A deep-learning approach to assess respiratory effort with a chest-worn accelerometer during sleep
Biomed. Signal Process. Control.(2023)
摘要
Objective: The objective is to develop a new deep learning method for the estimation of respiratory effort from a chest-worn accelerometer during sleep. We evaluate performance, compare it against a state-of-the art method, and assess whether it can differentiate between sleep stages. Methods: In 146 participants undergoing overnight polysomnography data were collected from an accelerometer worn on the chest. The study data were partitioned into train, validation, and holdout (test) sets. We used the train and validation sets to generate and train a convolutional neural network and performed model selection respectively, while we used the holdout set (72 participants) to evaluate performance. Results: A convolutional neural network with 9 layers and 207,855 parameters was automatically generated and trained. The neural network significantly outperformed the best performing conventional method, based on Principal Component Analysis; it reduced the Mean Squared Error from 0.26 to 0.11 and it also performed better in the detection of breaths (Sensitivity 98.4 %, PPV 98.2 %). In addition, the neural network exposed significant differences in characteristics of respiratory effort between sleep stages (p < 0.001). Conclusion: The deep learning method predicts respiratory effort with low error and is sensitive and precise in the detection of breaths. In addition, it reproduces differences between sleep stages, which may enable automatic sleep staging, using just a chest-worn accelerometer.
更多查看译文
关键词
Accelerometer,Respiratory effort,Sleep staging,Deep learning,Convolutional neural network,Receptive field,Adam stochastic optimization,Principal component analysis
AI 理解论文
溯源树
样例
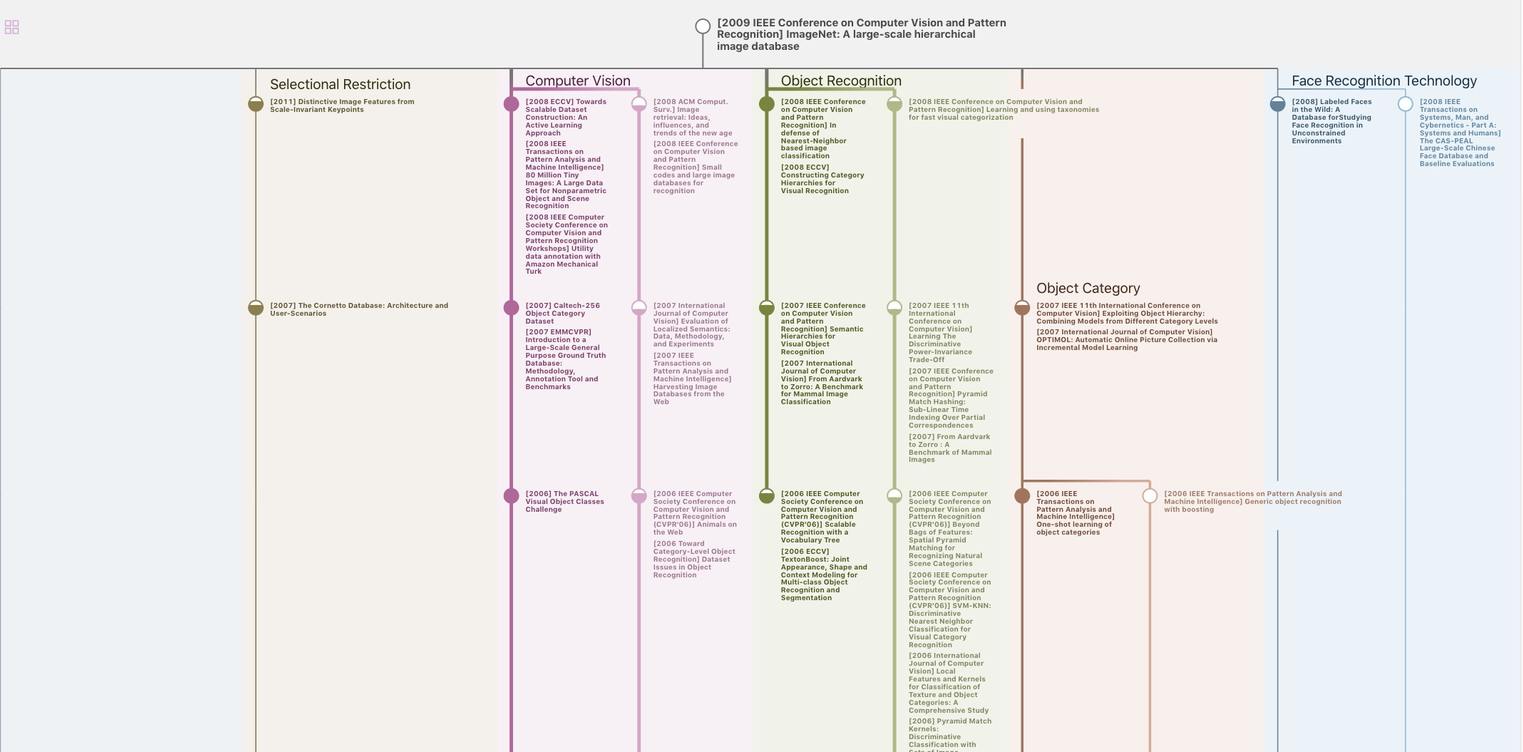
生成溯源树,研究论文发展脉络
Chat Paper
正在生成论文摘要