Nearly-lossless-to-lossy medical image compression by the optimized JPEGXT and JPEG algorithms through the anatomical regions of interest.
Biomed. Signal Process. Control.(2023)
摘要
Currently, plenty of image data is generated that complicate storage and image transmission. Great efforts have been attempted on how to increase compression ratio (CR) without loss of critical diagnostic information. In this study, we designed two optimized JPEGXT (JPEGXT_OPT) and JPEG (JPEG_OPT) approaches by amplifying discrete cosine transform coefficients and using the entire anatomical region as ROI (region of interest). We found that ROI percentages have a great impact CR: smaller ROI percentages (10-30 %) could obtain a larger CR. Under the near-lossless compression, JPEGXT_OPT could have CRs up to 4.0 under small ROI percentages (10-30 %), while only similar to 1.2 for large ROI percentages (90-100 %). JPEG_OPT could obtain a much higher CR: up to over 20.0 for both CT and MRI images under small ROI percentages (10 %-30 %), and over 10.0 in CT and 5.0 in MRI under large ROI percentages (90 %). Both of them show a compression efficiency than the DICOMrecommended JPEGXT and JEPG_2000. From the distortion analysis, MSSIM (Multiscale Structural Similarity) and PRD (percent ratio of distortion) indicate our methods have a less image distortion than DICOMrecommended JPEGXT (PDR > 20 %) and JPEG_2000: approximately 3.0 % PRD were seen under JPEG_OPT, while<0.15 % PRD were observed under JPEGXT_OPT. MSSIM > 0.98 was found in JPEG_OPT, which the reconstructed images have almost no changes in luminance, contrast, and structure, and this was confirmed by low PRD (about 3.0 %). Overall, our two methods could provide a high compression ratio of medical images without significant loss of important diagnostic information in reconstructed images.
更多查看译文
关键词
Medical image compression,Region of interest,JPEGXT_OPT,JPEG_OPT,Nearly-lossless and lossy compression
AI 理解论文
溯源树
样例
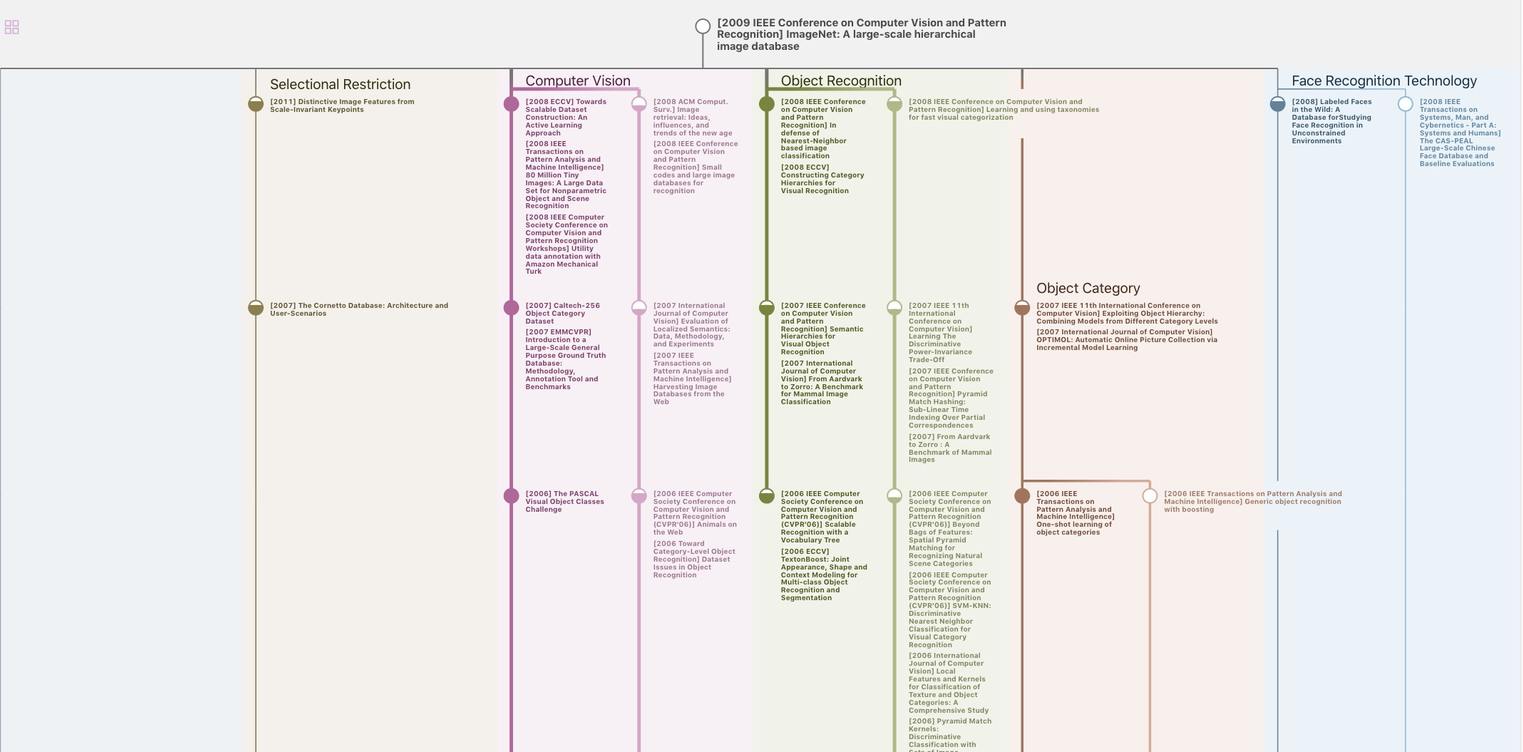
生成溯源树,研究论文发展脉络
Chat Paper
正在生成论文摘要