Cross-Domain Lossy Compression as Entropy Constrained Optimal Transport.
IEEE J. Sel. Areas Inf. Theory(2022)
摘要
We study an extension of lossy compression where the reconstruction is subject to a distribution constraint which can be different from the source distribution. We formulate our setting as a generalization of optimal transport with an entropy bottleneck to account for the rate constraint due to compression. We provide expressions for the tradeoff between compression rate and the achievable distortion with and without shared common randomness between the encoder and decoder. We study the examples of binary, uniform and Gaussian sources (in an asymptotic setting) in detail and demonstrate that shared randomness can strictly improve the tradeoff. For the case without common randomness and squared-Euclidean distortion, we show that the optimal solution partially decouples into the problem of optimal compression and transport and also characterize the penalty associated with fully decoupling them. We provide experimental results by training deep learning end-to-end compression systems for performing denoising on SVHN (The Street View House Numbers) and super-resolution on MNIST (Modified National Institute of Standards and Technology) datasets suggesting consistency with our theoretical results. Our code is available at
https://github.com/liuh127/Cross_domain_LC
.
更多查看译文
关键词
Information theory,rate-distortion theory,image compression,image restoration,optimal transport,deep learning
AI 理解论文
溯源树
样例
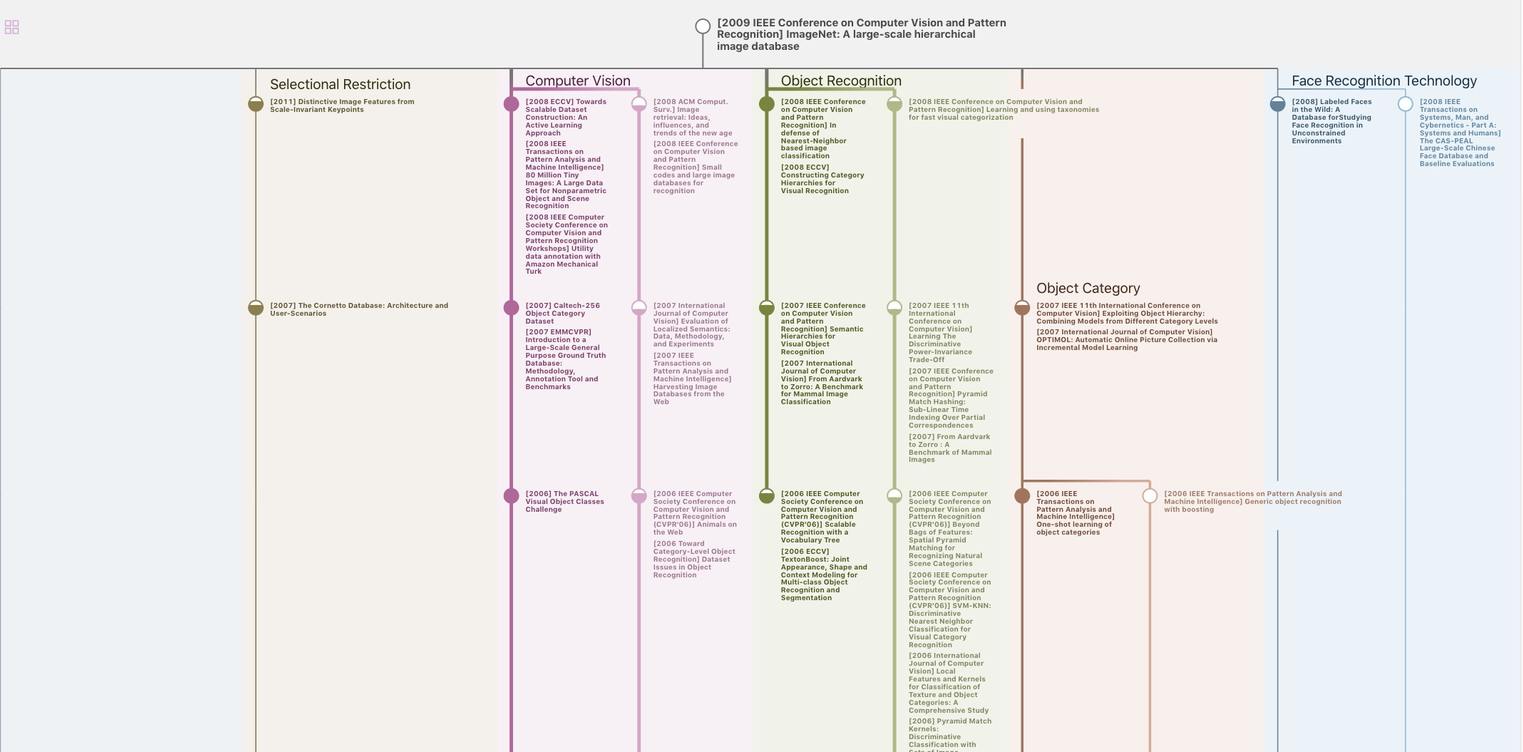
生成溯源树,研究论文发展脉络
Chat Paper
正在生成论文摘要