Same-day delivery with fair customer service.
Eur. J. Oper. Res.(2023)
摘要
The demand for same-day delivery (SDD) has increased rapidly in the last few years and has particu-larly boomed during the COVID-19 pandemic. The fast growth is not without its challenge. In 2016, due to low concentrations of memberships and far distance from the depot, certain minority neighborhoods were excluded from receiving Amazon's SDD service, raising concerns about fairness. In this paper, we study the problem of offering fair SDD service to customers. The service area is partitioned into differ-ent regions. Over the course of a day, customers request for SDD service, and the timing of requests and delivery locations are not known in advance. The dispatcher dynamically assigns vehicles to make de-liveries to accepted customers before their delivery deadline. In addition to overall service rate ( utility ), we maximize the minimal regional service rate across all regions ( fairness ). We model the problem as a multi-objective Markov decision process and develop a deep Q-learning solution approach. We introduce a novel transformation of learning from rates to actual services, which creates a stable and efficient learn-ing process. Computational results demonstrate the effectiveness of our approach in alleviating unfairness both spatially and temporally in different customer geographies. We show this effectiveness is valid with different depot locations, providing businesses with an opportunity to achieve better fairness from any location. We also show that the proposed approach performs efficiently when serving heterogeneously wealthy districts in the city.(c) 2022 Elsevier B.V. All rights reserved.
更多查看译文
关键词
Transportation,Same -day delivery,Fairness,Reinforcement learning
AI 理解论文
溯源树
样例
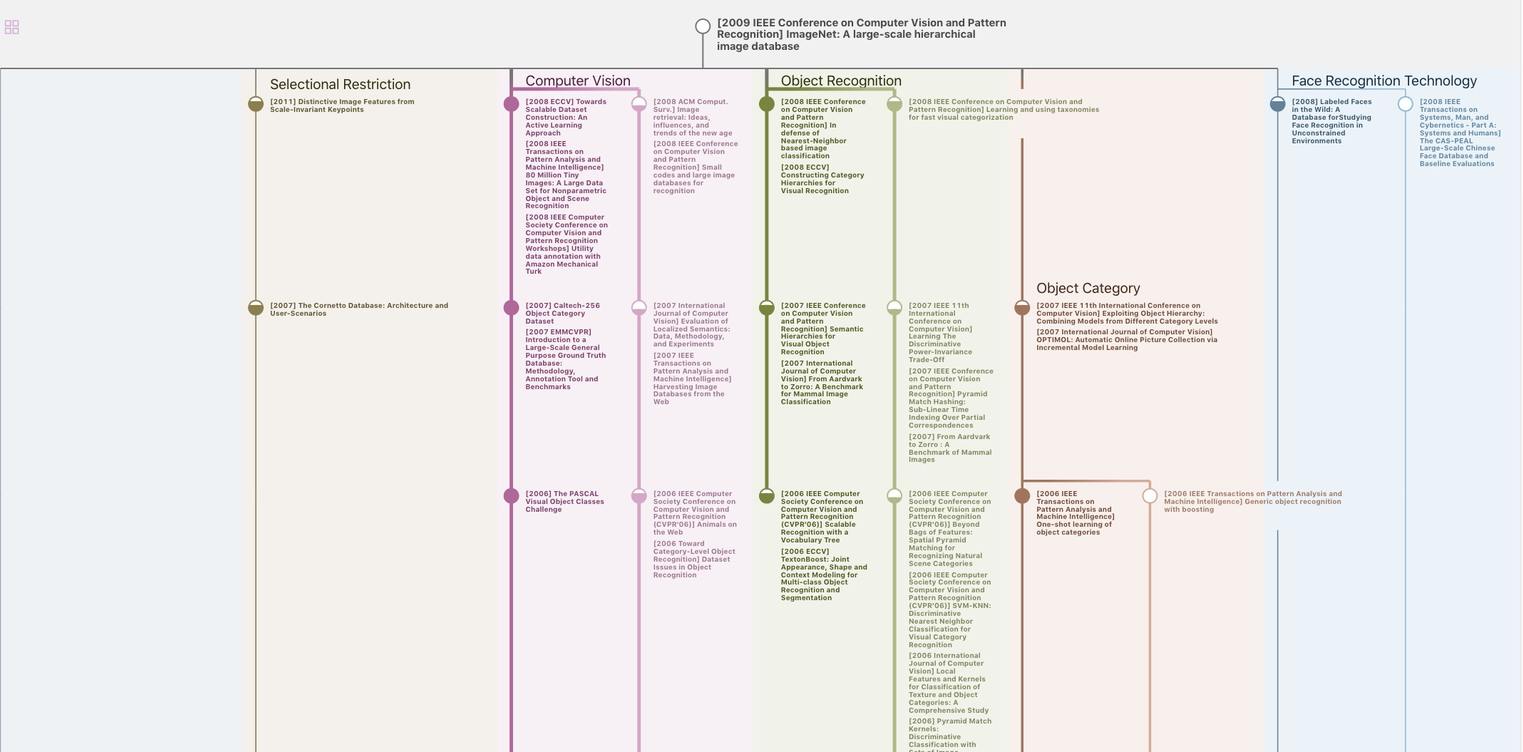
生成溯源树,研究论文发展脉络
Chat Paper
正在生成论文摘要