Ensemble of Fully Convolutional Neural Networks with End-to-End Learning for Small Object Semantic Segmentation.
RiTA(2022)
摘要
Small object segmentation is important due to its vast application potential for biomedical and surveillance. This paper describes several ensemble methods that combine different fully convolutional neural networks (FCNNs) for accurate semantic segmentation of aerial images. One problem with a single model for accurate small object segmentation is that the small object classes are suppressed by large object classes, which can increase overfitting problems and hinder generalization performance. Furthermore, using the single model for segmentation has particular strengths and weaknesses, and the segmentation accuracy changes with the different features used for training. Thus, this paper describes several ensemble methods that combine multiple fully convolutional networks for accurate semantic segmentation of unmanned aerial vehicles (UAVs) images. The proposed method starts with performing object localization for each single FCNN model. After that, each model’s channels of feature map outputs are combined to guide the training of the ensemble segmentation network. We have performed experiments on UAVid benchmark dataset. The results show that the proposed ensemble approach outperforms the single model that directly uses the channels of the single model’s feature map outputs.
更多查看译文
关键词
convolutional neural networks,segmentation,end-to-end
AI 理解论文
溯源树
样例
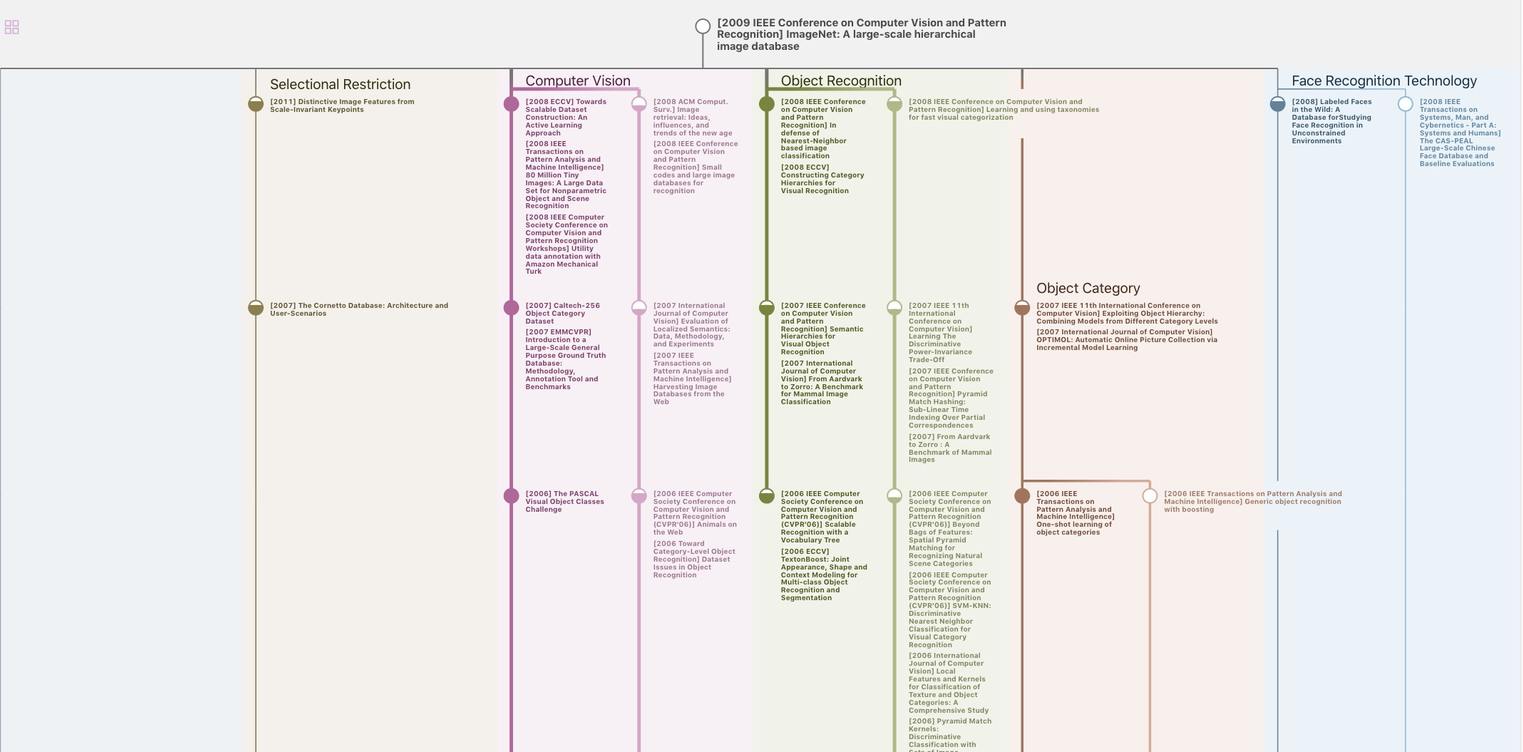
生成溯源树,研究论文发展脉络
Chat Paper
正在生成论文摘要