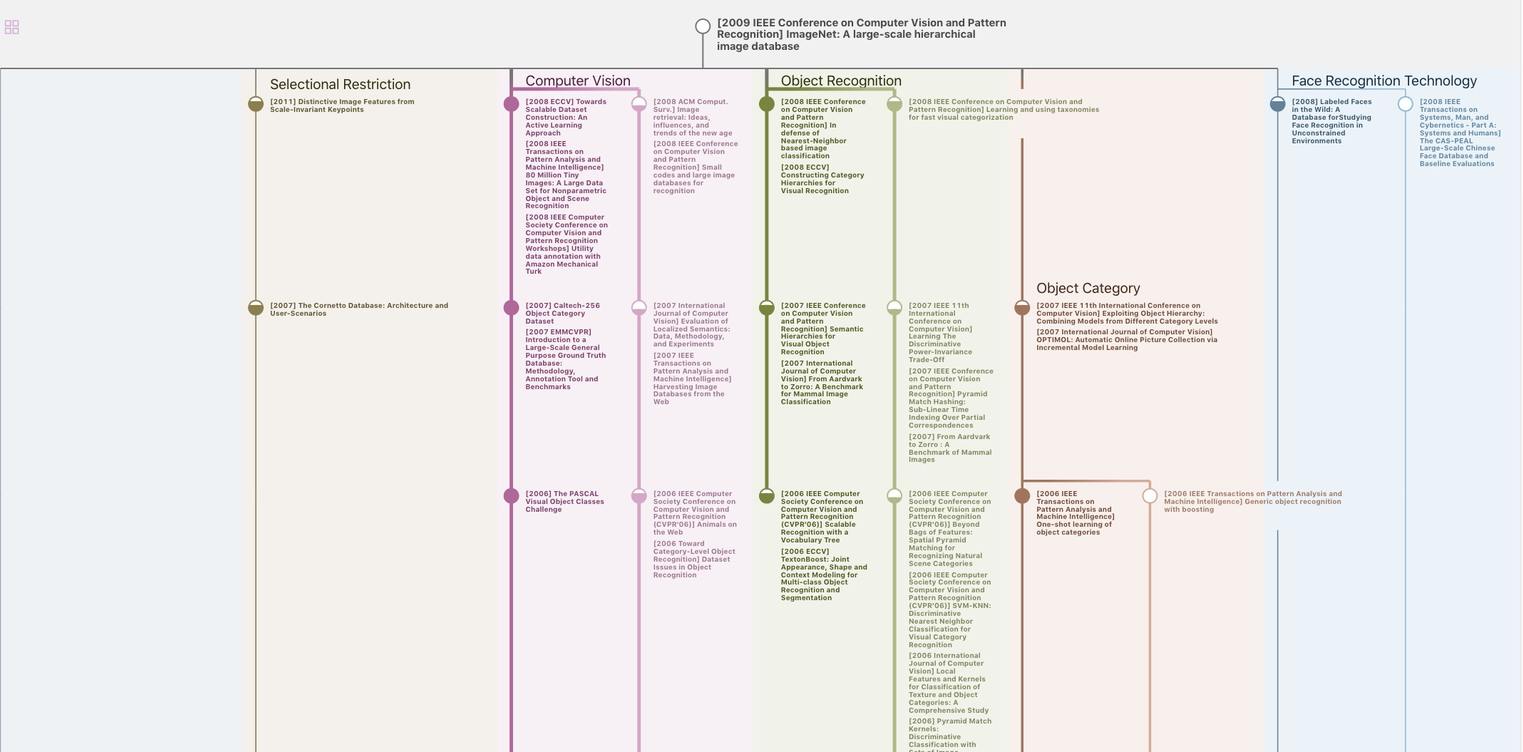
生成溯源树,研究论文发展脉络
Chat Paper
正在生成论文摘要