Justifying Multi-label Text Classifications for Healthcare Applications.
ECIR (2)(2023)
摘要
The healthcare domain is a very active area of research for Natural Language Processing (NLP). The classification ofmedical records according to codes from the International Classification of Diseases (ICD) is an essential task in healthcare. As a very sensitive application, the automatic classification of personal medical records cannot be immediately trusted without human approval. As such, it is desirable for classification models to provide reasons for each decision, such that the medical coder can validatemodel predictions without reading the entire document. AttentionXML is a multi-label classification model that has shown high applicability for this task and can provide attention distributions for each predicted label. In practice, we have found that these distributions do not always provide relevant spans of text. We propose a simple yet effective modification to AttentionXML for finding spans of text that can better aid the medical coders: splitting the BiLSTM of AttentionXML into a forward and a backward LSTM, creating two attention distributions that find the leftmost and rightmost limits of the text spans. We also propose a novel metric for the usefulness of ourmodel's suggestions by computing the drop in confidence from masking out the selected text spans. We show that our model has a similar classification performance toAttentionXML while surpassing it in obtaining relevant text spans.
更多查看译文
关键词
Healthcare, Multi-label classification, Span extraction
AI 理解论文
溯源树
样例
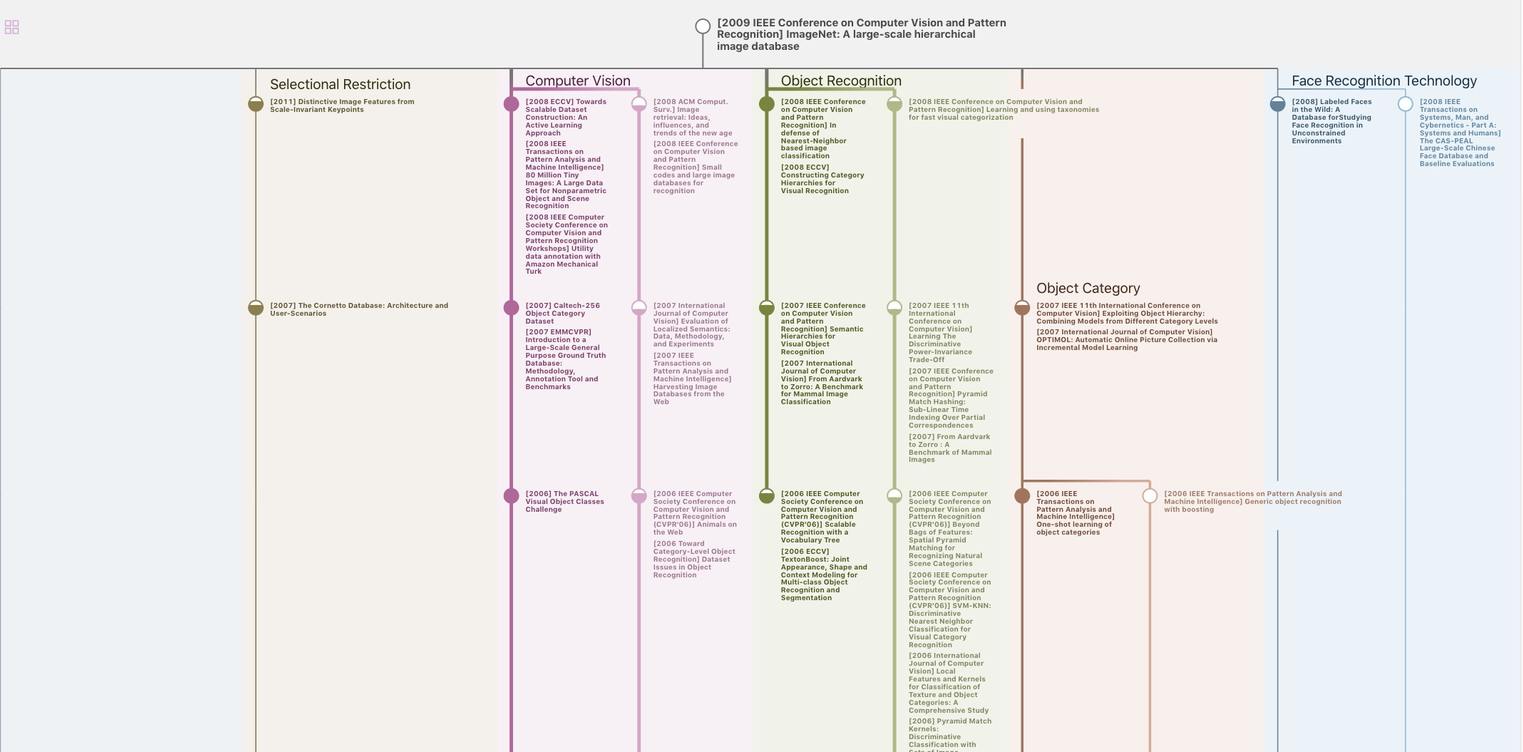
生成溯源树,研究论文发展脉络
Chat Paper
正在生成论文摘要