The Hysteresis Effect of Momentum Spillover in Asset Pricing via Spatial-Temporal Graph Learning.
ICNC(2023)
摘要
Asset pricing is a long-standing topic in finance. To capture the momentum spillovers of listed firms, recent pilot studies have been studying graph neural networks to lead the influence propagation among listed firms and predict stock movement. However, all these works ignored the hysteresis effect of momentum spillover where the future return of a target firm lags behind the historical momentum spillovers which would cause hysteresis influence. In this study, we argue that the hysteresis effect of momentum spillover is critical in asset pricing and propose a novel hysteresis spatial-temporal graph learning (HysGL) for it. For spatial learning, we dynamically estimate the momentum spillover generated at each moment to represent the influence propagated from changing relevant firms. For temporal learning, we progressively propose the time-sensitive and state-sensitive mechanisms to explicitly model the influence pattern of hysteresis effect by calculating the response strength of a target firm to all historical momentum spillovers over the time dimension, based on which the cumulative hysteresis influence received by the target firm is obtained and then sequentially embedded to extract the hidden state preserving the temporal dependency of momentum spillovers. Extensive experiments performed on two real-world financial datasets demonstrate the superiority of the HysGL over the state-of-the-art algorithms, including TGC, FinGAT and AD-GAT.
更多查看译文
关键词
Asset Pricing,Stock Prediction,Graph Neural Network,Spatial-Temporal Learning.
AI 理解论文
溯源树
样例
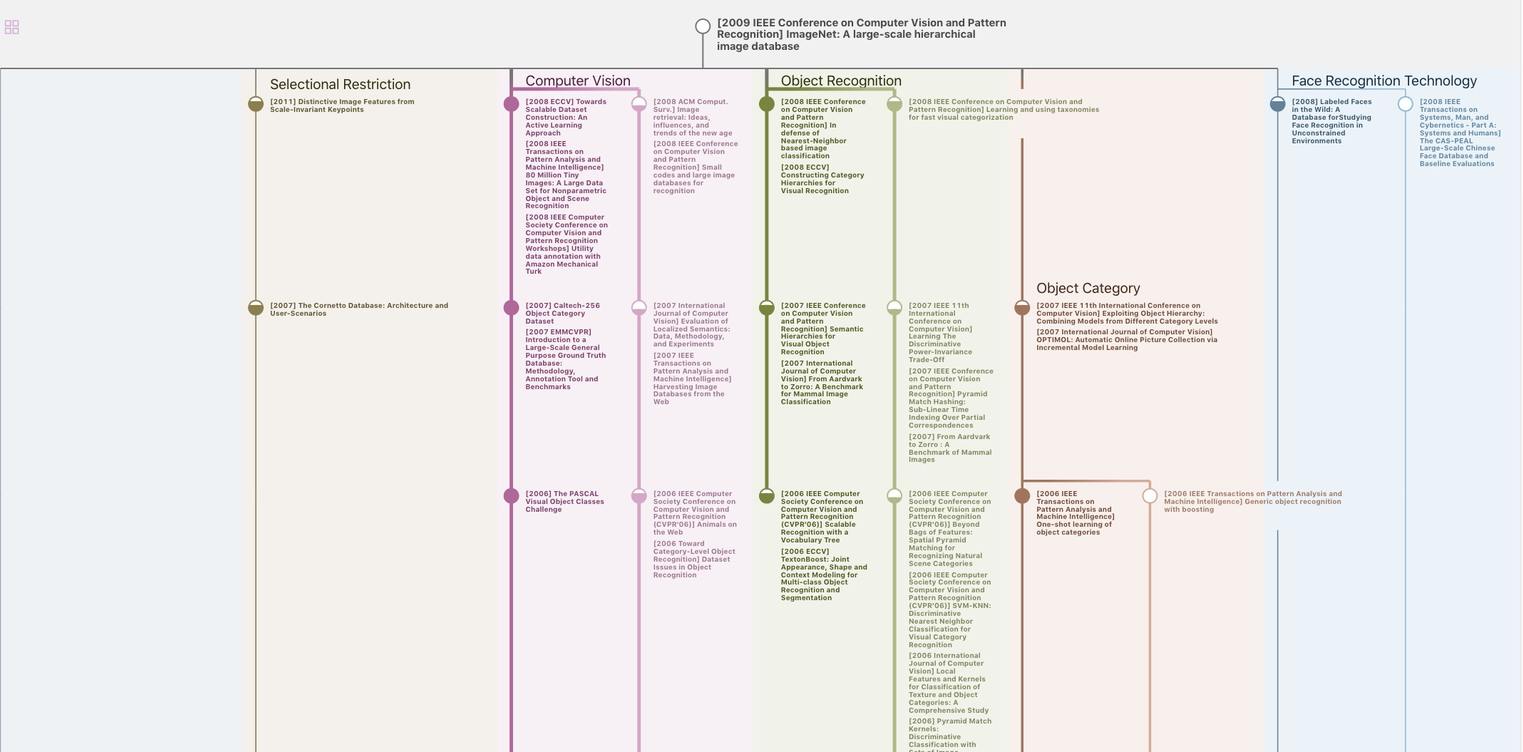
生成溯源树,研究论文发展脉络
Chat Paper
正在生成论文摘要