Augmenting Graph Convolutional Networks with Textual Data for Recommendations.
ECIR (2)(2023)
摘要
Graph Convolutional Networks have recently shown state-of-the-art performance for collaborative filtering-based recommender systems. However, many systems use a pure user-item bipartite interaction graph, ignoring available additional information about the items and users. This paper proposes an effective and general method, TextGCN, that utilizes rich textual information about the graph nodes, specifically user reviews and item descriptions, using pre-trained text embeddings. We integrate those reviews and descriptions into item recommendations to augment graph embeddings obtained using LightGCN, a SOTA graph network. Our model achieves a 7–23% statistically significant improvement over this SOTA baseline when evaluated on several diverse large-scale review datasets. Furthermore, our method captures semantic signals from the text, which are not available when using graph connections alone.
更多查看译文
关键词
Graph Convolutional Networks, Product recommendations, Textual augmentation
AI 理解论文
溯源树
样例
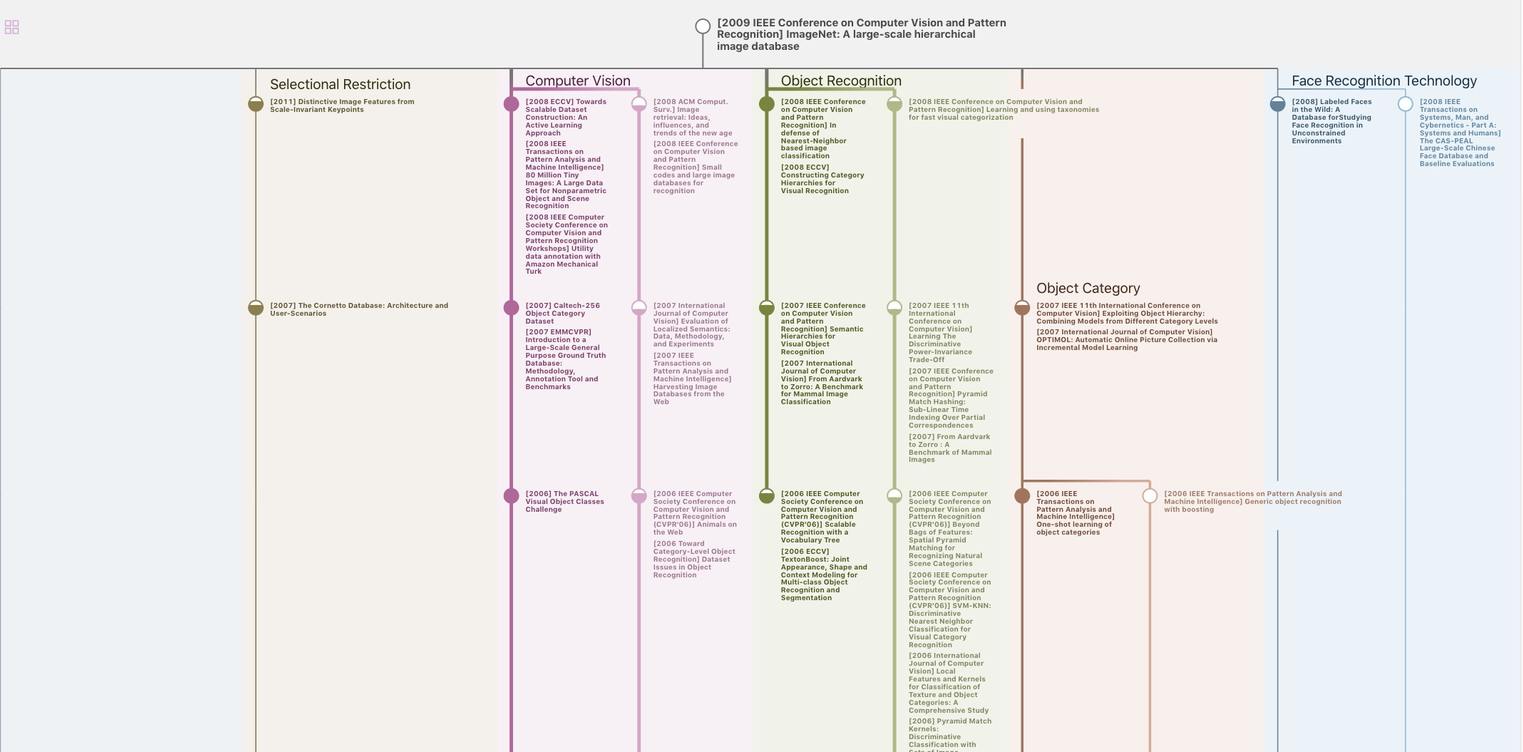
生成溯源树,研究论文发展脉络
Chat Paper
正在生成论文摘要