Integrating Machine Learning and Model Predictive Control for automotive applications: A review and future directions.
Eng. Appl. Artif. Intell.(2023)
摘要
In this review paper, the integration of Machine Learning (ML) and Model Predictive Control (MPC) in Automotive Control System (ACS) applications are discussed. ACS can be divided into these three main subsystems: enhancing safety, improving comfort, and reducing fuel consumption and emissions. Due to the development of new technologies such as advancing autonomous and connected vehicles the complexity of these subsystems is increasing. The ACS is meant to encompass the vehicle dynamics, powertrain control, passenger comfort, and accessories. Since vehicle manufacturers must meet stringent performance and emission requirements, optimal control methods for ACS applications are seen as a promising technology. MPC is an optimal control method for closed-loop control applications that allows constraints to be enforced in real-time while an objective function is minimized. The application of MPC in the automotive industry has been shown in the past decade. An important challenge in the design and real-time implementation of MPC is having a accurate predictive model that also does not require excessive real-time computation. Using ML to provide an accurate model at decreased computational cost improves MPC performance of ACS and is the main focus of this paper. How MPC in ML-based ACS applications ensures stability while meeting constraint is also discussed. Method to combine MPC and ML for the ACS subsystems of vehicle dynamics and powertrain control are reviewed and an outlook on future ACS is discussed.
更多查看译文
关键词
Machine Learning,Model Predictive Control,Automotive Control Systems
AI 理解论文
溯源树
样例
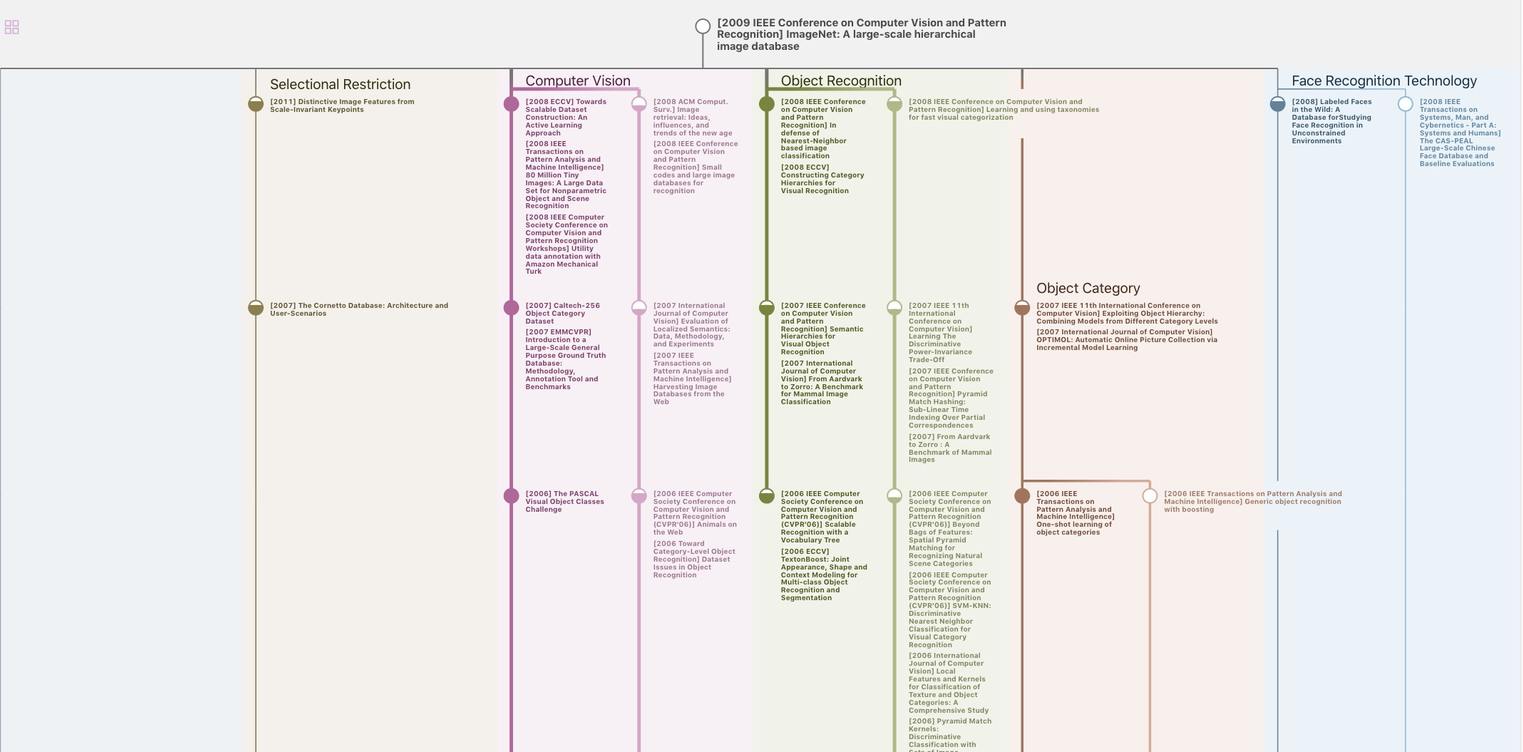
生成溯源树,研究论文发展脉络
Chat Paper
正在生成论文摘要