An in-depth study on adversarial learning-to-rank
Inf. Retr. J.(2023)
摘要
In light of recent advances in adversarial learning, there has been strong and continuing interest in exploring how to perform adversarial learning-to-rank. The previous adversarial ranking methods [e.g., IRGAN by Wang et al. (IRGAN: a minimax game for unifying generative and discriminative information retrieval models. Proceedings of the 40th SIGIR pp. 515–524, 2017)] mainly follow the generative adversarial networks (GAN) framework (Goodfellow et al. in Generative adversarial nets. Proceedings of NeurIPS pp. 2672–2680, 2014), and focus on either pointwise or pairwise optimization based on the rule-based adversarial sampling. Unfortunately, there are still many open problems. For example, how to perform listwise adversarial learning-to-rank has not been explored. Furthermore, GAN has many variants, such as f-GAN (Nowozin et al. in Proceedings of the 30th international conference on neural information processing systems, pp. 271–279, 2016) and EBGAN (Zhao et al. in Energy-based generative adversarial network. International conference on learning representations (ICLR), 2017), a natural question arises then: to what extent does the adversarial learning strategy affect the ranking performance? To cope with these problems, firstly, we show how to perform adversarial learning-to-rank in a listwise manner by following the GAN framework. Secondly, we investigate the effects of using a different adversarial learning framework, namely f-GAN. Specifically, a new general adversarial learning-to-rank framework via variational divergence minimization is proposed (referred to as IRf-GAN). Furthermore, we show how to perform pointwise, pairwise and listwise adversarial learning-to-rank within the same framework of IRf-GAN. In order to clearly understand the pros and cons of adversarial learning-to-rank, we conduct a series of experiments using multiple benchmark collections. The experimental results demonstrate that: (1) Thanks to the flexibility of being able to use different divergence functions, IRf-GAN-pair shows significantly better performance than adversarial learning-to-rank methods based on the IRGAN framework. This reveals that the learning strategy significantly affects the adversarial ranking performance. (2) An in-depth comparison with conventional ranking methods shows that although the adversarial learning-to-rank models can achieve comparable performance as conventional methods based on neural networks, they are still inferior to LambdaMART by a large margin. In particular, we pinpoint that the weakness of adversarial learning-to-rank is largely attributable to the gradient estimation based on sampled rankings which significantly diverge from ideal rankings. Careful examination of this weakness is highly recommended for developing adversarial learning-to-rank approaches.
更多查看译文
关键词
Learning-to-rank,Adversarial optimization,Variational divergence minimization,Reparameterization
AI 理解论文
溯源树
样例
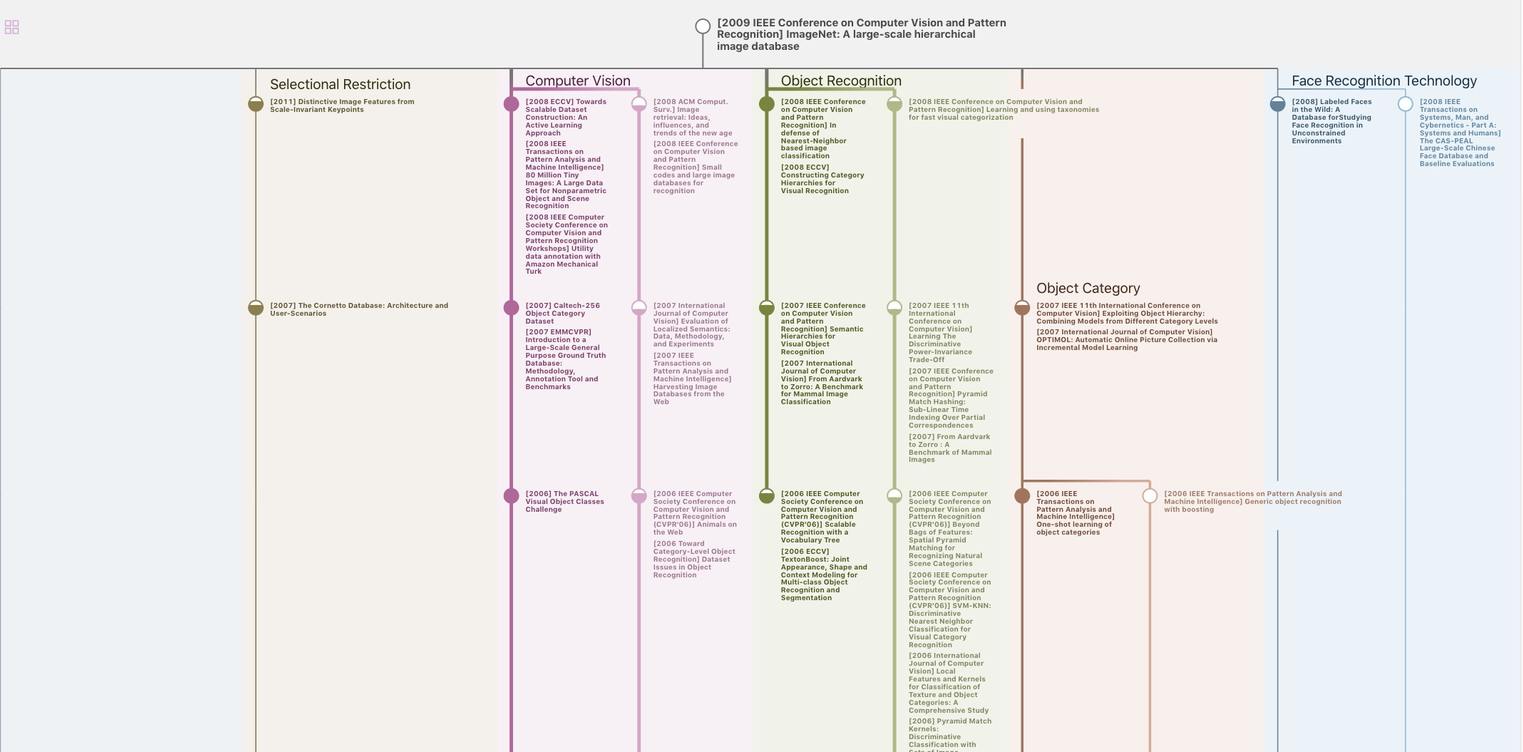
生成溯源树,研究论文发展脉络
Chat Paper
正在生成论文摘要