Basket Booster for Prototype-based Contrastive Learning in Next Basket Recommendation.
ECML/PKDD (1)(2022)
摘要
Next basket recommendation seeks to model the correlation of items and mine users' interests hidden in basket sequences, and tries to infer a set of items that tend to be adopted in the next session with the mined information. However, the feedback provided by users often involves only a small fraction of millions to billions of items. Sparse data makes it hard for model to infer high-quality representations for basket sequences, which further leads to poor recommendation. Inspired by the recent success of representation learning in some fields, e.g., computer vision and clustering, we propose a basket booster for prototype-based contrastive learning (BPCL) in next basket recommendation. A correlative basket booster is designed to mine self-supervised signals just from raw data and make augmentation for baskets. To our best knowledge, this is the first work to promote learning of prototype representation through basket augmentation, which helps overcome the difficulties caused by data sparsity and leads to a better next basket recommendation performance. Extensive experiments on three public real-world datasets demonstrate that the proposed BPCL method achieves better performance than the existing state-of-the-art methods.
更多查看译文
关键词
basket booster,contrastive learning,prototype-based
AI 理解论文
溯源树
样例
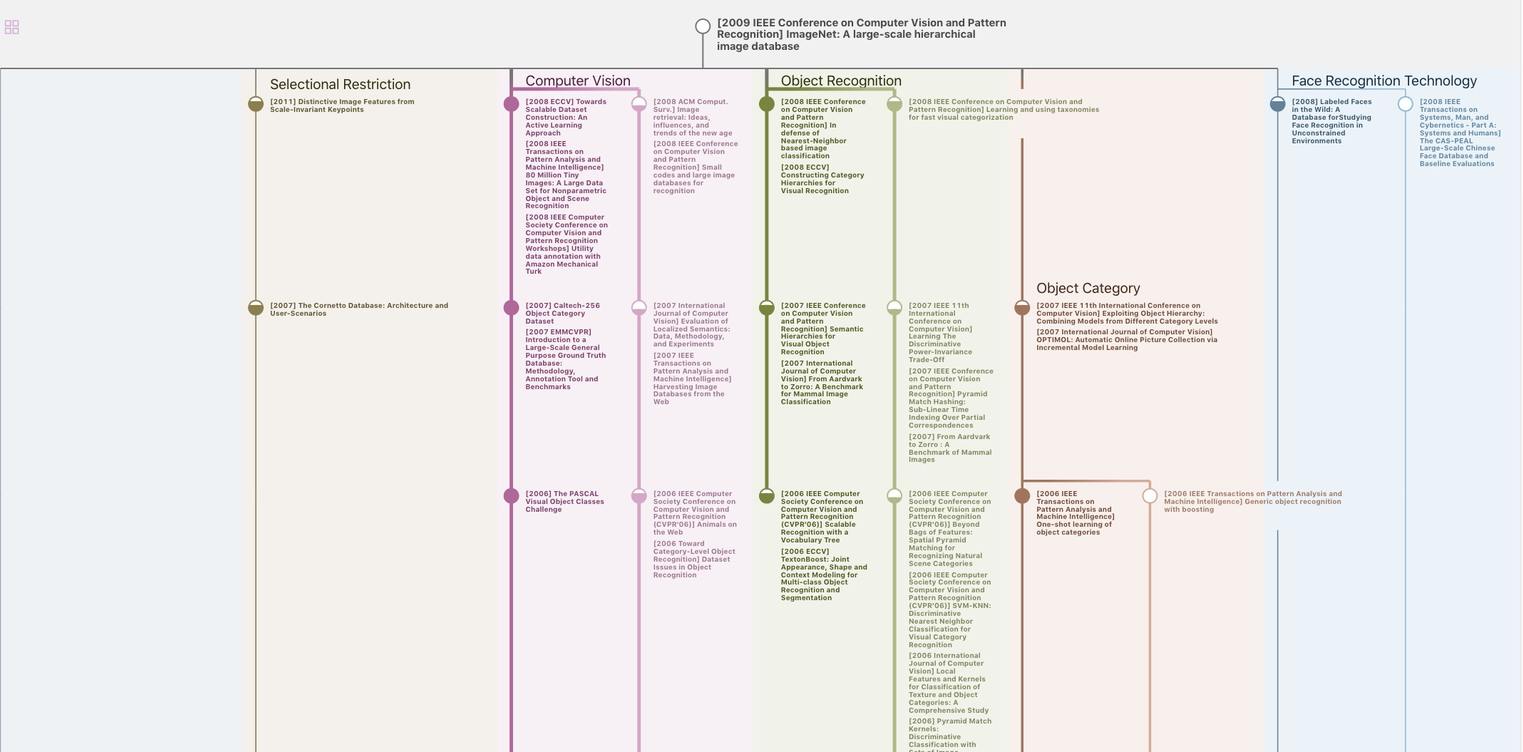
生成溯源树,研究论文发展脉络
Chat Paper
正在生成论文摘要