Attribute Enhancement using Aligned Entities between Knowledge Graphs.
ICSC(2023)
摘要
A knowledge graph (KG) is a structural form of semantic network that integrates triplets into a graph to support knowledge processing and reasoning. Popular KGs like DBpedia, YAGO, and Freebase have mostly been constructed by using automatic extraction methods on crowd-sourced content; therefore, they are not always complete or error-free. In this study, we propose an attribute enhancement framework (AEF) to enrich the attributes of entities by integrating multiple KGs based on their aligned information. Usually, similar entities from different KG contain different sets of attributes. AEF uses a representation-learning-based ranking model to determine the significant attributes. It employs a similarity mapping method to integrate new attributes into the target KG. AEF can also identify attribute value inconsistencies between two KGs. In our experimental dataset, 46% new attribute properties and 29% new values from DBpedia are proposed for the YAGO entities. In this study, we aim to include all the important attributes in the existing KGs with the goal of making a more robust and complete knowledge graph.
更多查看译文
关键词
Knowledge Graph,Attribute,Enhancement,Alignment
AI 理解论文
溯源树
样例
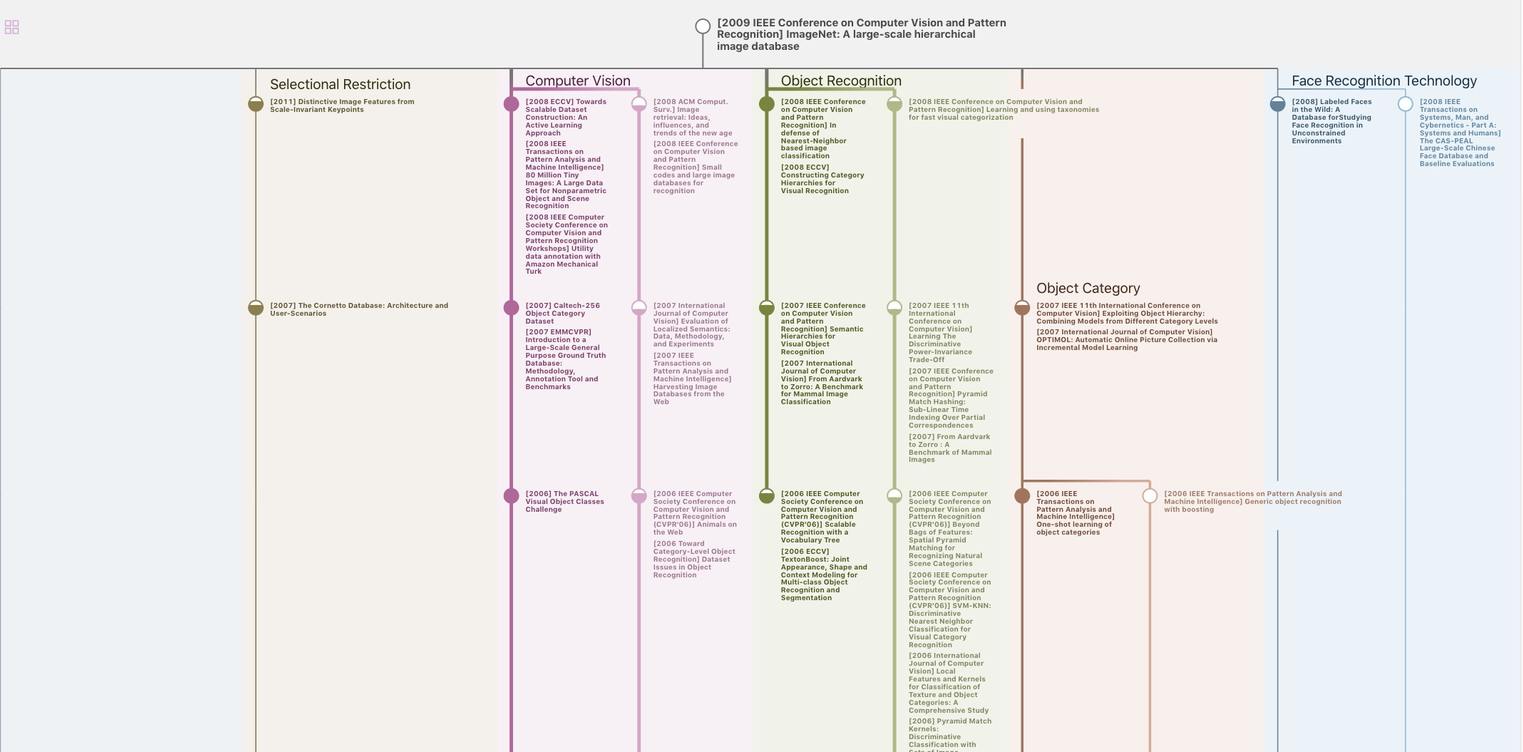
生成溯源树,研究论文发展脉络
Chat Paper
正在生成论文摘要