Automatic Detection of Individual Trees in Forests Based on Airborne LiDAR Data with a Tree Region-Based Convolutional Neural Network (RCNN).
Remote. Sens.(2023)
摘要
Light Detection and Ranging (LiDAR) has advantages in detecting individual trees because it can obtain information on the vertical structure and even on the lower layers. However, the current methods still cannot detect understory well, because the small trees are often clumped together and overlapped by large trees. To fill this gap, a two-stage network named Tree Region-Based Convolutional Neural Network (RCNN) was proposed to directly detect trees from point clouds. In the first stage, very dense anchors are generated anywhere in a forest. Then, Tree RCNN can directly focus on determining whether an anchor belongs to an individual tree or not and generate tree proposals based on the anchors. In this way, the small trees overlapped by big trees can be detected in the process. In the second stage, multi-position feature extraction is proposed to extract shape features of the tree proposals output in the first stage to refine the tree proposals. The positions and heights of detected trees can be obtained by the refined tree proposals. The performance of our method was estimated by a public dataset. Compared to methods provided by the dataset and the commonly used deep learning methods, Tree RCNN achieved the best performance, especially for the lower-layer trees. The root mean square value of detection rates (RMSass) of all plots of the dataset reached 61%, which was 6 percentage points higher than the best RMSass of other methods. The RMSass of the layers < 5 m, 5-10 m, 10-15 m, and 15-20 reached 20%, 38%, 48%, and 61%, which was 5, 6, 7, and 3 percentage points higher than the best RMSass of other methods, respectively. The results indicated our method can be a useful tool for tree detection.
更多查看译文
关键词
individual trees,forests,Region-Based Convolutional Neural Network,anchors,multi-position feature extraction
AI 理解论文
溯源树
样例
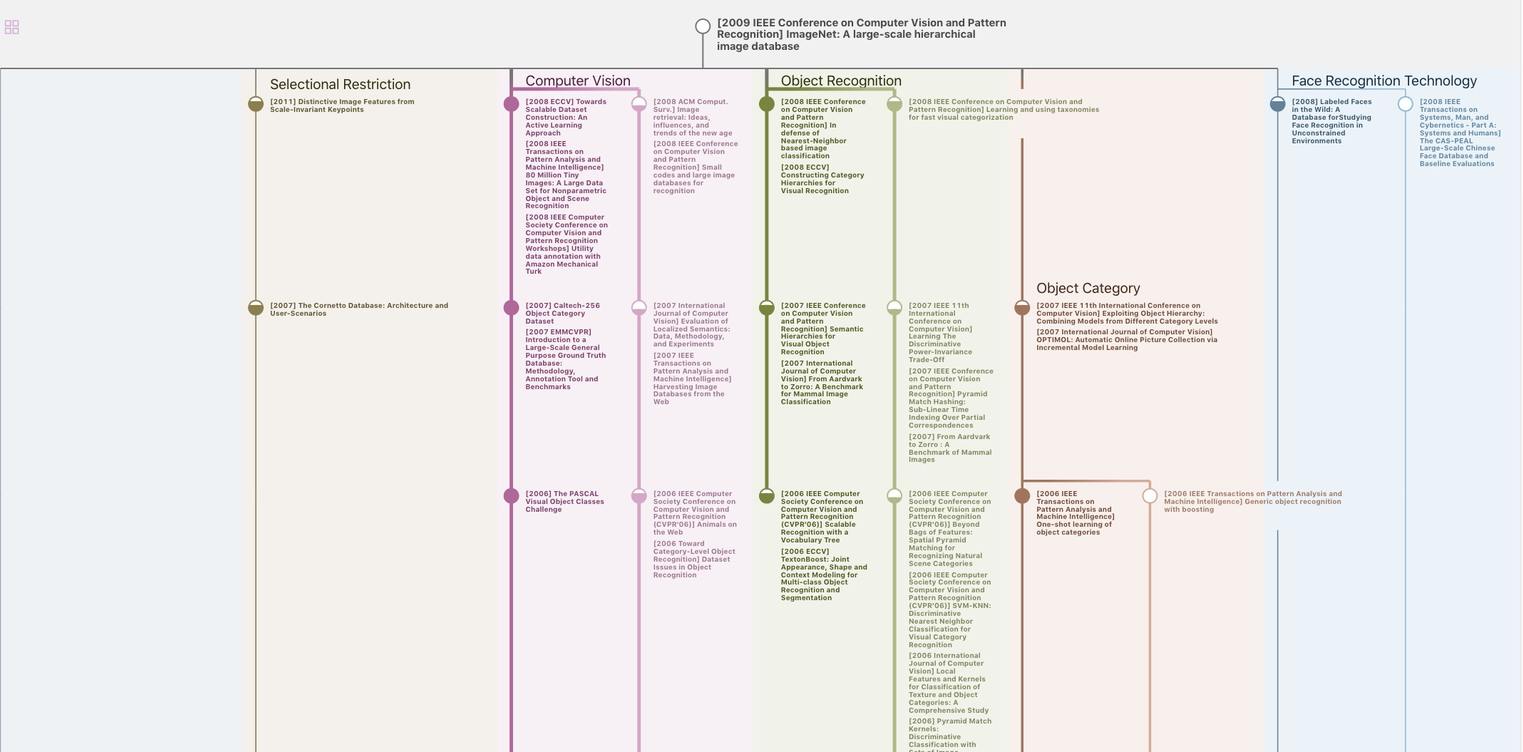
生成溯源树,研究论文发展脉络
Chat Paper
正在生成论文摘要